1
347
1-Learning AI/1-what_is_AI.md
Normal file
|
@ -0,0 +1,347 @@
|
||||||
|
<div align="justify" dir="rtl">
|
||||||
|
|
||||||
|
<p align="center" dir="rtl">بسم الله الرحمن الرحیم</p>
|
||||||
|
|
||||||
|
<p align="center">
|
||||||
|
<img src="img/1.jpg" />
|
||||||
|
<br>
|
||||||
|
<br>
|
||||||
|
</p>
|
||||||
|
اگر کمی هم به تکنولوژی، صنعت، علم و حتی هنر علاقهمند باشید، حتما عبارت هوش مصنوعی را در سالهای اخیر شنیدهاید. در پاسخ به سوال هوش مصنوعی چیست؟ در پاسخ باید گفت که این رشته شاخهای گسترده از علوم کامپیوتر محسوب میشود که بهطورخلاصه و ساده، هدفش تولید سیستمهای هوشمند قادر به انجام فعالیتهای نیازمند به هوش انسانی است. این فعالیت میتواند از نگارش همین محتوایی که در حال مطالعه هستید تا جراحی یا حتی آهنگسازیهای ساده را در بر بگیرد.
|
||||||
|
|
||||||
|
بهطور حتم در سالهای آینده، هوش مصنوعی قطعا نقش فعالتری در زندگی روزمرهی ما بازی خواهد کرد. بنابراین آشنایی با ماهیت، انواع، مزایا و دیگر جزئیات مربوط به آن میتواند جذاب و حتی ضروری باشد. در این مقاله، ضمن بررسی کلی این تکنولوژی و معرفی جوانب آن، انواع مختلفش را مرور کرده و سپس به بیان کاربردها، مفاهیم، مزایا و غیره میپردازیم. با ما همراه شده و با یکی از انقلابیترین تکنولوژیهای حال حاضر دنیا آشنا شوید.
|
||||||
|
|
||||||
|
## [فهرست محتوا](#X){#X}
|
||||||
|
|
||||||
|
<details >
|
||||||
|
<summary>+</summary>
|
||||||
|
<summary><a href="#A1">ماهیت هوش مصنوعی چیست؟</a></summary>
|
||||||
|
<summary><a href="#A2">مکانیسم عملکرد هوش مصنوعی چیست؟</a></summary>
|
||||||
|
<summary><a href="#A3">مثالی از سازوکار هوش مصنوعی</a></summary>
|
||||||
|
<summary><a href="#A4">برنامه نویسی هوش مصنوعی چیست؟</a></summary>
|
||||||
|
<details>
|
||||||
|
<summary><a href="#A5">هوش مصنوعی قوی یا ضعیف</a></summary>
|
||||||
|
<summary><a href="#A6">AI قوی چیست؟</a></summary>
|
||||||
|
<summary><a href="#A7">AI ضعیف چیست؟</a></summary>
|
||||||
|
</details>
|
||||||
|
<details>
|
||||||
|
<summary><a href="#A8">ماشین لرنینگ و دیپ لرنینگ دو المان مهم هوش مصنوعی</a></summary>
|
||||||
|
<summary><a href="#A9">منظور از ماشین لرنینگ در هوش مصنوعی</a></summary>
|
||||||
|
<summary><a href="#A10">منظور از دیپ لرنینگ در هوش مصنوعی</a></summary>
|
||||||
|
</details>
|
||||||
|
<details>
|
||||||
|
<summary><a href="#A11">انواع ماشین های هوش مصنوعی</a></summary>
|
||||||
|
<summary><a href="#A12">ماشین واکنشی</a></summary>
|
||||||
|
<summary><a href="#A13">ماشین حافظه محدود</a></summary>
|
||||||
|
<summary><a href="#A14">ماشین تئوری ذهن</a></summary>
|
||||||
|
<summary><a href="#A15">ماشین خودآگاهی</a></summary>
|
||||||
|
</details>
|
||||||
|
<details>
|
||||||
|
<summary><a href="#A16">نمونه هایی از کاربردها و چند شرکت هوش مصنوعی</a></summary>
|
||||||
|
<summary><a href="#A17">ChatGPT</a></summary>
|
||||||
|
<summary><a href="#A18">گوگل مپ</a></summary>
|
||||||
|
<summary><a href="#A19">دستیارهای هوشمند</a></summary>
|
||||||
|
<summary><a href="#A20">فیلترهای اسنپ چت</a></summary>
|
||||||
|
<summary><a href="#A21">ماشینهای خودران</a></summary>
|
||||||
|
<summary><a href="#A22">گجت های پوشیدنی</a></summary>
|
||||||
|
</details>
|
||||||
|
<details>
|
||||||
|
<summary><a href="#A23">نقش هوش مصنوعی در یادگیری</a></summary>
|
||||||
|
<summary><a href="#A24">آموزش شخصی سازی شده</a></summary>
|
||||||
|
<summary><a href="#A25">آموزش مدرس</a></summary>
|
||||||
|
<summary><a href="#A26">رفع محدودیت زمانی آموزش</a></summary>
|
||||||
|
<summary><a href="#A27">اتوماسیون امور آموزشی</a></summary>
|
||||||
|
<summary><a href="#A28">تولید محتوای هوشمند</a></summary>
|
||||||
|
</details>
|
||||||
|
<summary><a href="#A29">مزایای هوش مصنوعی چیست؟</a></summary>
|
||||||
|
<summary><a href="#A30">پذیرش عمومی؛ مهم ترین چالش هوش مصنوعی</a></summary>
|
||||||
|
<details>
|
||||||
|
<summary><a href="#A31">آینده هوش مصنوعی</a></summary>
|
||||||
|
<summary><a href="#A32">سخن آخر درباره AI</a></summary>
|
||||||
|
</details>
|
||||||
|
<summary><a href="#A33">سوالات متداول درباره هوش مصنوعی</a></summary>
|
||||||
|
</details>
|
||||||
|
|
||||||
|
## [ماهیت هوش مصنوعی چیست؟](#X){#A1}
|
||||||
|
|
||||||
|
بهطورکلی هوش مصنوعی یا Artificial Intelligence و بهاختصار AI عبارت از شبیهسازی فرآیندهای ذهنی و هوش انسانی توسط ماشینها و کامپیوترها بهمنظور تکرار این فرآیند و نتایج حاصل از آن، بدون نیاز به انسان است.
|
||||||
|
|
||||||
|
علیرغم قرارداشتن پایههای هوش مصنوعی در علوم کامپیوتر، امروزه به آن، بهعنوان یک علم میانرشتهای نگاه میشود. حتی ردپای علوم انسانی و پزشکی را نیز میتوان در برخی شاخههای مطالعاتی و کاربردی آن دید. بااینحال این علم، آنطور که شاید بهنظر برسد از زندگی روزمرهی ما دور نیست. در خانه و کامپیوتر هر یک از ما، ردپای آن در محصولات برندهایی مانند گوگل، اپل و آمازون دیده میشود. هربار که Siri را در گوشی اپل و Alexa را در سیستم هوشمند خانگی آمازون خود صدا میزنید، درواقع در حال استفاده از هوش مصنوعی هستید.
|
||||||
|
|
||||||
|
امروزه حتی هنگام خرید نیز ممکن است فروشنده یا تولیدکننده، مدعی استفاده از AI در محصولش شود. منظور آنها در بیشتر مواقع، حضور یکی از جوانب این تکنولوژی مانند ماشین لرنینگ یا یادگیری ماشینی (Machine Learning) در طراحی محصول است.
|
||||||
|
|
||||||
|
## [مکانیسم عملکرد هوش مصنوعی چیست؟](#X){#A2}
|
||||||
|
|
||||||
|
فارغ از تعریف علمی باید بدانیم که سازوکار یک ماشین هوش مصنوعی چیست؟ بهطورساده باید بگوییم اساس عملکرد این ماشینها بر آنالیز دادههای انبوه و سپس مدلسازی آنها استوار است. آنها سپس بر اساس مدل بهدستآمده تصمیم یا نتیجهی لازم را ارائه میدهند. مثلا جستجوی صوتی یا تصویری گوگل با آنالیز زبان، رنگ، المانها و غیره انجام میشود تا مرتبطترین نتایج منطبق با گفتار و مطالب موجود در اینترنت به مخاطب نمایش داده شود. گوگل برای این ویژگی و دیگر امکاناتش از چندین شرکت هوش مصنوعی کمک میگیرد.
|
||||||
|
|
||||||
|
هوش مصنوعی با گذشت دهها سال از زمان مطرح شدنش، هنوز بهطورکامل نتوانسته است خود را از نظارت و دخالت انسانی بینیاز کند. هرچند در برخی موارد مانند بازیهای ویدئویی، دیگر نیازی به نظارت انسانی نیست اما در بیشتر سیستمها حضور انسان برای مدلسازی بهتر و تصحیح اشتباهات ضروری است.
|
||||||
|
|
||||||
|
<p align="center">
|
||||||
|
<img src="img/2.jpg" />
|
||||||
|
<br>
|
||||||
|
مکانیسم هوش مصنوعی چیست؟
|
||||||
|
<br>
|
||||||
|
</p>
|
||||||
|
|
||||||
|
## [مثالی از سازوکار هوش مصنوعی](#X){#A3}
|
||||||
|
|
||||||
|
برای درک بهتر عملکرد هوش مصنوعی یک ربات چت را تصور کنید. امروزه این رباتها را با ورود به وبسایتهای مختلف بهویژه وبسایتهای فروشگاهی بهوفور میبینیم. درحالیکه تصور میشود شاید فردی در حال چت با شماست؛ اما در بیشتر مواقع، اینگونه نیست. این نرمافزارها، شامل تعداد زیادی پیام پیشفرض هستند که در زمان مناسب و در جواب به سوال یا درخواست مشخصی از شما بهعنوان کاربر برایتان ارسال میشود. درواقع، یک ربات چت، تشخیص میدهد که در برابر چه کلمات، حروف و جملاتی، کدام جواب را ارسال کند.
|
||||||
|
|
||||||
|
## [برنامه نویسی هوش مصنوعی چیست؟](#X){#A4}
|
||||||
|
|
||||||
|
اجرای هر تکنولوژی هوش مصنوعی به برنامهنویسی به این زبان نیاز دارد. برنامهنویسی AI شامل سه بخش اصلی زیر است:
|
||||||
|
|
||||||
|
یادگیری؛ در این بخش، قوانین و نحوهی عملکرد یک سیستم AI در قالب الگوریتمهای آن گنجانده شده و کل سیستم بر اساس الگوریتمهایش عمل میکند.
|
||||||
|
استدلال؛ این بخش از برنامهنویسی AI تعیین میکند که کدام الگوریتم برای چه منظوری باید راهاندازی شود.
|
||||||
|
اصلاح؛ این بخش از برنامهی نوشتهشده، خطاهای الگوریتمها را تشخیص داده و بر ارائهی هرچه دقیقتر جوابها در هر بار استفاده متمرکز است.
|
||||||
|
|
||||||
|
آموزش هوش مصنوعی با پایتون و R جاوا اسکریپت و دیگر زبانهای برنامه نویسی انجام میشود.
|
||||||
|
|
||||||
|
## [هوش مصنوعی قوی یا ضعیف](#X){#A5}
|
||||||
|
|
||||||
|
اما منظور از قوی (Strong) و ضعیف (Weak) در هوش مصنوعی چیست؟ در این بخش به این سوال پاسخ خواهیم داد.
|
||||||
|
|
||||||
|
<p align="center">
|
||||||
|
<img src="img/3.jpg" />
|
||||||
|
<br>
|
||||||
|
تفاوت هوش مصنوعی قوی و ضعیف
|
||||||
|
<br>
|
||||||
|
</p>
|
||||||
|
|
||||||
|
## [AI قوی چیست؟](#X){#A6}
|
||||||
|
|
||||||
|
هوش مصنوعی قوی یا Strong AI ماشینی است که میتواند روی مسائل یا مشکلاتی کار کند که برای آنها آموزش ندیده یا برنامهریزی نشده است. این تکنولوژی، هوش مصنوعی را هرچه بیشتر به انسانی شبیه کرده که میتواند در لحظه با هر مسئلهای دستوپنجه نرم کند. به این شاخه از AI، هوش مصنوعی عمومی (Artificial General Intelligence) یا بهاختصار AGI گفته میشود.
|
||||||
|
|
||||||
|
هدف اصلی AGI بهطورخلاصه، تکرار و شبیهسازی توانمندیهای شناختی مغز انسان است. توانمندیهای شناختی مغز مبتنی بر منطق فازی (Fuzzy Logic) بوده و برای انتقال دانش حل مسئله از یک زمینه به زمینهی دیگر استفاده میشوند. حتما میپرسید که معیار قوی بودن یک ماشین هوش مصنوعی چیست؟ در حالت تئوری، فرض دانشمندان بر این است که اگر ماشینی بتواند دو آزمون تورینگ یا بازی تقلید (Turing Test) و اتاق چینی (Chinese Room) را پشت سر بگذارد، میتوان آن را AI قوی قلمداد کرد. این دو تست برای ارزیابی میزان و قدرت هوش مصنوعی کامپیوترها و الگوریتمهای آنها طراحی شدهاند.
|
||||||
|
|
||||||
|
AI قوی را اکنون باید تنها در شخصیتهای فیلمهای علمیتخیلی مانند شخصیت Data در Star Track ببینیم. دانشمندان در آزمایشگاه به نتایج محدودی دربارهی این تکنولوژی رسیدهاند. آنها اما مانند بسیاری از تکنولوژیهایی که امروز از آنها استفاده میکنیم، امید دارند که AI قوی نیز قطعا روزی به واقعیت بدل شود. بسیاری نیز نگران نتایج غیرقابلکنترل اعمال ماشینهایی هستند که با موفقیت در راهاندازی کامل این تکنولوژی، ممکن است اتفاق بیفتد.
|
||||||
|
|
||||||
|
## [AI ضعیف چیست؟](#X){#A7}
|
||||||
|
|
||||||
|
اما نوع ضعیف هوش مصنوعی چیست؟ تابهامروز هر استفادهای که از هوش مصنوعی کردهایم، مربوط به این حوزه بوده است. به این شاخه، هوش مصنوعی باریک (Narrow AI) و Specialized AI نیز گفته میشود . منظور از AI ضعیف، کاربرد این تکنولوژی در شاخهای خاص از تکنولوژی، صنعت، پزشکی یا هر زمینهی دیگری است.
|
||||||
|
|
||||||
|
یک ماشین مجهز به هوش مصنوعی ضعیف، تنها قادر است که ذهن انسان را در رابطه با مهارت، چالش یا موضوعی خاص شبیهسازی کرده و بر اساس الگوریتمهایش مدلسازی کند. نمونههایی از هوش مصنوعی ضعیف عبارتند از:
|
||||||
|
|
||||||
|
- سیری و الکسا و کلیه Assistant های هوشمند
|
||||||
|
- ماشینهای خودران
|
||||||
|
- جستجوی گوگل
|
||||||
|
- رباتهای مکالمهای
|
||||||
|
- فیلترهای اسپم ایمیل
|
||||||
|
- پیشنهاددهندههای محتوا در شبکههای اجتماعی مانند یوتیوب و گوگل
|
||||||
|
|
||||||
|
## [ماشین لرنینگ و دیپ لرنینگ دو المان مهم هوش مصنوعی](#X){#A8}
|
||||||
|
|
||||||
|
دو المان مهم مفهومی و تکنولوژیکی هوش مصنوعی ماشین لرنینگ و دیپ لرنینگ (Deep Learning) هستند. درحالیکه بسیاری آنها را بهجای یکدیگر بهکار میبرند؛ اما ماهیت و کارکرد آنها متفاوت است. در این بخش، این دو المان را معرفی میکنیم.
|
||||||
|
|
||||||
|
<p align="center">
|
||||||
|
<img src="img/4.jpg" />
|
||||||
|
<br>
|
||||||
|
ماشین لرنینگ و دیپ لرنینگ
|
||||||
|
<br>
|
||||||
|
</p>
|
||||||
|
|
||||||
|
## [منظور از ماشین لرنینگ در هوش مصنوعی](#X){#A9}
|
||||||
|
|
||||||
|
منظور از ماشین لرنینگ در هوش مصنوعی چیست؟ ماشین لرنینگ فرایندی است که طی آن، دادههای یک الگوریتم توسط کامپیوتر و تکنیکهای آماری تغذیه میشوند. هدف از این کار، کمک به یادگیری و بهبود تدریجی عملکرد الگوریتم است. این الگوریتم لزوما برای انجام یک کار خاص برنامهریزی نشده است؛ اما بهواسطهی این سازوکار میتواند به تدریج، روند انجام آن را فرا بگیرد.
|
||||||
|
|
||||||
|
به یک الگوریتم Machine Learning بهاختصار ML گفته میشود. این الگوریتم از دادههای قبلی و ساختاریافته بهمنظور پیشبینی مقادیر خروجی خود استفاده میکند. بر این اساس، ماشین لرنینگ خود به دو نوع زیر تقسیم میشود:
|
||||||
|
|
||||||
|
یادگیری نظارتشده یا supervised learning که در آن، نتایج بر اساس دادههای ورودی برچسبگذاریشده یا ساختاریافته از قبل مشخص هستند.
|
||||||
|
یادگیری غیرنظارتشده یا unsupervised learning که در آن از دادههای بدون برچسب یا غیرساختاری استفاده میشود. نتایج این الگوریتم، غیرقابلپیشبینی هستند.
|
||||||
|
|
||||||
|
## [منظور از دیپ لرنینگ در هوش مصنوعی](#X){#A10}
|
||||||
|
|
||||||
|
اما منظور از دیپ لرنینگ (Deep Learning) در هوش مصنوعی چیست؟ این الگوریتم، نوعی الگوریتم ماشین لرنینگ است که دادههای ورودی خود را با الهام از مدلهای شبکهی عصبی موجودات زنده اجرا میکند. این مدلها از علم بیولوژی کپی میشوند. در یک شبکهی عصبی، لایههای متعدد (حداقل سه لایه) وجود دارند. هریک از این لایهها میتوانند ورودی یا خروجی باشند. وظیفهی نهایی آنها نیز این است که دادهها را در سطوح متفاوتی پردازش کنند. این مکانیسم به الگوریتم، امکان یادگیری عمیقتر الگوی موردنظر را میدهند.
|
||||||
|
|
||||||
|
یک شرکت هوش مصنوعی عمیق تلاش میکند با کاربرد دیپ لرنینگ در الگوریتمهای خود، مداخلات انسانی و نیاز به نظارت او را کاهش دهد. این الگوریتم، ویژگیهای خودکار بیشتری نسبت یادگیری ماشینی داشته و امکان پردازش دادههای بزرگتر را فراهم میکند. بهاینترتیب میتوان این دیپ لرنینگ را نوعی یادگیری ماشینی مقیاسپذیر دانست.
|
||||||
|
|
||||||
|
یادگیری عمیق همچنین قدرت بالایی در پردازش دادههای غیرساختاری خام مانند تصاویر و متون دارد. چنین سیستمی میتواند با استفاده از ویژگیهای سلسلهمراتبی که برایش تعریف شده، این نوع دادهها را بهراحتی و با دقت و سرعت بیشتری طبقهبندی کند.
|
||||||
|
|
||||||
|
## [انواع ماشین های هوش مصنوعی](#X){#A11}
|
||||||
|
|
||||||
|
از سال ۲۰۱۶ و بر اساس پیشنهاد آرند هینتزه (Arend Hintze) استاد دانشگاه ایالتی میشیگان در رشتههای زیستشناسی، علوم کامپیوتر و مهندسی، ماشینهای هوش مصنوعی به چهار دسته تقسیمبندی شدند. این دستهبندی جزئیات بیشتری در مورد نوع و پیچیدگی وظایف یک سیستم AI ارائه میدهد. در ادامه، انواع هوش مصنوعی بر این اساس را بررسی میکنیم.
|
||||||
|
|
||||||
|
## [ماشین واکنشی](#X){#A12}
|
||||||
|
|
||||||
|
ماشین واکنشی یا Reactive Machine در هوش مصنوعی چیست؟ این سیستم از ابتداییترین مفاهیم هوش مصنوعی بهره میبرد. همانطورکه از عنوان این ماشین پیداست، تنها قادر است از الگوریتمهای خود برای درک و واکنش متقابل استفاده کند. Reactive Machine، حافظهای ندارد و نمیتواند اطلاعات را ذخیره کند. بنابراین استفاده از دادههای گذشته برای مدلسازیهای بعدی نیز در آن منتفی است.
|
||||||
|
|
||||||
|
ماشینهای واکنشی یا واکنشگرا برای انجام وظایف خاصی طراحی میشوند. محدودیت عملکرد و ادراک آنها، سبب قابلاعتمادترشدن نتایج حاصل از الگوریتمهایشان میشود.
|
||||||
|
|
||||||
|
<p align="center">
|
||||||
|
<img src="img/5.jpg" />
|
||||||
|
<br>
|
||||||
|
انواع ماشین در هوش مصنوعی
|
||||||
|
<br>
|
||||||
|
</p>
|
||||||
|
|
||||||
|
## [ماشین حافظه محدود](#X){#A13}
|
||||||
|
|
||||||
|
یک ماشین هوش مصنوعی حافظه محدود (Limited Memory) میتواند دادها و پیشبینیهای قبلی ذخیره کند. اطلاعات هنگام مدلسازیها و ارائه نتایج در دفعات بعدی اجرای الگوریتمها به کمک سیستم آمده و نتایج آن را دقیقتر میکنند. هدف از طراحی چنین سیستمی بهدستآوردن پیشبینیهای محدود، دربارهی نتایج با توجه به دادههای گذشته است.
|
||||||
|
|
||||||
|
یک سیستم هوش مصنوعی حافظه محدود با پرورش یک مدل و آموزش آن، برای تجزیه و تحلیل و نحوه استفاده از دادههای جدید ساخته میشود. بهاینترتیب مدل موردنظر قابلیتهای عملکرد خودکار بیشتری نسبت به ماشینهای واکنشی خواهد داشت.
|
||||||
|
|
||||||
|
اگر میپرسید که نحوهی ساخت یک ماشین حافظه محدود هوش مصنوعی چیست؟ بهطورساده باید به مراحل زیر اشاره کنیم:
|
||||||
|
|
||||||
|
- تولید دادههای آموزشی برای ارائه به ماشین
|
||||||
|
- برنامهنویسی مدل ماشین لرنینگ
|
||||||
|
- تست و اطمینان از توانایی پیشبینی مدل
|
||||||
|
- اطمینان از توانایی مدل در دریافت بازخوردهای انسانی و محیطی
|
||||||
|
- ذخیره بازخوردهای انسانی و محیطی بهعنوان داده
|
||||||
|
- تکرار چرخهای پنج مرحلهی قبلی
|
||||||
|
|
||||||
|
## [ماشین تئوری ذهن](#X){#A14}
|
||||||
|
|
||||||
|
ماشین تئوری ذهن (Theory of the Mind) هنوز در حد تئوری بوده و بشر هنوز به تواناییهای لازم برای شکوفایی پتانسیلهای آن دست نیافته است. این تئوری بر یک فرضیهی اساسی روانشناختی استوار است که میگوید رفتار فرد میتواند تحت تاثیر افکار و احساسات دیگران قرار بگیرد.
|
||||||
|
|
||||||
|
بر این اساس، محققان این حوزه در تلاش برای ساختن ماشینی هستند که بتواند احساس یا منظور موجودات زنده و دیگر ماشینها را را درک کند. این ماشین از طریق تامل خودش (Self-Reflection) در مورد این اطلاعات، تصمیمگیری و عمل میکند. بنابراین با اختراع ماشین تئوری ذهن، ارتباط حسی در زمان واقعی بین ذهن انسان و هوش مصنوعی برقرار خواهد شد.
|
||||||
|
|
||||||
|
<p align="center">
|
||||||
|
<img src="img/6.jpg" />
|
||||||
|
<br>
|
||||||
|
ماشین تئوری ذهن در AI
|
||||||
|
<br>
|
||||||
|
</p>
|
||||||
|
|
||||||
|
## [ماشین خودآگاهی](#X){#A15}
|
||||||
|
|
||||||
|
کلمه خودآگاهی را در روانشناسی و علوم انسانی زیاد میشنویم؛ اما منظور از آن در هوش مصنوعی چیست؟ بهطورساده باید گفت که پیدایش این ماشین، به پیدایش ماشین هوش مصنوعی تئوری ذهن وابسته است. ماشین هوش مصنوعی، خودآگاهی (Self-Awareness) درحالحاضر حد نهایت پیشرفت این تکنولوژی تلقی میشود. سطح آگاهی چنین ماشینی در حد انسان بوده و از وجود خود در جهان و حضور دیگران و وضعیت احساسی و ذهنیشان آگاه است.
|
||||||
|
|
||||||
|
در حالت ایدهآل یک ماشین خودآگاه میتواند بفهمد که نیازهای دیگران نهفقط بهواسطهی دادههای ورودی؛ بلکه بر اساس نوع رفتار، حالت چهره، حالت صدا و بهطورکلی نحوهی برقراری ارتباط آنها چیست. لازمهی پیشرفت در این زمینه، پیش از هرچیز این است که مکانیسم هوشیاری و خودآگاهی در انسان درک شود. عرصهای که هنوز، ناشناختههای زیادی برای دانشمندان دارد. پسازآن لازم است که مدلهایی برای تکرار و پیادهسازی فرآیند خودآگاهی در ماشین هوش مصنوعی طراحی شوند.
|
||||||
|
|
||||||
|
## [نمونه هایی از کاربردها و چند شرکت هوش مصنوعی](#X){#A16}
|
||||||
|
|
||||||
|
همانطورکه تاکنون نیز متوجه شدهاید، هوش مصنوعی در میانهی راه خود قرار دارد. برخی اهداف و جنبههای آن محقق شده و برخی دیگر هنوز در مرحلهی تئوری هستند. هماکنون تعداد زیادی شرکت هوش مصنوعی با محصولات متفاوت در حال فعالیت هستند. برای درک بهتر موقعیت فعلی این تکنولوژی و کاربردهایش در ادامه مثالهایی از آن را بررسی میکنیم.
|
||||||
|
|
||||||
|
## [ChatGPT](#X){#A17}
|
||||||
|
|
||||||
|
ربات چت جیپیتی یک ربات، توسعهیافته با هوش مصنوعی است که قادر است محتوای نوشتاری در قالبهای مختلف تولید کند. این قالبها از مقاله گرفته تا کدهای برنامهنویسی و پاسخ به سوالات ساده را در بر میگیرند. شرکت هوش مصنوعی OpenAI در ماه نوامبر ۲۰۲۲ این محصول را با استفاده از یک مدل زبانی تولید کرد که قادر به تقلید بسیار عالی متون نوشتهشده توسط انسان است. برای مطالعهی بیشتر دراینباره مقالهی ChatGPT چیست و چگونه کار میکند؟ را به شما توصیه میکنیم.
|
||||||
|
ی
|
||||||
|
|
||||||
|
<p align="center">
|
||||||
|
<img src="img/7.jpg" />
|
||||||
|
<br>چت جی پی ت<br>
|
||||||
|
</p>
|
||||||
|
|
||||||
|
## [گوگل مپ](#X){#A18}
|
||||||
|
|
||||||
|
اگر میپرسید که رابطهی گوگل مپ و هوش مصنوعی چیست؟ باید بگوییم همهچیز! گوگل مپ یکی از شناختهشدهترین محصولات نرمافزاری توسعهیافته با هوش مصنوعی است. نقشههای گوگل بر اساس دادههایی تدوین یافتهاند که از موقعیت مکانی گوشیهای هوشمند و نیز دادههای گزارششده توسط خود کاربران تهیه میشوند. وضعیت ترافیکی جادهها، تصادف و بسته شدن موقت جاده، نمونههایی از این دادهها هستند. گوگل مپ با استفاده از هوش مصنوعی است که میتواند سریعترین و کوتاهترین مسیر، زمان تقریبی رسیدن شما به محل و دیگر جزئیات را به شما ارائه کند.
|
||||||
|
|
||||||
|
## [دستیارهای هوشمند](#X){#A19}
|
||||||
|
|
||||||
|
به Smart Assistant ها در بخشهای قبلی مقاله نیز اشاراتی کردیم. سیری، الکسا و کورتانا (متعلق به شرکت مایکروسافت) معروفترینهای این صنعت هستند. اما رابطهی آنها با هوش مصنوعی چیست؟ این دستگاهها از الگوریتمهای هوش مصنوعی پردازش زبان طبیعی (Natural Language Processing) یا بهاختصار NPL برای دریافت دستورالعملهای صوتی از کاربران استفاده میکنند. خدمات آنها مواردی مانند یادآوری، جستجوی اطلاعات آنلاین و کنترل نور محیط را شامل میشود. هوش مصنوعی دستیارهای هوشمند، قادر به بهبود عملکرد خود و ارائهی پیشنهادهای بهتر با ذخیرهی اطلاعات قبلی و مدلسازی ترجیحات کاربران است.
|
||||||
|
|
||||||
|
<p align="center">
|
||||||
|
<img src="img/8.jpg" />
|
||||||
|
<br>مثال هوش مصنوعی<br>
|
||||||
|
</p>
|
||||||
|
|
||||||
|
## [فیلترهای اسنپ چت](#X){#A20}
|
||||||
|
|
||||||
|
همانطورکه میدانید اسنپ چت، یک اپلیکیشن پیامرسان و شبکه اجتماعی است که از طریق آن میتوان پیامهای تصویری، ویدئوی و متنی ارسال کرد. ویژگی اصلی این اپلیکیشن، ناپدیدشدن پیامها پس از مشاهده توسط دریافتکننده است. اسنپ چت از الگوریتمهای ماشین لرنینگ برای تمایز سوژهی تصویری (پیام تصویری موردنظر) و پسزمینه استفاده میکند. به این ویژگی، فیلتر اسنپ چت گفته میشود.
|
||||||
|
|
||||||
|
## [ماشینهای خودران](#X){#A21}
|
||||||
|
|
||||||
|
در یک ماشین خودران نقش هوش مصنوعی چیست؟ در این صنعت، هوش مصنوعی همهکاره است. چنین خودرویی از الگوریتمهای یادگیری عمیق برای شناسایی اشیاء و خودروهای اطراف، تعیین فاصلهی ایمن، سرعت لازم، شناسایی مسیر، شناسایی علائم رانندگی و غیره استفاده میکند. بهعنوان مثال، ویژگی اتوپایلوت (Autopilot) در خودروهای شرکت تسلا و سوپرکروز (Super Cruise) در برخی محصولات جنرال موتورز از هوش مصنوعی استفاده میکنند.
|
||||||
|
|
||||||
|
## [گجت های پوشیدنی](#X){#A22}
|
||||||
|
|
||||||
|
حسگرها و دستگاههای دیجیتال پوشیدنی امروزه کاربرد فراوانی در زندگی روزمره بهخصوص با کاربردهای پزشکی پیدا کردهاند. آنها میتوانند فشار خون، ضربان قلب، قند خون و دیگر ویژگیهای مربوط به سلامتیتان را به شما گزارش کنند. این دستگاهها گزارشهای خود را با استفاده از دادههای پزشکی قبلیتان بهدست میآورند که با تکنولوژی جمعآوری شدهاند.
|
||||||
|
|
||||||
|
<p align="center">
|
||||||
|
<img src="img/9.jpg" />
|
||||||
|
<br>مزایای هوش مصنوعی<br>
|
||||||
|
</p>
|
||||||
|
|
||||||
|
##[نقش هوش مصنوعی در یادگیری](#X){#A23}
|
||||||
|
|
||||||
|
یکی از مهمترین کاربردهای AI در صنعت آموزش است. این تکنولوژی در سالهای اخیر آموزش شخصیسازیشده، سریع، جذاب، اینتراکتیو و چند بعدی را بیشازپیش محقق کرده و بهخصوص در دوران کرونا پتانسیلهای خود را بیشتر نشان داد. درحال حاضر تعداد زیاد شرکت هوش مصنوعی با تمرکز بر کاربردهای آن در آموزش در دنیا فعال بوده و حتی بودجههای دولتی دریافت میکنند. هدف آنها بهطورخلاصه، بهبود تجربهی آموزش برای سازمان، مدرس و فراگیر از جهات مالی، علمی و غیره است. در ادامه، نقش هوش مصنوعی در یادگیری را با بررسی پنج فاکتور مهم، بررسی میکنیم.
|
||||||
|
|
||||||
|
## [آموزش شخصی سازی شده](#X){#A24}
|
||||||
|
|
||||||
|
یکی از مهمترین معضلات آموزش، شباهت روش و محیط یادگیری برای همهی فراگیران است. این در حالی است که مغز هر فرد، الگوها و عادات یادگیری متفاوتی دارد. اگر میپرسید که در این زمینه فایدهی هوش مصنوعی چیست؟ باید گفت که ماشینهای AI، علایق، توانمندیها، اولویتها و دیگر اطلاعات در مورد فراگیران را دریافت کرده و فرآیند یادگیری را برای آنها شخصیسازی میکنند. یک نمونهی پیشرو از کاربرد AI در آموزش، پروژهی ملی آموزش در کشور استونی است.
|
||||||
|
|
||||||
|
## [آموزش مدرس](#X){#A25}
|
||||||
|
|
||||||
|
دیگر کاربرد هوش مصنوعی در یادگیری، آموزش و توسعهی علمی، مدیریتی و فردی مدرس است. چالشی که آموزش، همواره با آن مواجه بوده و یکی از دلایل افت کیفیتش محسوب میشود. تکنولوژی AI میتواند به توسعهی شبکههای کامپیوتری و اینترنتی منجر شود که مدرس بتواند از شیوههای جدید یادگیری آگاه شده و همواره بر لبهی علمی حوزهی آموزشی خود حرکت کند.
|
||||||
|
|
||||||
|
## [رفع محدودیت زمانی آموزش](#X){#A26}
|
||||||
|
|
||||||
|
به کمک AI یادگیری دیگر به ساعات کلاس محدود نخواهد بود. بهعنوان مثال دانشآموزان میتوانند با استفاده از رباتهای چت، سوالات علمی و سوالات مربوط به امور اداری آموزش را از سیستم هوش مصنوعی بپرسند. بهاینترتیب، سرعت و کیفیت یادگیری افزایش یافته و در هزینه و زمان مدرس و موسسهی آموزشی نیز صرفهجویی میشود.
|
||||||
|
|
||||||
|
<p align="center">
|
||||||
|
<img src="img/10.jpg" />
|
||||||
|
<br>نقش هوش مصنوعی در یادگیری<br>
|
||||||
|
</p>
|
||||||
|
|
||||||
|
## [اتوماسیون امور آموزشی](#X){#A27}
|
||||||
|
|
||||||
|
نظرسنجیها نشان میدهند که معلمهای مدارس در کشورهای پیشرفته، حدود ۳۰ درصد از زمان خود را صرف امور اداری آموزش میکنند. برنامهریزی آموزش، نمرهدهی، حضور و غیاب و غیره نمونههایی از این امور هستند. مشخص است که دراینباره فایده هوش مصنوعی چیست؟ این موارد میتوانند به کمک هوش مصنوعی تا حد زیادی بهصورت خودکار انجام شوند. این صنعت حتی میتواند به کمک تصحیح آزمونها و بررسی تکالیف دانشآموزان بیاید.
|
||||||
|
|
||||||
|
## [تولید محتوای هوشمند](#X){#A28}
|
||||||
|
|
||||||
|
بهویژه در آموزش مجازی، نقش محتوا بسیار پررنگ است. نقش هوش مصنوعی در یادگیری آنلاین و حتی حضوری از کمک به تولید محتوای آموزشی جذاب و سریع شروع شده و تا ایجاد محیطهای مطالعاتی پیچیده، ادامه مییابد. نرمافزارهای آموزشی متعددی از AI برای تولید محتوای خود استفاده میکنند. نرمافزار آموزش مجازی زبان دولینگو، یک نمونه از آنهاست. تولید و طراحی مواردی مانند دروس دیجیتال، راهنمای مطالعه، تمرین و آزمون نیز میتواند بهراحتی و با کمک الگوریتمهای AI انجام شود.
|
||||||
|
|
||||||
|
## [مزایای هوش مصنوعی چیست؟](#X){#A29}
|
||||||
|
|
||||||
|
هوش مصنوعی در بسیاری از زمینهها تحقق رویاهای دیرینه انسان را بیشازپیش به واقعیت نزدیک کرده است. بودجههای هنگفت صرفشده برای آن در چند سال اخیر، گواه این موضوع هستند. از مهمترین مزایای این تکنولوژی میتوان به موارد زیر اشاره کرد:
|
||||||
|
|
||||||
|
- توانایی پردازش حجم بسیار زیاد اطلاعات در مدتزمان بسیار اندک
|
||||||
|
- پردازش و نتیجهگیری از اطلاعات با دقتی بسیار بالاتر از هر ماشین و تکنولوژی دیگر
|
||||||
|
- توانایی پیشبینی بسیار بالا
|
||||||
|
- تسهیل زندگی روزمره و توسعهی جهانی رفاه انسانی
|
||||||
|
- کمک به شفافیت اطلاعات
|
||||||
|
|
||||||
|
بهعنوان مثال درحالحاضر در صنعت بانکداری، بیش از نیمی از شرکتهای خدمات مالی از هوش مصنوعی برای مدیریت داراییها، درآمدزایی و کاهش ریسک سرمایهگذاری استفاده میکنند. هوش مصنوعی، هزینههای آنها را در این زمینهها بهشدت کاهش میدهد. در صنعتی پزشکی نیز AI در کشف و توسعه واکسنها، تشخیص دقیقتر بیماریها و توسعه سیاستهای بهداشتی کاربردهای فراوانی پیدا کرده است. رسانهها و پلتفرمهای شبکهی اجتماعی نیز از هوش مصنوعی با هدف جلوگیری از سرقت ادبی و بهبود ویژگیهای گرافیکیشان بهره میبرند.
|
||||||
|
|
||||||
|
## [پذیرش عمومی؛ مهم ترین چالش هوش مصنوعی](#X){#A30}
|
||||||
|
|
||||||
|
اگر می پرسید که مهمترین چالش هوش مصنوعی چیست؟ باید بگوییم ترس! درحالحاضر علاوه بر چالشهای فنی، مالی و علمی AI، این تکنولوژی با چالش پذیرش عمومی روبهروست. نتایج نظرسنجیهای مختلف بهویژه در آمریکا نشان میدهد که ۳۰ الی ۴۰ درصد مردم، نسبت به این تکنولوژی بدبین هستند. بسیاری از آنها حتی اعتقاد دارند که AI میتواند بشریت را نابود کند.
|
||||||
|
|
||||||
|
حدود ۴۵ درصد افراد شرکتکننده در نظرسنجیها نیز نگرانیهایی در مورد آیندهی هوش مصنوعی دارند. نگرانی عمومی از جایگزینی رباتها با انسان در انجام کارها و افزایش نرخ بیکاری و نتایج ناگوار ناشی از خطا در توسعهی این تکنولوژی بهخصوص در پزشکی و اتوموبیلهای خودروان، برخی چالشهای پیش روی هر شرکت هوش مصنوعی هستند.
|
||||||
|
|
||||||
|
## [آینده هوش مصنوعی](#X){#A31}
|
||||||
|
|
||||||
|
با توجه به توضیحات ارائه شده سوال مهم و عمومی این است که در آیندهی نزدیک جوامع انسانی نقش هوش مصنوعی چیست؟ واقعیت این است که زیرساختها و هزینههای محاسباتی، اجرایی و تحقیقاتی لازم برای تحقق کامل اهداف هوش مصنوعی، هنوز کاملا فراهم نشدهاند. بااینحال، روند پیشرفت این صنعت در دههی ۲۰۱۰ و ابتدای دههی ۲۰۲۰، نشان میدهد که سرعت پیشرفت AI در سالهای اخیر، حتی از قانون مور (Moore) نیز بهتر بوده است. قانونی که در ارزیابی و پیشبینی پیشرفت صنایع تکنولوژیک، کاربرد داشته و میگوید که تعداد ترانزیستورهای روی یک تراشه، بهطور متوسط هر دو سال یک بار، دوبرابر میشود. به همین دلیل، پیشبینیها در مورد آینده هوش مصنوعی ، حاکی از شکوفایی کامل آن تا پیش از سال ۲۰۳۰ است. اتفاقی که برای اینترنت در ۱۰ سال گذشته رخ داد.
|
||||||
|
|
||||||
|
<p align="center">
|
||||||
|
<img src="img/11.jpg" />
|
||||||
|
<br>آینده هوش مصنوعی<br>
|
||||||
|
</p>
|
||||||
|
|
||||||
|
## [سخن آخر درباره AI](#X){#A32}
|
||||||
|
|
||||||
|
هوش مصنوعی چیست؟ این سوالی بود که تلاش کردیم در این مقاله، بهطور کامل به آن پاسخ دهیم. Artificial Intelligence یکی از تکنولوژیهای پیشرو در دنیای امروز ماست. این تکنولوژی تلاش میکند با طراحی و توسعهی الگوریتمهای یادگیری ماشینی، رفتار انسان را در ابتدا درک کرده و به آن پاسخ دهد. در حالت ایدهآل نیز ماشینهای توسعهیافتهی AI میتوانند مانند انسان رفتار کرده و حتی حالات و احساسات او را درک و تقلید کنند.
|
||||||
|
|
||||||
|
هوش مصنوعی هماکنون نیز در زندگی بسیاری از ما حضور داشته و نشانههای حضور آن در گوشیهای تلفن همراه، آموزش، پزشکی و دیگر صنایع، بهوضوح دیده میشود. با وجود چالشها و نگرانیهای موجود، بیشتر کارشناسان، آینده هوش مصنوعی را درخشان و قابلمقایسه با جایگاه اینترنت در دنیای امروز میدانند.
|
||||||
|
|
||||||
|
## [سوالات متداول درباره هوش مصنوعی](#X){#A33}
|
||||||
|
|
||||||
|
<details>
|
||||||
|
<summary>هوش مصنوعی چیست و چگونه کار میکند؟</summary>
|
||||||
|
هوش مصنوعی یک حوزه از علوم کامپیوتر است که به طراحی و توسعه سیستمها و برنامههایی میپردازد که به نحوی مشابه با نحوه عملکرد مغز انسان، بتوانند مسائل را حل و تصمیمگیری کنند. هوش مصنوعی بر اساس الگوریتمها و مدلهایی مانند شبکههای عصبی و یادگیری ماشینی کار میکند.
|
||||||
|
</details>
|
||||||
|
<details>
|
||||||
|
<summary>آیا هوش مصنوعی تنها در صنعت و فناوری مورد استفاده قرار میگیرد؟</summary>
|
||||||
|
|
||||||
|
خیر، هوش مصنوعی به عنوان یک حوزه چند رشتهای، در زمینههای مختلفی مانند پزشکی، حقوق، آموزش و پرورش، بازاریابی و حوزههای دیگر نیز استفاده میشود.
|
||||||
|
|
||||||
|
</details>
|
||||||
|
<details>
|
||||||
|
<summary>چه مزایا و معایبی برای استفاده از هوش مصنوعی وجود دارد؟</summary>
|
||||||
|
|
||||||
|
استفاده از هوش مصنوعی مزایای زیادی دارد از جمله: افزایش سرعت و کارایی در انجام وظایف، قابلیت تحلیل دادههای بزرگ و پیچیده، کاهش خطاهای انسانی و بهبود تصمیمگیری. اما در عین حال، معایبی مانند وابستگی بیش از حد به تکنولوژی، مسائل امنیتی و حریم خصوصی و همچنین نگرانیهای اجتماعی و اخلاقی نیز وجود دارد.
|
||||||
|
|
||||||
|
</details>
|
||||||
|
<details>
|
||||||
|
<summary>آیا هوش مصنوعی میتواند جایگزین انسانها در برخی فعالیتها شود؟</summary>
|
||||||
|
هوش مصنوعی میتواند در برخی فعالیتها و وظایف خاص جایگزین انسانها شود، اما برخی از فعالیتها مانند فعالیتهای خلاقیت و هنری که نیازمند دانش عمیق انسانی هستند، هنوز به طور کامل قابل جایگزینی توسط هوش مصنوعی نیستند.
|
||||||
|
</details>
|
||||||
|
<details>
|
||||||
|
<summary>چه تفاوتی بین هوش مصنوعی و یادگیری ماشینی وجود دارد؟</summary>
|
||||||
|
هوش مصنوعی به طور کلی به همه روشها و فنونی اطلاق میشود که برای تقویت هوش کامپیوترها استفاده میشود. در عین حال، یادگیری ماشینی یک زیرمجموعه از هوش مصنوعی است که تمرکز خود را بر روی توانایی کامپیوترها برای یادگیری از دادهها قرار میدهد.
|
||||||
|
</details>
|
||||||
|
|
||||||
|
هوش مصنوعی به طور کلی به همه روشها و فنونی اطلاق میشود که برای تقویت هوش کامپیوترها استفاده میشود. در عین حال، یادگیری ماشینی یک زیرمجموعه از هوش مصنوعی است که تمرکز خود را بر روی توانایی کامپیوترها برای یادگیری از دادهها قرار میدهد.
|
||||||
|
|
||||||
|
<p align="center" dir="rtl">صلوات</p>
|
||||||
|
</div>
|
BIN
1-Learning AI/img/1.jpg
Normal file
After Width: | Height: | Size: 71 KiB |
BIN
1-Learning AI/img/10.jpg
Normal file
After Width: | Height: | Size: 90 KiB |
BIN
1-Learning AI/img/11.jpg
Normal file
After Width: | Height: | Size: 67 KiB |
BIN
1-Learning AI/img/2.jpg
Normal file
After Width: | Height: | Size: 66 KiB |
BIN
1-Learning AI/img/3.jpg
Normal file
After Width: | Height: | Size: 35 KiB |
BIN
1-Learning AI/img/4.jpg
Normal file
After Width: | Height: | Size: 70 KiB |
BIN
1-Learning AI/img/5.jpg
Normal file
After Width: | Height: | Size: 60 KiB |
BIN
1-Learning AI/img/6.jpg
Normal file
After Width: | Height: | Size: 52 KiB |
BIN
1-Learning AI/img/7.jpg
Normal file
After Width: | Height: | Size: 69 KiB |
BIN
1-Learning AI/img/8.jpg
Normal file
After Width: | Height: | Size: 29 KiB |
BIN
1-Learning AI/img/9.jpg
Normal file
After Width: | Height: | Size: 50 KiB |
154
2-Learning NLP/1-Introduction_to_NLP.md
Normal file
|
@ -0,0 +1,154 @@
|
||||||
|
<div align="justify" dir="rtl">
|
||||||
|
|
||||||
|
<p align="center" dir="rtl">بسم الله الرحمن الرحیم</p>
|
||||||
|
|
||||||
|
<p align="center">
|
||||||
|
<img src="img/1.jpg" />
|
||||||
|
<br>
|
||||||
|
<br>
|
||||||
|
</p>
|
||||||
|
|
||||||
|
# پردازش زبان طبیعی چیست؟
|
||||||
|
|
||||||
|
## آشنایی کامل با این حوزه هوش مصنوعی
|
||||||
|
|
||||||
|
به لطف پیشرفت در حوزههایی مانند هوش مصنوعی، یادگیری ماشین و علم داده، انقلاب بزرگی در حال وقوع است. شاخه پردازش زبان طبیعی یکی از حوزههای هوش مصنوعی در حال پیشرفت است: امروزه دیگر از مرحله تفسیر یک متن یا گفتار بر اساس کلمات کلیدی آن گذشتیم و به دنبال درک معنای پشت آن کلمات هستیم. به این ترتیب میتوان اشکال گفتاری مانند کنایه را تشخیص داد یا حتی با کمک پردازش زبان، احساسات را تحلیل کرد. زبان انسان مملو از ابهاماتی است که نوشتن نرم افزاری را که به طور دقیق معنای متن را تعیین کند، بسیار دشوار میسازد. همنامها، هم آواها، کنایهها، اصطلاحات، استعارهها، گرامر و استثناهای کاربرد، تغییرات در ساختار جملات و... تنها تعداد کمی از بی نظمیهای زبان انسان هستند که یادگیری آن سالها طول میکشد، اما برنامه نویسان باید برنامههای کاربردی مبتنی بر زبان طبیعی را به گونهای آموزش دهند تا مفید باشند. اما به راستی پردازش زبان طبیعی به چه چیزی گفته میشود؟ این مقاله یک راهنمای کلی درباره همه چیزهایی است که باید در مورد پردازش زبان و ورود به این زمینه بدانید.
|
||||||
|
پردازش زبان طبیعی چیست؟
|
||||||
|
|
||||||
|
پردازش زبان طبیعی یا NLP (Natural Language Processing) شاخهای از هوش مصنوعی است که به ماشینها این امکان را میدهد تا زبانهای رایج میان انسانها را بخوانند، دادهها را درک کرده و سپس از آنها معنی استخراج کنند. روش کلی کار به این صورت است که NLP زبان شناسی و مدل سازی مبتنی بر قوانین زبان انسانی را با مدلهای آماری، یادگیری ماشین و یادگیری عمیق ترکیب میکند. این فناوریها با هم، رایانهها را قادر میسازند تا زبان انسان را در قالب متن پردازش کنند و در نهایت معنای دقیق را با هدف و احساسات نویسنده «درک» کنند. NLP زمینهای است که بر تعامل بین علم داده و زبان طبیعی میان انسانها تمرکز میکند و در صنایع زیادی در حال گسترش است. امروزه NLP به لطف پیشرفتهای عظیم در دسترسی به دادهها و افزایش قدرت محاسباتی به جنبههای مختلف زندگی وارد میشود. برای مثال NLP به پزشکان اجازه میدهد تا در زمینههایی مانند مراقبتهای بهداشتی، رسانه، مالی و منابع انسانی و غیره به نتایج معناداری دست یابند که در بخشهای بعدی به طور کامل به آن پرداخته میشود.
|
||||||
|
اهمیت نیاز به پردازش زبان طبیعی
|
||||||
|
|
||||||
|
هر چیزی که ما انسانها بیان میکنیم (چه به صورت شفاهی و چه به صورت نوشتاری) حاوی حجم عظیمی از اطلاعات است. موضوعی که انتخاب میکنیم، لحن ما، کلمات ما و... همگی شکلی از داده است که میتواند تفسیر شود و از آن اطلاعات استخراج شود. در نهایت، ما میتوانیم رفتار را با استفاده از آن اطلاعات درک کرده و حتی پیش بینی کنیم. اما یک مشکل وجود دارد: یک نفر ممکن است صدها یا هزاران کلمه را ایجاد کند و یا هر جمله را با پیچیدگی مخصوص به خود بسازد. دادههای تولید شده از مکالمات، اعلامیهها یا حتی توییتها نمونههایی از دادههای بدون ساختار هستند. دادههای بدون ساختار به خوبی در ساختار سطر و ستون سنتی پایگاههای داده رابطهای قرار نمیگیرند و جالب است بدانید که دادههای موجود در دنیای واقعی اغلب در این دسته قرار دارند. برای پردازش این نوع از داده به NLP نیاز داریم.
|
||||||
|
|
||||||
|
## تاریخچه پردازش زبان طبیعی
|
||||||
|
|
||||||
|
تاریخچه NLP به قرن هفدهم برمیگردد، زمانی که فیلسوفانی مانند لایب نیتس و دکارت پیشنهاداتی را برای کدهایی ارائه کردند که کلمات را بین زبانها مرتبط میکرد. البته تمامی این پیشنهادات در حد تئوری باقی ماندند و هیچ یک به توسعهی ماشینی واقعی منجر نشد. اولین حق ثبت اختراع در حوزه پردازش زبان در اواسط دهه 1930 انجام شد. این اختراع یک فرهنگ لغت دوزبانه خودکار با استفاده از نوار کاغذی بود که توسط ژرژ آرتسرونی توسعه یافته بود. پیشنهاد دیگر، از جانب پیتر ترویانسکی روسی و مفصلتر بود. این اختراع شامل فرهنگ لغت دوزبانه و هم روشی برای پرداختن به نقشهای دستوری بین زبانها بود. در سال 1950، آلن تورینگ مقاله معروف خود را با عنوان " ماشین آلات محاسباتی و هوش مصنوعی" منتشر کرد که امروزه آزمون تورینگ نامیده میشود. این معیار به توانایی یک برنامه رایانه ای برای جعل هویت انسان در یک مکالمه مکتوب در لحظه با یک داور انسان میپردازد.
|
||||||
|
|
||||||
|
<p align="center">
|
||||||
|
<img src="img/2.jpg" />
|
||||||
|
<br>
|
||||||
|
<br>
|
||||||
|
</p>
|
||||||
|
|
||||||
|
در سال 1957، ساختارهای نحوی نوام چامسکی، زبانشناسی را با « گرامر جهانی » متحول کرد. این ساختارها سیستمی مبتنی بر قوانین نحوی یک زبان بودند. سپس بودجه برای مدتی در حوزه پردازش زبان طبیعی به طور چشمگیری کاهش یافت و در نتیجه تحقیقات کمی در این زمینه تا اواخر دهه 1980 انجام شد. پس تا دهه 1980، اکثر سیستمهای NLP براساس مجموعهای پیچیده از قوانین دست نوشته بودند. با شروع از اواخر دهه 1980، با معرفی الگوریتمهای یادگیری ماشین در زمینه پردازش زبان، انقلابی در NLP رخ داد. بسیاری از موفقیتهای قابل توجه بعدی در این زمینه به واسطه مدلهای آماری پیچیدهتری توسعه یافتند، اتفاق افتاد. تحقیقات اخیر نیز به طور فزایندهای بر روی الگوریتمهای یادگیری بدون نظارت و نیمه نظارتی متمرکز شده است.
|
||||||
|
|
||||||
|
## کاربردهای NLPدر حوزه متن
|
||||||
|
|
||||||
|
NLP به برنامههای کامپیوتری کمک میکند تا متن را از یک زبان به زبان دیگر ترجمه کنند، به مکالمات متنی پاسخ دهند و حجم زیادی از متن را به سرعت خلاصه کنند (حتی به صورت درلحظه یا real time). دستیارهای دیجیتال، نرمافزار تصحیح، چترباتهای خدمات مشتری و سایر امکانات رفاهی مبتنی بر متن همگی از NLP استفاده میکنند. در این بخش به توضیح فواید به کارگیری پردازش زبان طبیعی در حوزه متن میپردازیم.
|
||||||
|
|
||||||
|
## تشخیص هرزنامه
|
||||||
|
|
||||||
|
در نگاه اول ممکن است تشخیص اسپم یا هرزنامه را به عنوان یکی از کاربردهای NLP در نظر نگیرید، اما بهترین فناوریهای حال حاضر (برای مثال گوگل در بخش جیمیل) برای تشخیص هرزنامه از قابلیتهای طبقه بندی متن با کمک NLP استفاده میکنند. برخی از شاخصهای طبقه بندی متن عبارتند از استفاده بیش از حد از برخی از اصطلاحات تبلیغاتی، گرامر بد، زبان تهدیدآمیز، موضوع نامناسب، نام شرکتها با املای اشتباه و... .
|
||||||
|
|
||||||
|
## ترجمه ماشینی
|
||||||
|
|
||||||
|
گوگل ترنسلیت (Google Translate) نمونهای از کاربرد مستقیم NLP است که به طور گسترده در دسترس همه قرار دارد. ترجمه ماشینی چیزی بیشتر از جایگزینی کلمات یک زبان با کلمات زبانی دیگر است. یک ترجمه مناسب باید معنی و لحن زبان ورودی را به دقت دریافت کرده و آن را به متنی با همان معنا و تاثیر دلخواه در زبان دوم ترجمه کند. ابزارهای ترجمه ماشینی از نظر دقت پیشرفت خوبی دارند. یک راه عالی برای آزمایش هر ابزار ترجمه ماشینی، ترجمه متن به یک زبان و سپس ترجمه مجدد خروجی به زبان اصلی است.
|
||||||
|
|
||||||
|
## چت باتهای گفتگو
|
||||||
|
|
||||||
|
دستیارهای مجازی مانند سیری در سیستم عامل اپل و الکسا در آمازون از تشخیص گفتار برای تشخیص الگوهای دستورات صوتی و تولید زبان طبیعی استفاده میکنند تا با اقدامات مناسب یا نظرات مفید پاسخ دهند. چت باتها همان رویکرد را در پاسخ به نوشتههای متنی تایپ شده در پیش میگیرند. بهترین چتباتها یاد میگیرند تا سرنخهای متنی را در درخواستهای انسانها تشخیص دهند و از آنها برای ارائه پاسخها یا گزینههای بهتر در طول زمان استفاده کنند. گام بعدی برای این برنامها پاسخگویی به سؤالات میباشد که شامل توانایی پاسخگویی به هر نوع سوالی - پیش بینی شده یا نشده - با پاسخهای مرتبط و مفید است.
|
||||||
|
|
||||||
|
## تجزیه و تحلیل احساسات
|
||||||
|
|
||||||
|
در سالهای اخیر NLP به یک ابزار تجاری ضروری برای کشف تاثیر دادههای پنهان به خصوص در رسانههای اجتماعی تبدیل شده است. با استفاده از تحلیل احساسات میتوان نوشتههای موجود در رسانههای اجتماعی، پاسخها و.... را برای استخراج نگرشها و احساسات در پاسخ به محصولات، تبلیغات و رویدادها تجزیه و تحلیل کرد. همچنین شرکتها میتوانند از این اطلاعات در طراحی محصول، کمپینهای تبلیغاتی و موارد دیگر استفاده کنند.
|
||||||
|
|
||||||
|
<p align="center">
|
||||||
|
<img src="img/3.jpg" />
|
||||||
|
<br>
|
||||||
|
<br>
|
||||||
|
</p>
|
||||||
|
|
||||||
|
## خلاصهسازی متن
|
||||||
|
|
||||||
|
خلاصهسازی متن از تکنیکهای NLP برای هضم حجم عظیمی از متن دیجیتالی و ایجاد خلاصههایی برای نمایهها، پایگاههای اطلاعاتی تحقیقاتی یا خوانندگان پرمشغلهای که وقت خواندن متن کامل را ندارند، استفاده میکند. بهترین برنامههای خلاصهسازی متن از استدلال معنایی و تولید زبان طبیعی (NLG) برای تولید متن با توجه به زمینه متن (اینکه متن ورزشی است یا خبری و...) و نتیجهگیری و جمع بندی استفاده میکنند.
|
||||||
|
|
||||||
|
## ابهام زدایی
|
||||||
|
|
||||||
|
فرآیند ابهام زدایی عبارت است از انتخاب معنای یک کلمه از میان معانی چندگانه از طریق یک رویکرد تحلیل معنایی. بر این اساس کلمهای انتخاب میشود که معنایش بیشترین انطباق را براساس متن داده شده دارد. برای مثال، ابهام زدایی از معنای کلمات موجود در متن استفاده میکند تا معنای کلمه "شیر" در متن را تشخیص دهد.
|
||||||
|
|
||||||
|
## شناسایی موجودیت
|
||||||
|
|
||||||
|
شناسایی موجودیت نامگذاری شده یا NEM، کلمات یا عبارات را به عنوان موجودیتهای مفید شناسایی میکند. NEM "شیراز" را به عنوان یک مکان یا "بابک" را به عنوان نام یک مرد شناسایی میکند. هرساله الگوریتمهای مفیدی در این زمینه توسعه داده میشوند.
|
||||||
|
|
||||||
|
## کاربردهای NLPدر سایر حوزهها
|
||||||
|
|
||||||
|
به زبان ساده، یک ماشین با استفاده از NLP میتواند زبان طبیعی انسان را از روی متن به طور کامل تشخیص داده و آن را درک کند. هرچند اینکه یک ماشین چطور میتواند این مسائل را تشخیص دهد به خودی خود جذاب است، اما نتایج پردازش زبان طبیعی دارای کاربردهای زیادی در زندگی روزمره است. برای مثال از NLP همچنین در هر دو مرحله جستجو و انتخاب جذب استعداد، شناسایی مهارتهای استخدامهای بالقوه و همچنین شناسایی افراد ماهر قبل از ورود به بازار کار استفاده میشود. در ادامه به چند نمونه دیگر اشاره میکنیم.
|
||||||
|
|
||||||
|
## پردازش زبان طبیعی در پزشکی
|
||||||
|
|
||||||
|
NLP تشخیص و پیش بینی بیماریها را بر اساس پرونده الکترونیکی سلامت و گفتار خود بیمار امکان پذیر میکند. این قابلیت در شرایط سلامتی مختلفی بررسی میشود؛ از بیماریهای قلبی عروقی گرفته تا افسردگی و حتی اسکیزوفرنی. به عنوان مثال، Comprehend Medical یکی از سرویسهای آمازون است که از NLP برای استخراج شرایط بیماری، داروها و نتایج درمان از یادداشتهای بیمار، گزارشهای کارآزمایی بالینی و سایر سوابق سلامت الکترونیکی استفاده میکند.
|
||||||
|
|
||||||
|
<p align="center">
|
||||||
|
<img src="img/4.jpg" />
|
||||||
|
<br>
|
||||||
|
<br>
|
||||||
|
</p>
|
||||||
|
|
||||||
|
## پردازش زبان طبیعی در سیستمهای توصیهگر
|
||||||
|
|
||||||
|
دست اندرکاران در IBM یک دستیار شناختی ایجاد کردند که با یادگیری همه چیز در مورد شما مانند یک موتور جستجوی شخصی عمل کرده و نام، آهنگ یا هر چیزی را که در لحظه ای که به آن نیاز دارید به یاد نمیآورید را به شما یادآوری میکند. یا شرکتهایی مانند یاهو و گوگل ایمیلهای شما را با تجزیه و تحلیل متن با کمک NLP فیلتر و طبقهبندی میکنند. برای کمک به شناسایی اخبار جعلی ، گروه NLP در MIT سیستم جدیدی را برای تعیین درستی یا مغرضانه بودن یک منبع ایجاد کرد و تشخیص داد که آیا میتوان به یک منبع خبری اعتماد کرد یا خیر. الکسای آمازون و سیری اپل نمونههایی از رابطهای صوتی هوشمند هستند که از NLP برای پاسخ به اعلانهای صوتی استفاده میکنند و هر کاری را انجام میدهند؛ مانند یافتن یک فروشگاه خاص، اطلاع از پیشبینی آب و هوا، پیشنهاد بهترین مسیر به دفتر یا روشن کردن چراغهای خانه.
|
||||||
|
|
||||||
|
## مفاهیم مقدماتی NLP
|
||||||
|
|
||||||
|
چالش برانگیزترین موردی که در NLP وجود دارد این است که زبان طبیعی و رایج میان انسانها بسیار پیچیده است. فرآیند درک و دستکاری یک زبان بسیار پیچیده است و به همین دلیل از تکنیکهای مختلفی استفاده میشود. زبانهای برنامهنویسی مانند پایتون (Python) یا R برای اجرای این تکنیکها بسیار مورد استفاده قرار میگیرند، اما قبل از آشنایی با چگونگی کدنویسی با این زبانها، درک مفاهیم مقدماتی نیز بسیار مهم است. به همین دلیل به شرح برخی از الگوریتمهای پرکاربرد در NLP میپردازیم.
|
||||||
|
|
||||||
|
## کیسه کلمات
|
||||||
|
|
||||||
|
کیسه کلمات (Bag of Words) یک مدل متداول است که به شما اجازه میدهد تا تمام کلمات موجود در یک متن را بشمارید. این روش یک ماتریس رخداد هر کلمه را (بدون توجه به دستور زبان و ترتیب کلمات) برای جمله یا سند ایجاد میکند. از این فرکانسها یا رخدادها به عنوان ویژگیهایی برای آموزش یک مدل طبقهبندی کننده متن استفاده میشود. این رویکرد ممکن است موجب ایجاد چندین جنبه منفی مانند از دست رفتن معنای کلمات و زمینه معنایی باشد یا اینکه به برخی از کلمات متداول (مانند the) به اشتباه امتیاز بالایی دهد.
|
||||||
|
|
||||||
|
## روش TFIDF
|
||||||
|
|
||||||
|
برای حل مشکل کیسه کلمات، یک رویکرد این است که بسامد کلمات را بر اساس تعداد دفعات ظاهر شدن آنها در همه متون (نه فقط متنی که در حال تجزیه و تحلیل ما هستیم) مجددا مقیاس بندی کنیم تا امتیازات کلمات متداول مانند "the" که در سایر متون نیز متداول است، به درستی محاسبه شود. این رویکرد امتیازدهی TFIDF نامیده میشود و مجموعه کلمات را بر اساس وزن میسنجد. از طریق TFIDF اصطلاحات مکرر در متن "پاداش" دریافت میکنند اما اگر این عبارات در متون دیگری که ما نیز در الگوریتم گنجانده ایم، تکرار شده باشند، "مجازات" میشوند. برعکس، این روش با در نظر گرفتن همه متون آزمایشی، اصطلاحات منحصر به فرد یا کمیاب را برجسته میکند و «پاداش» میدهد. هرچند بهتر است بدانید که این رویکرد هنوز هیچ زمینه و معنایی ندارد.
|
||||||
|
|
||||||
|
## توکن سازی
|
||||||
|
|
||||||
|
توکن سازی (Tokenizer) شامل فرآیند تقسیم متن به جملات و کلمات است. در اصل، وظیفه این بخش، برش یک متن به قطعاتی به نام نشانه و در عین حال دور انداختن کاراکترهای خاص مانند علائم نگارشی است. اگرچه ممکن است در زبانهایی مانند انگلیسی این فرآیند ابتدایی به نظر برسد (کافی است تا متن را براساس فضای خالی میان هر کلمه تقسیم کنید)، اما باید بدانید که همه زبانها یکسان رفتار نمیکنند و حتی در خود زبان انگلیسی هم فضاهای خالی به تنهایی کافی نیستند. برای مثال نامهای خاص (مانند سانفرانسیسکو) یا عبارات خارجی وارد شده به یک زبان این روند را دچار پیچیدگی میکنند. مسئله دیگر این است که اگر فواصل به درستی رعایت نشده باشند حذف کلمات میتواند اطلاعات مربوطه را از بین ببرد و مفهوم کلی را در یک جمله خاص تغییر دهد. به عنوان مثال، اگر در حال تجزیه و تحلیل احساسات باشید و کلمه "نه" را به اشتباه حذف کنید، ممکن است الگوریتم خود را از مسیر خارج کنید.
|
||||||
|
|
||||||
|
<p align="center">
|
||||||
|
<img src="img/5.jpg" />
|
||||||
|
<br>
|
||||||
|
<br>
|
||||||
|
</p>
|
||||||
|
|
||||||
|
## ریشه یابی
|
||||||
|
|
||||||
|
ریشه یابی یا Stemming به فرآیند برش دادن انتهای یا ابتدای کلمات به قصد حذف پیشوندها، میانوندها و پسوندها برای دستیابی به ریشه یک کلمه اشاره دارد. از ریشه یابی میتوان برای تصحیح غلطهای املایی استفاده کرد. Stemmerها برای استفاده سادهتر و سبکتر هستند، بسیار سریع اجرا میشوند و اگر سرعت و عملکرد در مدل NLP مهم است، میتوان از آن با هدف بهبود عملکرد استفاده کرد.
|
||||||
|
|
||||||
|
## Lemmatization
|
||||||
|
|
||||||
|
هدف این فرآیند تبدیل یک کلمه به شکل اصلی آن و گروه بندی اشکال مختلف یک کلمه است. برای مثال، افعال در زمان گذشته به مصدر تبدیل میشوند (مثلا «رفت» به «رفتن» تغییر میکند) و مترادفها یکسان میشوند (مثلا صفت بهترین و برترین یکی میشود)، از این رو ریشه کلماتی با معنای مشابه استاندارد میشوند. اگرچه به نظر میرسد که این روش ارتباط نزدیکی با فرآیند ریشه یابی دارد، اما Lemmatization از رویکرد متفاوتی برای رسیدن به اشکال ریشهای کلمات استفاده میکند. به عنوان مثال، کلمات " run"، "runs" و "ran" همگی اشکال کلمه " run" هستند ، بنابراین " run" لم تمام کلمات قبلی است. Lemmatization همچنین برای حل مشکلات دیگری مانند ابهام زدایی، بافت کلمه را در نظر میگیرد، به این معنی که میتواند بین کلمات یکسانی که بسته به بافت خاص معانی متفاوتی دارند، تمایز قائل شود. به کلماتی مانند "شیر" (که میتواند مربوط به حیوان یا نوشیدنی یا لوله آب باشد) فکر کنید. با ارائه یک معیار (چه اسم، یک فعل و غیره) میتوان نقشی برای آن کلمه در جمله تعریف کرد و ابهام زدایی را حذف کرد.
|
||||||
|
|
||||||
|
## مدل سازی موضوع
|
||||||
|
|
||||||
|
مدلسازی موضوع برای طبقهبندی متون، ایجاد سیستمهای توصیهگر (مثلاً برای توصیه کتابها بر اساس مطالعههای گذشتهتان) یا حتی تشخیص گرایشها در انتشارات آنلاین بسیار مفید است. مدل سازی موضوع روشی برای کشف ساختارهای پنهان در مجموعه ای از متون یا اسناد است. این روش در اصل متون را خوشه بندی میکند تا موضوعات پنهان را بر اساس محتوای آنها کشف کند، تک تک کلمات را پردازش کند و بر اساس توزیع به آنها مقادیر را اختصاص دهد. این تکنیک بر این فرض استوار است که هر سند از ترکیبی از موضوعات تشکیل شده است و هر موضوع از مجموعهای از کلمات تشکیل شده است، به این معنی که اگر بتوان این موضوعات پنهان را شناسایی کرد، میتوان به معنای متن اصلی نیز دست یافت. از میان تکنیکهای مدلسازی موضوعی، تخصیص دیریکله پنهان (LDA) احتمالاً رایجترین مورد استفاده است که در ادامه آن را معرفی میکنیم.
|
||||||
|
|
||||||
|
## الگوریتم LDA
|
||||||
|
|
||||||
|
این الگوریتم نسبتا جدید (که کمتر از 20 سال پیش اختراع شده است) به عنوان یک روش یادگیری بدون نظارت عمل میکند که موضوعات مختلف اسناد را کشف میکند. در روشهای یادگیری بدون نظارت مانند این، هیچ متغیر خروجی برای هدایت فرآیند یادگیری وجود ندارد و دادهها توسط الگوریتمها برای یافتن الگوها کاوش میشوند. برای دقیقتر بودن، LDA گروه هایی از کلمات مرتبط را بر اساس موارد زیر پیدا میکند:
|
||||||
|
|
||||||
|
- اختصاص دادن هر کلمه به یک موضوع تصادفی
|
||||||
|
- تعداد موضوعاتی که کاربر میخواهد کشف کند
|
||||||
|
|
||||||
|
الگوریتم همه اسناد را بهگونهای به موضوعات مرتبط میکند که کلمات در هر سند عمدتاً توسط آن موضوعات خیالی گرفته میشوند. الگوریتم هر کلمه را به صورت تکراری مرور میکند و با در نظر گرفتن احتمال تعلق کلمه به یک موضوع و احتمال ایجاد سند توسط یک موضوع، کلمه را دوباره به یک موضوع اختصاص میدهد. این احتمالات چندین بار تا زمان همگرایی الگوریتم محاسبه میشوند. برخلاف سایر الگوریتمهای خوشهبندی مانند K-means که خوشهبندی قطعی را انجام میدهند (موضوعات از هم جدا هستند)، LDA هر سند را به ترکیبی از موضوعات اختصاص میدهد، به این معنی که هر سند را میتوان با یک یا چند موضوع توصیف کرد و باعث میشود تا نتایج واقعیتری منعکس شود.
|
||||||
|
|
||||||
|
<p align="center">
|
||||||
|
<img src="img/6.jpg" />
|
||||||
|
<br>
|
||||||
|
<br>
|
||||||
|
</p>
|
||||||
|
|
||||||
|
# ابزارها و رویکردهای NLP
|
||||||
|
|
||||||
|
## پایتون و the Natural Language Toolkit (NLTK)
|
||||||
|
|
||||||
|
زبان برنامه نویسی پایتون طیف وسیعی از ابزارها و کتابخانهها را برای به کارگیری در وظایف خاص NLP فراهم میکند. بسیاری از این موارد در Natural Language Toolkit یا NLTK، مجموعه ای منبع باز از کتابخانه ها، برنامهها و منابع آموزشی برای ساخت برنامههای NLP پیدا میشوند. NLTK شامل کتابخانههایی برای بسیاری از وظایف NLP ذکر شده در بخشهای قبلی و همچنین کتابخانههایی برای وظایف فرعی، مانند تجزیه جملات، تقسیمبندی کلمات، ریشهیابی و ریشه یابی و توکن سازی است. پایتون همچنین شامل کتابخانههایی برای پیادهسازی قابلیتهایی مانند استدلال معنایی، توانایی رسیدن به نتایج منطقی بر اساس حقایق استخراجشده از متن است.
|
||||||
|
NLP آماری، یادگیری ماشین و یادگیری عمیق
|
||||||
|
|
||||||
|
اولین برنامههای پردازش طبیعی متن، سیستمهای مبتنی بر قواعد و کدگذاری دستی بودند که میتوانستند وظایف NLP خاصی را انجام دهند، اما نمیتوانستند به راحتی مقیاسپذیر شوند تا جریان به ظاهر بیپایانی از استثناها یا حجم فزاینده متن را در خود جای دهند. NLP آماری الگوریتمهای کامپیوتری را با مدلهای یادگیری ماشین و یادگیری عمیق ترکیب میکند تا به طور خودکار عناصر متن را استخراج، طبقهبندی و برچسبگذاری کند و سپس احتمال آماری را به هر معنای احتمالی آن عناصر اختصاص دهد. امروزه، مدلهای یادگیری عمیق و تکنیکهای یادگیری مبتنی بر شبکههای عصبی کانولوشنال (CNN) و شبکههای عصبی مکرر (RNN) سیستمهای NLP را قادر میسازند که در حین کار «یاد بگیرند» و معنای دقیقتری را از حجم عظیمی از متن خام، بدون ساختار و بدون برچسب استخراج کنند.
|
||||||
|
|
||||||
|
## جمع بندی
|
||||||
|
|
||||||
|
در حال حاضر NLP در حال تلاش برای تشخیص تفاوتهای ظریف در معنای زبان است. (به دلیل کمبود متن، اشتباهات املایی یا تفاوتهای گویش) در مارس 2016 مایکروسافت Tay را راه اندازی کرد ، یک چت ربات هوش مصنوعی (AI) که در توییتر به عنوان یک آزمایش NLP منتشر شد. ایده این بود که هرچه کاربران بیشتر با Tay صحبت کنند، هوشمندتر میشود. خوب، نتیجه این شد که پس از 16 ساعت، تای به دلیل اظهارات نژادپرستانه و توهین آمیزش حذف شد J مایکروسافت از تجربه خود استفاده کرد و چند ماه بعد Zo را منتشر کرد، نسل دوم چتبات انگلیسی زبان که دچار اشتباهات مشابه قبلی نمیشد. Zo از ترکیبی از رویکردهای نوآورانه برای شناسایی و ایجاد مکالمه استفاده میکند و سایر شرکتها در حال بررسی با رباتهایی هستند که میتوانند جزئیات خاص یک مکالمه را به خاطر بسپارند. اگرچه آینده برای NLP بسیار چالش برانگیز و پر از تهدید به نظر میرسد، این حوزه با سرعتی بسیار سریع در حال توسعه است و با ترکیب با یادگیری عمیق در سالهای آینده به سطحی از پیشرفت خواهیم رسید که ساخت برنامههای پیچیده (مانند chatgpt) ممکن میشود.
|
||||||
|
|
||||||
|
<p align="center" dir="rtl">صلوات</p>
|
||||||
|
</div>
|
360
2-Learning NLP/2-learnin_NLP.md
Normal file
|
@ -0,0 +1,360 @@
|
||||||
|
<div align="justify" dir="rtl">
|
||||||
|
|
||||||
|
<p align="center" dir="rtl">بسم الله الرحمن الرحیم</p>
|
||||||
|
|
||||||
|
# NLP چیست؟ – هر آنچه باید درباره ان ال پی بدانید
|
||||||
|
|
||||||
|
<p align="center">
|
||||||
|
<img src="img/learn/1.jpg" />
|
||||||
|
<br>
|
||||||
|
ان ال پی چیست
|
||||||
|
<br>
|
||||||
|
</p>
|
||||||
|
|
||||||
|
کسب و کارها غرق در دادههای بدون ساختار هستند، برای آنها تحلیل و پردازش همه این دادهها و اطلاعات بدون کمک «پردازش زبان طبیعی» (Natural Language Processing | NLP) غیرممکن است. با مطالعه این مطلب به فراگیری اینکه NLP چیست میپردازیم و درمییابیم که چطور ان ال پی میتواند باعث اثرگذاری بیشتر کسب و کارها شود و همچنین به محبوبیت تکنیکها و مثالهای NLP نیز پی خواهیم برد. در آخر نشان خواهیم داد که چطور میتوان از ابزارهای NLP به راحتی استفاده و مسیر حرفهای تحلیل دادههای زبانی را آغاز کرد.
|
||||||
|
|
||||||
|
## فهرست مطالب این نوشته {#FIRST}
|
||||||
|
|
||||||
|
[NLP چیست ؟](#NLP)
|
||||||
|
|
||||||
|
[چرا NLP مهم است؟](#Important)
|
||||||
|
|
||||||
|
[چالشهای NLP چیست؟](#Challenge)
|
||||||
|
|
||||||
|
[NLP چگونه کار می کند؟](#HOWWORK)
|
||||||
|
|
||||||
|
[معرفی فیلم های آموزش داده کاوی و یادگیری ماشین](#FILM)
|
||||||
|
|
||||||
|
[الگوریتم های NLP چیست ؟](#ALGURITM)
|
||||||
|
|
||||||
|
[نمونه هایی از تکنیک ها و روش های NLP](#TECHNIC)
|
||||||
|
|
||||||
|
[کاربرد های NLP چیست؟](#WORKING)
|
||||||
|
|
||||||
|
[برترین ابزار های NLP برای شروع چیست؟](#TOOLS)
|
||||||
|
|
||||||
|
[تکامل NLP](#COMPLATE)
|
||||||
|
|
||||||
|
[نکات پایانی](#END)
|
||||||
|
|
||||||
|
### NLP چیست ؟ {#NLP}
|
||||||
|
|
||||||
|
پردازش زبان طبیعی (NLP) زیرشاخهای از «هوش مصنوعی» (AI) است و به ماشینها در درک و پردازش زبان انسانها کمک میکند، تا آنها بتوانند بهصورت خودکار وظایف تکراری را انجام دهند. به عنوان مثال این وظایف شامل «ترجمه ماشینی» (Machine Translation)، «خلاصه سازی» (Summarization)، «طبقهبندی» (Classification) و «تصحیح املا» (Spell Checker) میشوند.
|
||||||
|
|
||||||
|
همانطور که گفته شد، پردازش زبان طبیعی زیرمجموعهای از هوش مصنوعی است که شامل وجه اشتراک با حوزههای «یادگیری ماشین» (Machine Learning | ML) و «یادگیری عمیق» (Deep Learning | DL) میشود؛ به طوری که برای پیادهسازی و انجام پردازش زبان طبیعی، برخی مدلها و الگوریتمهای یادگیری ماشین و یادگیری عمیق مورد نیاز هستند.
|
||||||
|
|
||||||
|
<p align="center">
|
||||||
|
<img src="img/learn/2.jpg" />
|
||||||
|
<br>
|
||||||
|
nlp در هوش مصنوعی
|
||||||
|
<br>
|
||||||
|
</p>
|
||||||
|
|
||||||
|
به عنوان مثال «تحلیل احساسات» (Sentiment Analysis) را در نظر بگیرید که در آن از پردازش زبان طبیعی برای تشخیص احساسات در متن استفاده میشود. این فرایند دستهبندی، یکی از محبوبترین روشها در حوزه NLP است که اغلب توسط کسب و کارها برای تشخیص خودکار احساسات نسبت به برندهای تجاری در رسانههای اجتماعی استفاده میشود. تجزیه و تحلیل این تعاملات میتواند به برندها کمک کند تا مسائل فوری مشتری را که باید سریعا به آنها پاسخ دهند، شناسایی کنند یا بتوانند بر رضایتمندی کلی مشتری نظارت داشته باشند.
|
||||||
|
آموزش مبانی یادگیری عمیق یا Deep Learning
|
||||||
|
فیلم آموزش مبانی یادگیری عمیق یا Deep Learning در فرادرس
|
||||||
|
کلیک کنید
|
||||||
|
|
||||||
|
## NLP مخفف چیست؟
|
||||||
|
|
||||||
|
NLP مخفف «Natural Language Processing» یعنی «پردازش زبان طبیعی» است. توسعهدهندگان این رشته تلاش میکنند تا با فهماندن زبان طبیعی انسان با استفاده از هوش مصنوعی به ماشینهای کامپیوتری، گامی بزرگ برای پیشرفت بردارند. زیرا در صورت درک زبان انسان توسط ماشینها بوسیله ان ال پی، بسیاری از کسب و کارها و پروژهها و حتی زندگی روزمره انسانها تحت تاثیر قرار میگیرد و روند رو به رشدی خواهد داشت.
|
||||||
|
|
||||||
|
## [چرا NLP مهم است؟](#FIRST){#Important}
|
||||||
|
|
||||||
|
یکی از دلایل اصلی اهمیت NLP برای کسب و کارها این است که میتوان از آن برای تجزیه و تحلیل حجم زیادی از دادههای متنی مانند نظرات رسانههای اجتماعی، بلیطهای پشتیبانی مشتری، دیدگاههای آنلاین، گزارشهای خبری و موارد دیگر استفاده کرد. همه دادههای کسب و کارها دارای انبوهی از شواهد ارزشمند هستند و NLP میتواند به کسب و کارها در کشف فوری آن شواهد کمک کند. NLP این کار را با کمک ماشینهایی که زبان انسان را درک میکنند، به روشی سریعتر، دقیقتر و سازگارتر از عوامل انسانی انجام میدهد.
|
||||||
|
|
||||||
|
ابزارهای NLP دادهها را بلادرنگ، ۲۴ ساعته و ۷ روز هفته پردازش و شاخصهای یکسانی را برای همه دادههای شما اعمال میکنند. بنابراین میتوان اطمینان حاصل کرد که نتایج بدست آمده دقیق و خالی از تناقض هستند. زمانی ابزارهای NLP میتوانند بفهمند که بخشی از متن درباره چیست، و حتی مواردی مثل احساسات آن را اندازهگیری کنند، کسب و کارها میتوانند شروع به اولویتبندی و سازماندهی دادههای خود کنند، بهطوریکه مناسب و مطابق با نیازهایشان باشد.
|
||||||
|
|
||||||
|
## [چالشهای NLP چیست؟](#FIRST){#Challenge}
|
||||||
|
|
||||||
|
با وجود چالشهای فراوان پردازش زبان طبیعی، مزایای NLP برای کسب و کارها به حدی است که NLP را به یک زمینهٔ سرمایهگذاری ارزشمند تبدیل میکند. با این حال، میبایست پیش از شروع یادگیری NLP نسبت به این چالشها آگاهی داشته باشیم.
|
||||||
|
|
||||||
|
زبان انسانی پیچیده، مبهم، بینظم و متنوع است. بیش از ۶۵۰۰ زبان در جهان وجود دارد که هر کدام از آنها قوانین سینتکسی و معنایی خاص خود را دارند. حتی خود انسانها نیز برای درک کامل زبان دچار مشکل هستند. بنابراین برای اینکه ماشین بتواند زبان طبیعی را درک کند، زبان طبیعی ابتدا باید به چیزی تبدیل شود که توسط رایانهها قابل تفسیر باشد.
|
||||||
|
|
||||||
|
در NLP، تحلیلهای سینتکسی و معنایی برای درک ساختار دستوری یک متن و شناسایی چگونگی ارتباط کلمات با یکدیگر در یک زمینه معین، امری کلیدی است. اما تبدیل متن به چیزی که توسط رایانه قابل تفسیر باشد، پیچیده است. دانشمندان داده باید ابزارهای NLP را به نحوی آموزش دهند تا فراتر از تعاریف و ترتیب کلمات، الگوریتم NLP برای درک بافت و مفهوم متن، به ابهامات کلمهای و سایر مفاهیم پیچیده مرتبط با زبان انسانی توجه کند.
|
||||||
|
|
||||||
|
<p align="center">
|
||||||
|
<img src="img/learn/3.jpg" />
|
||||||
|
<br>
|
||||||
|
پردازش زبان طبیعی چیست
|
||||||
|
<br>
|
||||||
|
</p>
|
||||||
|
|
||||||
|
وجود تعدادی از چالشهای NLP این واقعیت را اثبات میکند که زبان طبیعی، همیشه در حال تکامل و تا حدی مبهم است. این چالشها عبارتند از:
|
||||||
|
|
||||||
|
- «صحت» (Precision):
|
||||||
|
از دیرباز کامپیوترها نیاز داشتند تا انسانها با زبان برنامه نویسی دقیق، بدون ابهام و بسیار ساختار یافته یا از طریق تعداد محدودی از دستورات صوتی به وضوح بیان شده با آنها صحبت کنند. به هرحال گفتار انسان همیشه دقیق نیست؛ اغلب مبهم است و ساختار زبانی میتواند به بسیاری از متغیرهای پیچیده از جمله زبان عامیانه، گویشهای منطقهای و بافت اجتماعی بستگی داشته باشد.
|
||||||
|
|
||||||
|
- لحن صدا و «تصریف» (Inflection):
|
||||||
|
NLP هنوز کامل نشده است. برای نمونه، «تحلیل معنایی» (Semantic Analysis) هنوز میتواند یک چالش باشد. از جمله مشکلات و چالشهای دیگر NLP میتوان به این واقعیت اشاره کرد که استفاده انتزاعی از زبان معمولاً برای برنامههای کامپیوتری دشوار است. به عنوان مثال، پردازش زبان طبیعی به راحتی «طعنه» را متوجه نمیشود. این موضوعات معمولاً مستلزم درک کلمات مورد استفاده و مضمون آنها در مکالمه است. به عنوان نمونهای دیگر، یک جمله بسته به اینکه گوینده روی کدام کلمه یا هجا تاکید میکند، میتواند معنا را تغییر دهد. الگوریتمهای NLP ممکن است تغییرات ظریف اما مهم در لحن را در هنگام انجام تشخیص گفتار از دست بدهند. لحن و انحراف گفتار نیز ممکن است بین لهجههای مختلف متفاوت باشد، که تجزیه آن برای الگوریتم چالشبرانگیز است.
|
||||||
|
|
||||||
|
- استفاده رو به رشد از زبان:
|
||||||
|
پردازش زبان طبیعی نیز با این واقعیت به چالش کشیده شده است که زبان و نحوه استفاده مردم از آن، به طور مداوم در حال تغییر است. اگرچه قوانینی برای زبان وجود دارد، اما اینطور نیست که این قوانین را روی سنگ نوشته باشند و قابل تغییر نباشند بنابراین، در طول زمان در معرض تحولات زیادی قرار میگیرند. قوانین محاسباتی سختی که اکنون کار میکنند، ممکن است با تغییر ویژگیهای زبان دنیای واقعی در طول زمان منسوخ شوند.
|
||||||
|
|
||||||
|
<p align="center">
|
||||||
|
<img src="img/learn/4.jpg" />
|
||||||
|
<br>
|
||||||
|
ابهامات زبان طبیعی برای پردازش های کامپیوتری
|
||||||
|
<br>
|
||||||
|
</p>
|
||||||
|
|
||||||
|
سیستمهای کامپیوتری درکی از کلمات ندارند و برای فهماندن معانی هر کلمه به ماشینها، راه دشواری پیش روی توسعهدهندگان است. به عنوان نمونهای طنز به تصویر بالا نگاهی بیاندازید، یک ماشین چطور میتواند تفاوت بین دو مفهوم مختلفی که میتوان از جمله «I am a huge metal fan» برداشت کرد را متوجه شود، زیرا از این جمله هم میتوان برداشت کرد که یک پنکه فلزی بزرگ دارد خودش را معرفی میکند و هم ممکن است منظور این باشد که شخصی طرفدار پر و پا قرص موسیقی متال است. گنگ بودن ذاتی زبان طبیعی انسان، چالش بزرگی برای ماشینها به حساب میآید که متخصصان این حوزه همچنان در پی پیدا کردن راهحل هایی برای این موضوع هستند.
|
||||||
|
مطلب پیشنهادی:
|
||||||
|
ساخت هوش مصنوعی — آموزش کامل رایگان + نمونه پروژه
|
||||||
|
|
||||||
|
## [NLP چگونه کار می کند؟](#FIRST){#HOWWORK}
|
||||||
|
|
||||||
|
پس از دانستن چیستی NLP، به سراغ نحوه کارکرد آن میرویم. در پردازش زبان طبیعی، زبان انسانی به تکههایی تقسیم میشود به نحوی که بتوان ساختار دستوری جملات و معنای کلمات را در آن تکه متن با توجه به زمینه مفهومی متن، مورد تجزیه و تحلیل قرار داده و درک کرد. این به رایانهها کمک میکند تا متن گفتاری یا نوشتاری را به همان روش انسان خوانده و درک کنند. وظایف پیشپردازش اساسیای که دانشمندان داده میبایست انجام دهند تا ابزارهای NLP بتوانند زبان انسانی را درک کنند، عبارت است از:
|
||||||
|
|
||||||
|
- واحدسازی (Tokenization): متن را به واحدهای معنایی کوچکتر یا بندهای منفرد تقسیم میکند.
|
||||||
|
|
||||||
|
<p align="center">
|
||||||
|
<img src="img/learn/5.jpg" />
|
||||||
|
<br>
|
||||||
|
Tokenization چیست
|
||||||
|
<br>
|
||||||
|
</p>
|
||||||
|
|
||||||
|
- برچسبگذاری نقش کلمات (Part-Of-Speech tagging): کلمات را به عنوان اسم، فعل، صفت، قید، ضمایر و غیره علامتگذاری میکند.
|
||||||
|
|
||||||
|
<p align="center">
|
||||||
|
<img src="img/learn/6.jpg" />
|
||||||
|
<br>
|
||||||
|
Part-Of-Speech tagging چیست
|
||||||
|
<br>
|
||||||
|
</p>
|
||||||
|
|
||||||
|
- بنواژهسازی (Lemmatization) و ریشهیابی (Stemming): کلمات را با تبدیل آنها به شکل و فرم ریشه، استانداردسازی میکند.
|
||||||
|
|
||||||
|
<p align="center">
|
||||||
|
<img src="img/learn/7.jpg" />
|
||||||
|
<br>
|
||||||
|
Lemmatization و Stemming چیست
|
||||||
|
<br>
|
||||||
|
</p>
|
||||||
|
|
||||||
|
- حذف کلمات توقف (Stop Words): فیلتر کردن کلمات متداول که اطلاعات کم یا غیریکتایی را اضافه میکنند.
|
||||||
|
|
||||||
|
<p align="center">
|
||||||
|
<img src="img/learn/8.jpg" />
|
||||||
|
<br>
|
||||||
|
Stop Words چیست
|
||||||
|
<br>
|
||||||
|
</p>
|
||||||
|
|
||||||
|
در این صورت ابزارهای NLP میتوانند متن را به چیزی تبدیل کنند که یک رایانه بتواند آن را درک کند. مرحله بعدی، ساخت یک الگوریتم ان ال پی شرح داده خواهد شد. به نظر شما الگوریتم مناسب برای حل مسائل NLP چیست ؟
|
||||||
|
|
||||||
|
## [معرفی فیلم های آموزش داده کاوی و یادگیری ماشین](#FIRST){#FILM}
|
||||||
|
|
||||||
|
برای یادگیری هوش مصنوعی، یادگیری ماشین و داده کاوی مجموعهای آموزشی شامل چندین دوره مختلف در فرادرس ایجاد شده است که با استفاده از آنها علاقهمندان میتوانند این مباحث را به گونهای کاربردی و جامع یاد بگیرند. در این مجموعه، دورههای عملی و تئوری بسیاری وجود دارد که برای یادگیری هوش مصنوعی و یادگیری ماشین با پایتون یا متلب میتوان از آنها استفاده کرد. علاوه بر آن، بیش از ۴۰ دوره آموزشی با موضوعات مختلف هوش مصنوعی مثل شبکههای عصبی، سیستمهای فازی، داده کاوی، بهینهسازی، الگوریتم ژنتیک، خوشهبندی، انتخاب ویژگی، هوش مصنوعی توزیع شده، دستهبندی، بازشناسی الگو و بسیاری از موارد دیگر در این مجموعه در دسترس هستند.
|
||||||
|
|
||||||
|
<p align="center">
|
||||||
|
<a href="https://faradars.org/how-to-learn/machine-learning-and-data-mining?utm_source=blog.faradars&utm_medium=referral-post"><img src="img/learn/9.jpg" /> </a>
|
||||||
|
<br>
|
||||||
|
فیلمهای آموزشی
|
||||||
|
<br>
|
||||||
|
</p>
|
||||||
|
|
||||||
|
به ادامه مطلب و ارائه توضیحاتی پیرامون الگوریتمهای NLP میپردازیم.
|
||||||
|
|
||||||
|
## [الگوریتم های NLP چیست ؟](#FIRST){#ALGURITM}
|
||||||
|
|
||||||
|
پس از دانستن چیستی NLP و «پیشپردازش دادهها» (Pre-processed)، وقت آن رسیده است که به مرحله بعدی برویم؛ یعنی ساخت یک الگوریتم ان ال پی و آموزش آن به نحوی که بتواند زبان طبیعی را تفسیر کرده و وظایف خاصی را انجام دهد. دو الگوریتم اصلی برای حل مسائل NLP عبارت است از:
|
||||||
|
|
||||||
|
- «رویکرد مبتنی بر قانون» (Rule-based Approach): سیستمهای مبتنی بر قانون، به قوانین دستوری دستسازی که توسط متخصصان زبان شناسی یا «مهندسان دانش» (Knowledge Engineer) ایجاد میشود، \* متکی هستند. این اولین رویکرد برای ساخت الگوریتم های NLP بود و در حال حاضر هم امروزه بسیار مورد استفاده قرار میگیرند.
|
||||||
|
- «الگوریتمهای یادگیری ماشین» (Machine Learning Algorithms): از طرف دیگر، مدلهای یادگیری ماشین، مبتنی بر روشهای آماری هستند و یاد میگیرند که پس از دریافت نمونهها (دادههای آموزشی) وظایف خاصی را انجام دهند.
|
||||||
|
|
||||||
|
بزرگترین مزیت الگوریتمهای یادگیری ماشین، توانایی آنها برای یادگیری با اتکا به خود است. در اینجا لازم نیست قوانین دستی تعریف شوند. در عوض ماشینها از دادههای قبلی دانش را فرا میگیرند تا متکی بر خود پیشبینی کنند و در نتیجه این روشها امکان انعطافپذیری بیشتری را فراهم میکنند.
|
||||||
|
|
||||||
|
الگوریتمهای یادگیری ماشین، برای یادگیری و فهمیدن رابطه میان ورودیها و خروجیها، دادههای آموزش و خروجیهای (برچسبها) متناظر آنها را دریافت میکند. سپس ماشین، از روشهای تجزیه و تحلیل آماری برای ساختن یک "بانک دانش" استفاده میکند و پیش از آنکه دادههای از پیش دیدهنشده (متون جدید) را پیشبینی کند، تشخیص میدهد که کدام یک از ویژگیها (Features)، نمود بهتری برای متن هستند.
|
||||||
|
|
||||||
|
<p align="center">
|
||||||
|
<img src="img/learn/10.jpg" />
|
||||||
|
<br>
|
||||||
|
ان ال پی
|
||||||
|
<br>
|
||||||
|
</p>
|
||||||
|
|
||||||
|
## [نمونه هایی از تکنیک ها و روش های NLP](#FIRST){#TECHNIC}
|
||||||
|
|
||||||
|
پردازش زبان طبیعی شما را قادر میسازد تا اعمال مختلفی از جمله طبقهبندی متن و استخراج قطعاتی از دادههای مرتبط، تا ترجمه متن از یک زبان به زبان دیگر و خلاصهسازی قطعات طولانی متن را انجام دهید.
|
||||||
|
|
||||||
|
## طبقهبندی متن (Text Classification)
|
||||||
|
|
||||||
|
طبقهبندی متن یکی از اصلیترین وظایف NLP است و از تخصیص دستهها (برچسبها) به متن بر اساس محتوای آن تشکیل میشود. مدلهای طبقهبندی میتوانند اهداف مختلفی داشته باشند، برای مثال به موارد تحلیل احساسات، «طبقهبندی موضوعی» (Topic Classification) و «تشخیص قصد و قرض» (Intent Detection) اشاره خواهیم کرد و در ادامه توضیح مفصلتری درباره هر یک میدهیم.
|
||||||
|
آموزش پردازش زبان های طبیعی NLP در پایتون Python با پلتفرم NLTK
|
||||||
|
فیلم آموزش پردازش زبان های طبیعی NLP در پایتون Python با پلتفرم NLTK در فرادرس
|
||||||
|
کلیک کنید
|
||||||
|
|
||||||
|
## تحلیل احساسات (Sentiment Analysis)
|
||||||
|
|
||||||
|
تحلیل احساسات فرآیند بررسی عواطف موجود در متن و طبقهبندی آنها به عنوان مثبت، منفی یا خنثی است. با اجرای تجزیه و تحلیل احساسات در پستهای رسانههای اجتماعی، دیدگاههای محصول، نظرسنجیهای (Net Promotor Score | NPS) و بازخورد مشتریان، کسبوکارها میتوانند شواهد ارزشمند بودن سرمایه خود را درباره چگونگی درک برند آنها توسط مشتریان دریافت کنند.
|
||||||
|
|
||||||
|
مجهز بودن به NLP، یک طبقهبندی احساسات میتواند تفاوت ظریفی که در هر نظر و عقیدهای وجود دارد را درک کند، و به طور خودکار دیدگاهها را به عنوان مثبت یا منفی برچسبگذاری کند. تصور کنید یک جهش ناگهانی از نظرات منفی درباره برند شما در رسانههای اجتماعی شکل گرفته باشد، ابزارهای تحلیل احساسات توانایی تشخیص این اتفاقات را به سرعت دارند، و با استفاده از آنها میتوان از بروز مشکلات بزرگتر جلوگیری کرد.
|
||||||
|
|
||||||
|
<p align="center">
|
||||||
|
<img src="img/learn/11.jpg" />
|
||||||
|
<br>
|
||||||
|
تحلیل احساسات nlp
|
||||||
|
<br>
|
||||||
|
</p>
|
||||||
|
|
||||||
|
## طبقهبندی موضوعی (Topic Classification)
|
||||||
|
|
||||||
|
طبقهبندی موضوعی از شناسایی موضوع یا مبحث اصلی داخل متن و اختصاص تگهای از پیش تعریف شده برای آنها تشکیل میشود. برای آموزش مدل طبقهبندی کننده موضوع خود، نیاز به آشنایی با تجزیه و تحلیل دادهها دارید، بنابراین میتوانید دستهبندیهای مربوطه را تعریف کنید. برای مثال، ممکن است در یک شرکت نرمافزاری مشغول باشید و تعدادی زیادی بلیط پشتیبانی مشتری دریافت کنید که به مشکلات فنی، قابلیت استفاده و درخواستهای ویژگی اشاره میکند. در این مورد ممکن است برچسبها به عنوان اشکالات، ویژگیها، درخواستها، «طراحی تعامل/تجربه کاربری» (UX/IX | User Experience/Interaction Design) تعریف شوند.
|
||||||
|
|
||||||
|
<p align="center">
|
||||||
|
<img src="img/learn/12.jpg" />
|
||||||
|
<br>
|
||||||
|
طبقه بندی موضوعی nlp
|
||||||
|
<br>
|
||||||
|
</p>
|
||||||
|
|
||||||
|
## تشخیص قصد (Intent Detection)
|
||||||
|
|
||||||
|
تشخیص قصد شامل شناسایی مفهوم، منظور و هدف پشت یک متن است. یک راه بسیار خوب برای مرتبسازی خروجی پاسخهای ایمیل فروش، براساس علاقهمندی، نیاز به اطلاعات بیشتر، لغو اشتراک، برگشتن زدن و غیره است. برچسب علاقهمندی میتواند به شما کمک کند تا به محض اینکه ایمیلی وارد صندوق ورودی شما شد، پتانسیل بالقوه فرصت فروش را پیدا کنید.
|
||||||
|
|
||||||
|
## استخراج متن (Text Extraction)
|
||||||
|
|
||||||
|
نمونه دیگری از استفادههای NLP در استخراج متن وجود دارد، که شامل بیرون کشیدن قطعات خاصی از دادههایی است که قبلاً در یک متن وجود داشتند. این یک راه عالی برای خلاصهسازی خودکار متن یا پیدا کردن اطلاعات کلیدی است. رایجترین نمونههای مدلهای استخراج عبارت از «استخراج کلمات کلیدی» (Keyword Extraction) و «تشخیص موجودیتهای نامدار» (Named Entity Recognition | NER) است که در ادامه توضیحات بیشتری درمورد آنها خواهیم خواند.
|
||||||
|
|
||||||
|
## استخراج کلمات کلیدی (Keyword Extraction)
|
||||||
|
|
||||||
|
استخراج کلمات کلیدی بهطور خودکار مهمترین کلمات و عبارات داخل یک متن را بیرون میکشد. این مسئله برای شما قابلیت دستهبندی از پیش نمایش محتوا و موضوعات اصلی آن، بدون نیاز به خواندن هر قطعه را فراهم میکند.
|
||||||
|
|
||||||
|
<p align="center">
|
||||||
|
<img src="img/learn/13.jpg" />
|
||||||
|
<br>
|
||||||
|
استخراج کلمات کلیدی nlp
|
||||||
|
<br>
|
||||||
|
</p>
|
||||||
|
|
||||||
|
## تشخیص موجودیت های نامدار Named Entity Recognition (NER)
|
||||||
|
|
||||||
|
تشخیص موجودیتهای نامدار، امکان استخراج نام افراد، شرکتها، مکانها و سایر موارد را از داخل دادهها میدهد.
|
||||||
|
تشخیص موجودیت های نامدار nlp
|
||||||
|
|
||||||
|
## ترجمه ماشینی (Machine Translation)
|
||||||
|
|
||||||
|
این یکی از اولین مشکلاتی بود که محققان NLP به آن پرداختند. ابزارهای ترجمه آنلاین (مانند Google Translate) از تکنیکهای مختلف پردازش زبان طبیعی برای دستیابی به سطوح انسانی از دقت در ترجمه گفتار و متن به زبانهای مختلف استفاده میکنند. مدلهای مترجم سفارشی میتوانند برای به حداکثر رساندن دقت نتایج یک حوزه خاص آموزش داده شوند.
|
||||||
|
|
||||||
|
<p align="center">
|
||||||
|
<img src="img/learn/14.jpg" />
|
||||||
|
<br>
|
||||||
|
ترجمه ماشینی nlp
|
||||||
|
<br>
|
||||||
|
</p>
|
||||||
|
|
||||||
|
## مدل سازی موضوعی (Topic Modeling)
|
||||||
|
|
||||||
|
مدل سازی موضوعی بسیار شبیه طبقهبندی موضوعی است. این نمونه از پردازش زبان طبیعی با گروهبندی متنها بر اساس کلمات و عبارات مشابه، موضوعات مرتبط را در یک متن پیدا میکند. از آنجایی که نیازی به ایجاد لیستی از تگهای از پیش تعریف شده یا برچسبگذاری هیچ دادهای ندارید، زمانی که هنوز با دادههای خود آشنا نیستید، مدلسازی موضوعی گزینه مناسبی برای تجزیه و تحلیل کندوکاوانه در متن است.
|
||||||
|
|
||||||
|
<p align="center">
|
||||||
|
<img src="img/learn/15.jpg" />
|
||||||
|
<br>
|
||||||
|
مدل سازی موضوعی nlp
|
||||||
|
<br>
|
||||||
|
</p>
|
||||||
|
|
||||||
|
## تولید زبان طبیعی در NLP چیست ؟
|
||||||
|
|
||||||
|
تولید زبان طبیعی، به اختصار NLG، یکی از وظایف پردازش زبان طبیعی است که شامل تحلیل دادههای بدون ساختار است و از آن به عنوان ورودی خودکار برای ساختن محتوا استفاده میشود. از کاربردهای این مورد میتوان به تولید پاسخهای خودکار، نوشتن ایمیل و حتی کتاب اشاره کرد.
|
||||||
|
|
||||||
|
<p align="center">
|
||||||
|
<img src="img/learn/16.jpg" />
|
||||||
|
<br>
|
||||||
|
تولید زبان طبیعی nlp
|
||||||
|
<br>
|
||||||
|
</p>
|
||||||
|
|
||||||
|
## [کاربرد های NLP چیست؟](#FIRST){#WORKING}
|
||||||
|
|
||||||
|
پردازش زبان طبیعی به کسب و کارها اجازه میدهد تا دادههای بدون ساختار مانند ایمیل، پستهای رسانههای اجتماعی، بررسیهای محصول، نظرسنجیهای آنلاین و بلیطهای پشتیبانی مشتری را تحلیل
|
||||||
|
و درک کنند و اطلاعات ارزشمندی را برای ارتقاء فرایندهای تصمیمگیری خود بدست آورند. شرکتها همچنین پس از دانستن اینکه NLP چیست، از آن برای خودکارسازی وظایف روزمره، کاهش زمان، هزینه و در نهایت کارآمدتر شدن، استفاده میکنند. در ادامه چند نمونه از کاربردهای NLP در مشاغل را بررسی خواهیم نمود.
|
||||||
|
تحلیل خودکار بازخورد مشتریان در NLP چیست ؟
|
||||||
|
|
||||||
|
تجزیه و تحلیل خودکار بازخورد مشتری برای دانستن اینکه مشتریان دربارهٔ محصول شما چه فکری میکنند ضروری است. با این حال، پردازش این دادهها ممکن است دشوار باشد. NLP میتواند به شما در استفاده از دادههای کیفی در نظرسنجیهای آنلاین، بررسی محصول یا پستهای رسانه های اجتماعی کمک کند و برای بهبود تجارت خود اطلاعات کسب کنید.
|
||||||
|
|
||||||
|
به عنوان مثال، شاخص «NPS | Net Promoter Score» اغلب برای اندازهگیری رضایت مشتریها استفاده میشود. در مرحله اول، از مشتریان خواسته می شود كه از صفر تا ده، شرکتی را بر اساس اینكه احتمالاً آن را به یك دوست توصیه میكنند، امتیازدهی کنند (امتیازهای پایین به عنوان دفعکنندهها، امتیاز متوسط به عنوان خنثی و امتیازات بالا به عنوان ترویجکنندهها طبقهبندی میشوند). سپس با یک سؤال پایانباز، دلایل نمره خود را از مشتریان میپرسند.
|
||||||
|
|
||||||
|
با استفاده از یک طبقهبندیکننده موضوع NLP، میتوانید هر پاسخ پایانباز را به گروههایی مانند UX محصول، پشتیبانی مشتری، سهولت استفاده و غیره برچسب گذاری کنید، سپس، این دادهها را در دستههای ترویجکننده، دفعکننده و خنثی طبقهبندی کنید تا ببینید که هر دسته در کدام گروه شایعتر است:
|
||||||
|
|
||||||
|
در این مثال، در بالا، نتایج نشان میدهد که مشتریان از جنبههایی مانند سهولت استفاده و UX محصول بسیار راضی هستند (از آنجا که بیشتر این پاسخها از طرف ترویجکنندهها هستند)، در حالی که از سایر ویژگیهای محصول رضایت چندانی ندارند.
|
||||||
|
عملیات خودکار پشتیبانی از مشتری در NLP چیست ؟
|
||||||
|
|
||||||
|
کسب و کارها از مدلهای NLP برای خودکارسازی وظایف خستهکننده و وقتگیر در زمینههایی مانند خدمات مشتریان استفاده میکنند. این منجر به فرآیندهای کارآمدتری میشود و نمایندگان پشتیبانی، زمان بیشتری را برای تمرکز روی آنچه مهم است، یعنی «ارائه تجربهٔ پشتیبانی برجسته» صرف خواهند کرد. اتوماسیون خدمات مشتری با استفاده از ان ال پی مجموعهای از فرآیندها، از مسیریابی تیکتها به مناسبترین فرد گرفته تا استفاده از چتبات برای حل سؤالات مکرر را شامل میشود. در ادامه چند مثال در این خصوص ارائه شده است.
|
||||||
|
|
||||||
|
- مدلهای طبقهبندی متن به شرکتها امکان میدهد تیکتهای پشتیبانی را بر اساس معیارهای مختلف، مانند موضوع، احساسات یا زبان برچسبگذاری کرده و تیکت به مناسبترین نمایندهٔ پشتیانی ارسال شود. به عنوان مثال، یک شرکت تجارت الکترونیک ممکن است از یک طبقهبندیکننده موضوع استفاده کند تا تیکت پشتیبانی به مشکل حمل و نقل، کالای گمشده یا کالای برگشتی از سایر دستهها تفکیک شود.
|
||||||
|
|
||||||
|
- همچنین میتوان از طبقهبندها برای تشخیص فوریت در بلیطهای پشتیبانی مشتری با شناخت عباراتی مانند "در اسرع وقت، بلافاصله یا همین حالا" استفاده کرد و این امر به نمایندگان پشتیبانی اجازه میدهد که ابتدا این موارد را بررسی کنند.
|
||||||
|
|
||||||
|
- تیمهای پشتیبانی مشتری به طور فزایندهای از چتباتها برای رسیدگی به سؤالات روزمره استفاده میکنند. این امر باعث کاهش هزینهها میشود و نمایندگان پشتیبانی را قادر میسازد تا بیشتر روی وظایفی تمرکز کنند که نیاز به شخصیسازی بیشتری دارند و در نتیجه زمان انتظار مشتری کاهش مییابد.
|
||||||
|
|
||||||
|
<p align="center">
|
||||||
|
<img src="img/learn/16.jpg" />
|
||||||
|
<br>
|
||||||
|
کاربرد های nlp
|
||||||
|
<br>
|
||||||
|
</p>
|
||||||
|
|
||||||
|
## [برترین ابزار های NLP برای شروع چیست؟](#FIRST){#TOOLS}
|
||||||
|
|
||||||
|
پردازش زبان طبیعی یکی از پیچیدهترین زمینههای هوش مصنوعی است. اما نیازی به ورود مستقیم در بسیاری از وظایف NLP مانند تجزیه و تحلیل احساسات یا استخراج کلمات کلیدی ندارد. ابزارهای آنلاین پردازش زبان طبیعی بسیاری وجود دارند که پردازش زبان را در دسترس همه قرار میدهند و این امکان را فراهم میکنند که حجم زیادی از دادهها به روشی بسیار ساده و بصری تجزیه و تحلیل شوند.
|
||||||
|
|
||||||
|
پلتفرمهای «نرمافزار به عنوان یک سرویس» (SaaS) جایگزینهای بسیار خوبی برای کتابخانههای منبع باز هستند، زیرا آنها راهحلهای آماده استفادهای را ارائه میدهند که اغلب برای بهکارگیری، بسیار آسان هستند و به برنامهنویسی یا دانش یادگیری ماشین احتیاج ندارند.
|
||||||
|
آموزش یادگیری ماشین
|
||||||
|
فیلم آموزش یادگیری ماشین در فرادرس
|
||||||
|
کلیک کنید
|
||||||
|
|
||||||
|
بیشتر این ابزارها، APIهای NLP خود را برای زبان برنامهنویسی پایتون ارائه میدهند که تنها با وارد کردن چند خط کد در کد منبع، با برنامههای روزمرهٔ خود، قابلیت ادغام دارند. چند مورد از بهترین ابزارهای SaaS پردازش زبان طبیعی عبارتند از:
|
||||||
|
|
||||||
|
- Google Cloud NLP
|
||||||
|
- IBM Watson
|
||||||
|
- Aylien
|
||||||
|
- Amazon Comprehend
|
||||||
|
- MeaningCloud
|
||||||
|
|
||||||
|
انتخاب ابزار NLP، بستگی به احساس راحتی هنگام استفاده از آن و وظایفی دارد که میخواهید انجام دهید. به عنوان مثال، Google Cloud NLP مجموعهای از ابزارهای NLP بدون نیاز به کد را ارائه میدهد که به راحتی برای کاربران قابل استفاده است. پس از فراگیری این ابزارها، میتوان یک مدل یادگیری ماشین سفارشی ساخت و آن را با معیارهای خود آموزش داد تا نتایج دقیقتری بدست آید.
|
||||||
|
|
||||||
|
<p align="center">
|
||||||
|
<img src="img/learn/16.jpg" />
|
||||||
|
<br>
|
||||||
|
nlp چیست
|
||||||
|
<br>
|
||||||
|
</p
|
||||||
|
|
||||||
|
در بخش بعدی مطلب NLP چیست به بحث تکامل NLP در طول زمان پرداخته شده است.
|
||||||
|
|
||||||
|
## [تکامل NLP](#FIRST){#COMPLATE}
|
||||||
|
|
||||||
|
پردازش زبان طبیعی ریشه در رشتههای مختلفی، از جمله علوم کامپیوتر و زبانشناسی محاسباتی دارد که به اواسط قرن بیستم باز میگردند. تکامل این حوزه شامل نقاط عطف زیر است:
|
||||||
|
|
||||||
|
- دهه ۵۰ میلادی:
|
||||||
|
ریشههای پردازش زبان طبیعی به این دهه باز میگردد، هنگامی که آلن تورینگ، تست تورینگ را به منظور بررسی هوشمندی رایانهها توسعه داد. این آزمایش شامل تفسیر خودکار و توسعهٔ زبان طبیعی به عنوان معیار هوشمندی بود.
|
||||||
|
|
||||||
|
- دهههای ۵۰ تا ۹۰ میلادی:
|
||||||
|
NLP تا حد زیادی مبتنی بر قوانین بود؛ قوانینی دستساز و ساختهشده توسط زبانشناسان برای تعیین چگونگی پردازش زبان در رایانهها
|
||||||
|
|
||||||
|
- دهه ۹۰ میلادی:
|
||||||
|
رویکرد بالا به پایین پردازش زبان طبیعی با یک رویکرد آماریتر جایگزین شد، زیرا پیشرفت در محاسبات، این روش را به روشی کارآمدتر برای توسعه فناوری NLP تبدیل کرده بود. رایانهها سریعتر شده و میتوانستند برای تدوین قوانین آماری زبان بدون نیاز به زبانشناس، مورد استفاده قرار گیرند. پردازش زبان طبیعی مبتنی بر داده، طی این دهه به جریان اصلی تبدیل شد. پردازش زبان طبیعی از یک رویکرد مبتنی بر زبانشناسی به یک رویکرد مبتنی بر مهندسی تبدیل شده و به جای آنکه تنها به زبانشناسی بپردازد، طیف گستردهتری از رشتههای علمی را ترسیم میکند.
|
||||||
|
|
||||||
|
- سالهای ۲۰۰۰ تا ۲۰۲۰ میلادی:
|
||||||
|
مجبوبیت پردازش زبان طبیعی در این سالها به شدت افزایش پیدا کرده است. پس از دانستن این موضوع که NLP چیست و با پیشرفتهای توان محاسباتی، پردازش زبان طبیعی کاربردهای فراوانی در دنیای واقعی به دست آورده است. امروزه، رویکردهای NLP شامل ترکیبی از زبانشناسی کلاسیک و روشهای آماری است.
|
||||||
|
|
||||||
|
ان ال پی نقش مهمی در فناوری و نحوه تعامل انسان با آن دارد. حال پردازش زبان طبیعی، در بسیاری از کاربردهای دنیای واقعی در هر دو فضای کسبکارها و مصرفکنندهها قابل استفاده است، از این کاربردها میتوان به چتباتها، امنیت سایبری، موتورهای جستجو و تجزیه و تحلیل دادههای کلان اشاره نمود. بدون درنظر گرفتن چالشهای آن، انتظار می رود NLP همچنان بخش مهمی از صنعت و زندگی روزمره آینده را تشکیل دهد.
|
||||||
|
|
||||||
|
همچنین با وجود تمام تردیدها، پردازش زبان طبیعی در زمینه تصویربرداری پزشکی نیز، پیشرفتهای قابل توجهی داشته است. برای مثال رادیولوژیستها از هوش مصنوعی و پردازش زبان طبیعی بهره میبرند تا نتایج خود را مرور کرده و آنها را با یکدیگر مقایسه کنند.
|
||||||
|
آموزش اصول و روش های متن کاوی Text Mining
|
||||||
|
فیلم آموزش اصول و روش های متن کاوی Text Mining در فرادرس
|
||||||
|
کلیک کنید
|
||||||
|
|
||||||
|
به این ترتیب در بخش انتهایی مطلب NLP چیست به نکات پایانی اشاره شده است.
|
||||||
|
|
||||||
|
## [نکات پایانی](#FIRST){#END}
|
||||||
|
|
||||||
|
پردازش زبان طبیعی یکی از امیدوار کنندهترین زمینهها در هوش مصنوعی به حساب میآید، و در حال حاضر در بسیاری از برنامههایی که ما بهصورت روزانه از آنها استفاده میکنیم، از چتباتها گرفته تا موتورهای جستجو، کاربرد دارد. به لطف NLP، کسب و کارها برخی از فرآیندهای روزانه خود را خودکارسازی میکنند و از اغلب دادههای بدون ساختار خود، شواهد عملیاتیای دریافت میکنند، که میتوان برای ایجاد بهبود رضایت مشتری و ارائه تجربیات بهتر آنها از این شواهد استفاده کرد.
|
||||||
|
|
||||||
|
باوجود پیچیدگیهای موجود در NLP، این زمینه به لطف ابزارهای آنلاین روز به روز برای کاربران دست یافتنیتر میشود، که به سادگی میتوان برای مدلهای سفارشی شده به عنوان وظایف طبقهبندی و استخراج متون آن را ایجاد کرد. در این مطلب یاد گرفتیم که NLP چیست و هر اطلاعاتی را کسب کردیم که در وهله اول نیاز به دانستن آن در زمینه پردازش زبان طبیعی وجود داشت.
|
||||||
|
|
||||||
|
<p align="center" dir="rtl">صلوات</p>
|
||||||
|
</div>
|
BIN
2-Learning NLP/img/1.jpg
Normal file
After Width: | Height: | Size: 147 KiB |
BIN
2-Learning NLP/img/2.jpg
Normal file
After Width: | Height: | Size: 91 KiB |
BIN
2-Learning NLP/img/3.jpg
Normal file
After Width: | Height: | Size: 86 KiB |
BIN
2-Learning NLP/img/4.jpg
Normal file
After Width: | Height: | Size: 72 KiB |
BIN
2-Learning NLP/img/5.jpg
Normal file
After Width: | Height: | Size: 85 KiB |
BIN
2-Learning NLP/img/6.jpg
Normal file
After Width: | Height: | Size: 94 KiB |
BIN
2-Learning NLP/img/learn/1.jpg
Normal file
After Width: | Height: | Size: 137 KiB |
BIN
2-Learning NLP/img/learn/10.jpg
Normal file
After Width: | Height: | Size: 20 KiB |
BIN
2-Learning NLP/img/learn/11.jpg
Normal file
After Width: | Height: | Size: 7.1 KiB |
BIN
2-Learning NLP/img/learn/12.jpg
Normal file
After Width: | Height: | Size: 6.8 KiB |
BIN
2-Learning NLP/img/learn/13.jpg
Normal file
After Width: | Height: | Size: 8.7 KiB |
BIN
2-Learning NLP/img/learn/14.jpg
Normal file
After Width: | Height: | Size: 17 KiB |
BIN
2-Learning NLP/img/learn/15.jpg
Normal file
After Width: | Height: | Size: 9.1 KiB |
BIN
2-Learning NLP/img/learn/16.jpg
Normal file
After Width: | Height: | Size: 7.8 KiB |
BIN
2-Learning NLP/img/learn/17.jpg
Normal file
After Width: | Height: | Size: 9.5 KiB |
BIN
2-Learning NLP/img/learn/18.jpg
Normal file
After Width: | Height: | Size: 25 KiB |
BIN
2-Learning NLP/img/learn/19.jpg
Normal file
After Width: | Height: | Size: 40 KiB |
BIN
2-Learning NLP/img/learn/2.jpg
Normal file
After Width: | Height: | Size: 17 KiB |
BIN
2-Learning NLP/img/learn/3.jpg
Normal file
After Width: | Height: | Size: 24 KiB |
BIN
2-Learning NLP/img/learn/4.jpg
Normal file
After Width: | Height: | Size: 18 KiB |
BIN
2-Learning NLP/img/learn/5.jpg
Normal file
After Width: | Height: | Size: 8.2 KiB |
BIN
2-Learning NLP/img/learn/6.jpg
Normal file
After Width: | Height: | Size: 7.7 KiB |
BIN
2-Learning NLP/img/learn/7.jpg
Normal file
After Width: | Height: | Size: 22 KiB |
BIN
2-Learning NLP/img/learn/8.jpg
Normal file
After Width: | Height: | Size: 7.2 KiB |
BIN
2-Learning NLP/img/learn/9.jpg
Normal file
After Width: | Height: | Size: 93 KiB |
228
3-NLP_services/.gitignore
vendored
Normal file
|
@ -0,0 +1,228 @@
|
||||||
|
### JetBrains template
|
||||||
|
# Covers JetBrains IDEs: IntelliJ, RubyMine, PhpStorm, AppCode, PyCharm, CLion, Android Studio, WebStorm and Rider
|
||||||
|
# Reference: https://intellij-support.jetbrains.com/hc/en-us/articles/206544839
|
||||||
|
|
||||||
|
|
||||||
|
|
||||||
|
|
||||||
|
# User-specific stuff
|
||||||
|
.idea/**/workspace.xml
|
||||||
|
.idea/**/tasks.xml
|
||||||
|
.idea/**/usage.statistics.xml
|
||||||
|
.idea/**/dictionaries
|
||||||
|
.idea/**/shelf
|
||||||
|
|
||||||
|
# Generated files
|
||||||
|
.idea/**/contentModel.xml
|
||||||
|
|
||||||
|
*.jsonl
|
||||||
|
*.7zip
|
||||||
|
*.7z
|
||||||
|
.idea/
|
||||||
|
fastapi_flair/idea/
|
||||||
|
|
||||||
|
# Sensitive or high-churn files
|
||||||
|
.idea/**/dataSources/
|
||||||
|
.idea/**/dataSources.ids
|
||||||
|
.idea/**/dataSources.local.xml
|
||||||
|
.idea/**/sqlDataSources.xml
|
||||||
|
.idea/**/dynamic.xml
|
||||||
|
.idea/**/uiDesigner.xml
|
||||||
|
.idea/**/dbnavigator.xml
|
||||||
|
|
||||||
|
# Gradle
|
||||||
|
.idea/**/gradle.xml
|
||||||
|
.idea/**/libraries
|
||||||
|
|
||||||
|
# Gradle and Maven with auto-import
|
||||||
|
# When using Gradle or Maven with auto-import, you should exclude module files,
|
||||||
|
# since they will be recreated, and may cause churn. Uncomment if using
|
||||||
|
# auto-import.
|
||||||
|
# .idea/artifacts
|
||||||
|
# .idea/compiler.xml
|
||||||
|
# .idea/jarRepositories.xml
|
||||||
|
# .idea/modules.xml
|
||||||
|
# .idea/*.iml
|
||||||
|
# .idea/modules
|
||||||
|
# *.iml
|
||||||
|
# *.ipr
|
||||||
|
|
||||||
|
# CMake
|
||||||
|
cmake-build-*/
|
||||||
|
|
||||||
|
# Mongo Explorer plugin
|
||||||
|
.idea/**/mongoSettings.xml
|
||||||
|
|
||||||
|
# File-based project format
|
||||||
|
*.iws
|
||||||
|
|
||||||
|
# IntelliJ
|
||||||
|
out/
|
||||||
|
|
||||||
|
# mpeltonen/sbt-idea plugin
|
||||||
|
.idea_modules/
|
||||||
|
|
||||||
|
# JIRA plugin
|
||||||
|
atlassian-ide-plugin.xml
|
||||||
|
|
||||||
|
# Cursive Clojure plugin
|
||||||
|
.idea/replstate.xml
|
||||||
|
|
||||||
|
# Crashlytics plugin (for Android Studio and IntelliJ)
|
||||||
|
com_crashlytics_export_strings.xml
|
||||||
|
crashlytics.properties
|
||||||
|
crashlytics-build.properties
|
||||||
|
fabric.properties
|
||||||
|
|
||||||
|
# Editor-based Rest Client
|
||||||
|
.idea/httpRequests
|
||||||
|
|
||||||
|
# Android studio 3.1+ serialized cache file
|
||||||
|
.idea/caches/build_file_checksums.ser
|
||||||
|
|
||||||
|
### Python template
|
||||||
|
# Byte-compiled / optimized / DLL files
|
||||||
|
__pycache__/
|
||||||
|
*.py[cod]
|
||||||
|
*$py.class
|
||||||
|
|
||||||
|
# C extensions
|
||||||
|
*.so
|
||||||
|
|
||||||
|
# Distribution / packaging
|
||||||
|
.Python
|
||||||
|
build/
|
||||||
|
develop-eggs/
|
||||||
|
dist/
|
||||||
|
downloads/
|
||||||
|
eggs/
|
||||||
|
.eggs/
|
||||||
|
lib/
|
||||||
|
lib64/
|
||||||
|
parts/
|
||||||
|
sdist/
|
||||||
|
var/
|
||||||
|
wheels/
|
||||||
|
share/python-wheels/
|
||||||
|
*.egg-info/
|
||||||
|
.installed.cfg
|
||||||
|
*.egg
|
||||||
|
MANIFEST
|
||||||
|
|
||||||
|
# PyInstaller
|
||||||
|
# Usually these files are written by a python script from a template
|
||||||
|
# before PyInstaller builds the exe, so as to inject date/other infos into it.
|
||||||
|
*.manifest
|
||||||
|
*.spec
|
||||||
|
|
||||||
|
# Installer logs
|
||||||
|
pip-log.txt
|
||||||
|
pip-delete-this-directory.txt
|
||||||
|
|
||||||
|
# Unit test / coverage reports
|
||||||
|
htmlcov/
|
||||||
|
.tox/
|
||||||
|
.nox/
|
||||||
|
.coverage
|
||||||
|
.coverage.*
|
||||||
|
.cache
|
||||||
|
nosetests.xml
|
||||||
|
coverage.xml
|
||||||
|
*.cover
|
||||||
|
*.py,cover
|
||||||
|
.hypothesis/
|
||||||
|
.pytest_cache/
|
||||||
|
cover/
|
||||||
|
|
||||||
|
# Translations
|
||||||
|
*.mo
|
||||||
|
*.pot
|
||||||
|
|
||||||
|
# Django stuff:
|
||||||
|
*.log
|
||||||
|
local_settings.py
|
||||||
|
db.sqlite3
|
||||||
|
db.sqlite3-journal
|
||||||
|
|
||||||
|
# Flask stuff:
|
||||||
|
instance/
|
||||||
|
.webassets-cache
|
||||||
|
|
||||||
|
# Scrapy stuff:
|
||||||
|
.scrapy
|
||||||
|
|
||||||
|
# Sphinx documentation
|
||||||
|
docs/_build/
|
||||||
|
|
||||||
|
# PyBuilder
|
||||||
|
.pybuilder/
|
||||||
|
target/
|
||||||
|
|
||||||
|
# Jupyter Notebook
|
||||||
|
.ipynb_checkpoints
|
||||||
|
|
||||||
|
# IPython
|
||||||
|
profile_default/
|
||||||
|
ipython_config.py
|
||||||
|
|
||||||
|
# pyenv
|
||||||
|
# For a library or package, you might want to ignore these files since the code is
|
||||||
|
# intended to run in multiple environments; otherwise, check them in:
|
||||||
|
# .python-version
|
||||||
|
|
||||||
|
# pipenv
|
||||||
|
# According to pypa/pipenv#598, it is recommended to include Pipfile.lock in version control.
|
||||||
|
# However, in case of collaboration, if having platform-specific dependencies or dependencies
|
||||||
|
# having no cross-platform support, pipenv may install dependencies that don't work, or not
|
||||||
|
# install all needed dependencies.
|
||||||
|
#Pipfile.lock
|
||||||
|
|
||||||
|
# PEP 582; used by e.g. github.com/David-OConnor/pyflow
|
||||||
|
__pypackages__/
|
||||||
|
|
||||||
|
# Celery stuff
|
||||||
|
celerybeat-schedule
|
||||||
|
celerybeat.pid
|
||||||
|
|
||||||
|
# SageMath parsed files
|
||||||
|
*.sage.py
|
||||||
|
|
||||||
|
# Environments
|
||||||
|
.env
|
||||||
|
.venv
|
||||||
|
env/
|
||||||
|
venv/
|
||||||
|
ENV/
|
||||||
|
env.bak/
|
||||||
|
venv.bak/
|
||||||
|
|
||||||
|
# Spyder project settings
|
||||||
|
.spyderproject
|
||||||
|
.spyproject
|
||||||
|
|
||||||
|
# Rope project settings
|
||||||
|
.ropeproject
|
||||||
|
|
||||||
|
# mkdocs documentation
|
||||||
|
/site
|
||||||
|
|
||||||
|
# mypy
|
||||||
|
.mypy_cache/
|
||||||
|
.dmypy.json
|
||||||
|
dmypy.json
|
||||||
|
|
||||||
|
# Pyre type checker
|
||||||
|
.pyre/
|
||||||
|
|
||||||
|
# pytype static type analyzer
|
||||||
|
.pytype/
|
||||||
|
|
||||||
|
# Cython debug symbols
|
||||||
|
cython_debug/
|
||||||
|
|
||||||
|
data/ARMAN-MSR-persian-base-PN-summary
|
||||||
|
data/mT5_multilingual_XLSum
|
||||||
|
data/ner-model.pt
|
||||||
|
data/pos-model.pt
|
||||||
|
data/pos-model.7z
|
||||||
|
|
34
3-NLP_services/Dockerfile
Normal file
|
@ -0,0 +1,34 @@
|
||||||
|
FROM python:3.9-slim
|
||||||
|
|
||||||
|
# Maintainer info
|
||||||
|
LABEL maintainer="khaledihkh@gmail.com"
|
||||||
|
|
||||||
|
# Make working directories
|
||||||
|
RUN mkdir -p /service
|
||||||
|
WORKDIR /service
|
||||||
|
|
||||||
|
# Upgrade pip with no cache
|
||||||
|
RUN pip install --no-cache-dir -U pip
|
||||||
|
|
||||||
|
# Copy application requirements file to the created working directory
|
||||||
|
COPY requirements.txt .
|
||||||
|
|
||||||
|
# Install application dependencies from the requirements file
|
||||||
|
RUN pip install -r requirements.txt
|
||||||
|
|
||||||
|
# Copy every file in the source folder to the created working directory
|
||||||
|
COPY . .
|
||||||
|
RUN pip install ./src/piraye
|
||||||
|
RUN pip install transformers[sentencepiece]
|
||||||
|
|
||||||
|
|
||||||
|
ENV NER_MODEL_NAME ""
|
||||||
|
ENV NORMALIZE "False"
|
||||||
|
ENV SHORT_MODEL_NAME ""
|
||||||
|
ENV LONG_MODEL_NAME ""
|
||||||
|
ENV POS_MODEL_NAME ""
|
||||||
|
ENV BATCH_SIZE "4"
|
||||||
|
ENV DEVICE "gpu"
|
||||||
|
|
||||||
|
EXPOSE 80
|
||||||
|
CMD ["uvicorn", "main:app", "--host", "0.0.0.0", "--port", "80"]
|
18
3-NLP_services/README.md
Normal file
|
@ -0,0 +1,18 @@
|
||||||
|
# Services
|
||||||
|
## Requirements
|
||||||
|
````shell
|
||||||
|
pip install -r requirements.txt
|
||||||
|
````
|
||||||
|
## Download Models
|
||||||
|
download models and place in data folder
|
||||||
|
https://www.mediafire.com/folder/tz3t9c9rpf6fo/models
|
||||||
|
|
||||||
|
## Getting started
|
||||||
|
|
||||||
|
````shell
|
||||||
|
cd fastapi_flair
|
||||||
|
uvicorn main:app --reload
|
||||||
|
````
|
||||||
|
|
||||||
|
## Documentation
|
||||||
|
[Open Documentations](./docs/docs.md)
|
10
3-NLP_services/data/users.json
Normal file
|
@ -0,0 +1,10 @@
|
||||||
|
[
|
||||||
|
{
|
||||||
|
"client-id":"hassan",
|
||||||
|
"client-password":"1234"
|
||||||
|
},
|
||||||
|
{
|
||||||
|
"client-id":"ali",
|
||||||
|
"client-password":"12345"
|
||||||
|
}
|
||||||
|
]
|
8
3-NLP_services/docker-compose.yml
Normal file
|
@ -0,0 +1,8 @@
|
||||||
|
version: '3.0'
|
||||||
|
|
||||||
|
services:
|
||||||
|
web:
|
||||||
|
image: "services"
|
||||||
|
command: uvicorn main:app --host 0.0.0.0
|
||||||
|
ports:
|
||||||
|
- 8008:8000
|
10
3-NLP_services/docs/docs.md
Normal file
|
@ -0,0 +1,10 @@
|
||||||
|
### Libraries:
|
||||||
|
|
||||||
|
1. Uvicorn: Uvicorn is an ASGI (The Asynchronous Server Gateway Interface is a calling convention for web servers to forward requests to asynchronous-capable Python programming language frameworks, and applications. It is built as a successor to the Web Server Gateway Interface.) web server implementation for Python. Until recently Python has lacked a minimal low-level server/application interface for async frameworks. The ASGI specification fills this gap, and means we're now able to start building a common set of tooling usable across all async frameworks.
|
||||||
|
2. FastAPI: FastAPI framework, high performance, easy to learn, fast to code, ready for production
|
||||||
|
FastAPI is a modern, fast (high-performance), web framework for building APIs with Python 3.7+ based on standard Python type hints.
|
||||||
|
3. NLTK: NLTK is a leading platform for building Python programs to work with human language data. It provides easy-to-use interfaces to over 50 corpora and lexical resources such as WordNet, along with a suite of text processing libraries for classification, tokenization, stemming, tagging, parsing, and semantic reasoning, wrappers for industrial-strength NLP libraries, and an active discussion forum.
|
||||||
|
4. Piraye: NLP Utils, A utility for normalizing persian, arabic and english texts
|
||||||
|
5. ...
|
||||||
|
|
||||||
|
|
199
3-NLP_services/main.py
Normal file
|
@ -0,0 +1,199 @@
|
||||||
|
from __future__ import annotations
|
||||||
|
import uvicorn
|
||||||
|
import torch
|
||||||
|
from fastapi import FastAPI, HTTPException, Request
|
||||||
|
from starlette.status import HTTP_201_CREATED
|
||||||
|
|
||||||
|
from src.database_handler import Database
|
||||||
|
from src.requests_data import Requests
|
||||||
|
from model.request_models import InputNerType, InputSummaryType, InputNREType
|
||||||
|
from model.response_models import BaseResponse, ResponseNerModel, ResponseSummaryModel, ResponseOIEModel, \
|
||||||
|
ResponseNREModel
|
||||||
|
from src.response_ner import NerResponse, ResponsesNer
|
||||||
|
from fastapi import BackgroundTasks
|
||||||
|
|
||||||
|
from src.response_nre import NREResponse, ResponsesNRE
|
||||||
|
from src.response_oie import OIEResponse, ResponsesOIE
|
||||||
|
from src.response_summary import ShortResponse, LongResponse, ResponsesSummary, NormalizerTexts
|
||||||
|
|
||||||
|
print("torch version : ", torch.__version__)
|
||||||
|
app = FastAPI()
|
||||||
|
# ner_model_name = os.getenv("NER_MODEL_NAME")
|
||||||
|
# normalize = os.getenv("NORMALIZE")
|
||||||
|
# short_model_name = os.getenv("SHORT_MODEL_NAME")
|
||||||
|
# long_model_name = os.getenv("LONG_MODEL_NAME")
|
||||||
|
# pos_model_name = os.getenv("POS_MODEL_NAME")
|
||||||
|
# batch_size = int(os.getenv("BATCH_SIZE"))
|
||||||
|
# device = os.getenv("DEVICE")
|
||||||
|
#
|
||||||
|
# print(ner_model_name, normalize, short_model_name, long_model_name, pos_model_name, batch_size, device)
|
||||||
|
|
||||||
|
# initial docker parameters
|
||||||
|
pos_model_name = "./data/pos-model.pt"
|
||||||
|
ner_model_name = "./data/ner-model.pt"
|
||||||
|
short_model_name = "csebuetnlp/mT5_multilingual_XLSum"
|
||||||
|
long_model_name = "alireza7/ARMAN-MSR-persian-base-PN-summary"
|
||||||
|
# oie_model_name = "default"
|
||||||
|
oie_model_name = ""
|
||||||
|
ner_model_name = "default"
|
||||||
|
pos_model_name = "default"
|
||||||
|
nre_model_name = ""
|
||||||
|
|
||||||
|
bert_oie = './data/mbert-base-parsinlu-entailment'
|
||||||
|
short_model_name = ""
|
||||||
|
long_model_name = ""
|
||||||
|
normalize = "False"
|
||||||
|
batch_size = 4
|
||||||
|
device = "cpu"
|
||||||
|
|
||||||
|
if ner_model_name == "":
|
||||||
|
ner_response = None
|
||||||
|
else:
|
||||||
|
if ner_model_name.lower() == "default":
|
||||||
|
ner_model_name = "./data/ner-model.pt"
|
||||||
|
ner_response = NerResponse(ner_model_name, batch_size=batch_size, device=device)
|
||||||
|
|
||||||
|
if oie_model_name == "":
|
||||||
|
oie_response = None
|
||||||
|
else:
|
||||||
|
if oie_model_name.lower() == "default":
|
||||||
|
oie_model_name = "./data/mbertEntail-2GBEn.bin"
|
||||||
|
oie_response = OIEResponse(oie_model_name, BERT=bert_oie, batch_size=batch_size, device=device)
|
||||||
|
|
||||||
|
if nre_model_name == "":
|
||||||
|
nre_response = None
|
||||||
|
else:
|
||||||
|
if nre_model_name.lower() == "default":
|
||||||
|
nre_model_name = "./data/model.ckpt.pth.tar"
|
||||||
|
nre_response = NREResponse(nre_model_name)
|
||||||
|
|
||||||
|
if normalize.lower() == "false" or pos_model_name == "":
|
||||||
|
short_response = ShortResponse(normalizer_input=None, device=device)
|
||||||
|
long_response = LongResponse(normalizer_input=None, device=device)
|
||||||
|
else:
|
||||||
|
if pos_model_name.lower() == "default":
|
||||||
|
pos_model_name = "./data/pos-model.pt"
|
||||||
|
normalizer_pos = NormalizerTexts(pos_model_name)
|
||||||
|
short_response = ShortResponse(normalizer_input=normalizer_pos, device=device)
|
||||||
|
long_response = LongResponse(normalizer_input=normalizer_pos, device=device)
|
||||||
|
if short_model_name != "":
|
||||||
|
short_response.load_model(short_model_name)
|
||||||
|
if long_model_name != "":
|
||||||
|
long_response.load_model(long_model_name)
|
||||||
|
|
||||||
|
requests: Requests = Requests()
|
||||||
|
database: Database = Database()
|
||||||
|
|
||||||
|
|
||||||
|
@app.get("/")
|
||||||
|
async def root():
|
||||||
|
return {"message": "Hello World"}
|
||||||
|
|
||||||
|
|
||||||
|
@app.get("/ner/{item_id}", response_model=ResponseNerModel)
|
||||||
|
async def read_item(item_id, request: Request) -> ResponseNerModel:
|
||||||
|
if database.check_client(client_id=request.headers.get('Client-Id'),
|
||||||
|
client_password=request.headers.get('Client-Password')) and ner_response:
|
||||||
|
if not ResponsesNer.check_id(int(item_id)):
|
||||||
|
raise HTTPException(status_code=404, detail="Item not found")
|
||||||
|
return ResponsesNer.get_response(int(item_id))
|
||||||
|
else:
|
||||||
|
raise HTTPException(status_code=403, detail="you are not allowed")
|
||||||
|
|
||||||
|
|
||||||
|
@app.get("/oie/{item_id}", response_model=ResponseOIEModel)
|
||||||
|
async def read_item(item_id, request: Request) -> ResponseOIEModel:
|
||||||
|
if database.check_client(client_id=request.headers.get('Client-Id'),
|
||||||
|
client_password=request.headers.get('Client-Password')) and oie_response:
|
||||||
|
if not ResponsesOIE.check_id(int(item_id)):
|
||||||
|
raise HTTPException(status_code=404, detail="Item not found")
|
||||||
|
return ResponsesOIE.get_response(int(item_id))
|
||||||
|
else:
|
||||||
|
raise HTTPException(status_code=403, detail="you are not allowed")
|
||||||
|
|
||||||
|
|
||||||
|
@app.get("/nre/{item_id}", response_model=ResponseNREModel)
|
||||||
|
async def read_item(item_id, request: Request) -> ResponseNREModel:
|
||||||
|
if database.check_client(client_id=request.headers.get('Client-Id'),
|
||||||
|
client_password=request.headers.get('Client-Password')) and nre_response:
|
||||||
|
if not ResponsesNRE.check_id(int(item_id)):
|
||||||
|
raise HTTPException(status_code=404, detail="Item not found")
|
||||||
|
return ResponsesNRE.get_response(int(item_id))
|
||||||
|
else:
|
||||||
|
raise HTTPException(status_code=403, detail="you are not allowed")
|
||||||
|
|
||||||
|
|
||||||
|
@app.get("/summary/{item_id}", response_model=ResponseSummaryModel)
|
||||||
|
async def read_item(item_id, request: Request) -> ResponseSummaryModel:
|
||||||
|
if database.check_client(client_id=request.headers.get('Client-Id'),
|
||||||
|
client_password=request.headers.get('Client-Password')):
|
||||||
|
if not ResponsesSummary.check_id(int(item_id)):
|
||||||
|
raise HTTPException(status_code=404, detail="Item not found")
|
||||||
|
return ResponsesSummary.get_response(int(item_id))
|
||||||
|
else:
|
||||||
|
raise HTTPException(status_code=403, detail="you are not allowed")
|
||||||
|
|
||||||
|
|
||||||
|
@app.post("/ner", response_model=BaseResponse, status_code=HTTP_201_CREATED)
|
||||||
|
async def ner(input_json: InputNerType, background_tasks: BackgroundTasks, request: Request) -> BaseResponse:
|
||||||
|
if database.check_client(client_id=request.headers.get('Client-Id'),
|
||||||
|
client_password=request.headers.get('Client-Password')) and ner_model_name != "":
|
||||||
|
request_id = requests.add_request_ner(input_json)
|
||||||
|
background_tasks.add_task(ner_response.predict_json, input_json, request_id)
|
||||||
|
return BaseResponse(status="input received successfully", id=request_id)
|
||||||
|
else:
|
||||||
|
raise HTTPException(status_code=403, detail="you are not allowed")
|
||||||
|
# return ner_response.predict_json(input_json)
|
||||||
|
|
||||||
|
|
||||||
|
@app.post("/oie", response_model=BaseResponse, status_code=HTTP_201_CREATED)
|
||||||
|
async def oie(input_json: InputNerType, background_tasks: BackgroundTasks, request: Request) -> BaseResponse:
|
||||||
|
if database.check_client(client_id=request.headers.get('Client-Id'),
|
||||||
|
client_password=request.headers.get('Client-Password')) and oie_model_name != "":
|
||||||
|
request_id = requests.add_request_oie(input_json)
|
||||||
|
background_tasks.add_task(oie_response.predict_json, input_json, request_id)
|
||||||
|
return BaseResponse(status="input received successfully", id=request_id)
|
||||||
|
else:
|
||||||
|
raise HTTPException(status_code=403, detail="you are not allowed")
|
||||||
|
# return ner_response.predict_json(input_json)
|
||||||
|
|
||||||
|
|
||||||
|
@app.post("/nre", response_model=BaseResponse, status_code=HTTP_201_CREATED)
|
||||||
|
async def oie(input_json: InputNREType, background_tasks: BackgroundTasks, request: Request) -> BaseResponse:
|
||||||
|
if database.check_client(client_id=request.headers.get('Client-Id'),
|
||||||
|
client_password=request.headers.get('Client-Password')) and nre_model_name != "":
|
||||||
|
request_id = requests.add_request_nre(input_json)
|
||||||
|
background_tasks.add_task(nre_response.predict_json, input_json, request_id)
|
||||||
|
return BaseResponse(status="input received successfully", id=request_id)
|
||||||
|
else:
|
||||||
|
raise HTTPException(status_code=403, detail="you are not allowed")
|
||||||
|
# return ner_response.predict_json(input_json)
|
||||||
|
|
||||||
|
|
||||||
|
@app.post("/summary/short", response_model=BaseResponse, status_code=HTTP_201_CREATED)
|
||||||
|
async def short_summary(input_json: InputSummaryType, background_tasks: BackgroundTasks,
|
||||||
|
request: Request) -> BaseResponse:
|
||||||
|
if database.check_client(client_id=request.headers.get('Client-Id'),
|
||||||
|
client_password=request.headers.get('Client-Password')) and short_model_name != "":
|
||||||
|
request_id = requests.add_request_summary(input_json)
|
||||||
|
background_tasks.add_task(short_response.get_result, input_json, request_id)
|
||||||
|
return BaseResponse(status="input received successfully", id=request_id)
|
||||||
|
else:
|
||||||
|
raise HTTPException(status_code=403, detail="you are not allowed")
|
||||||
|
# return ner_response.predict_json(input_json)
|
||||||
|
|
||||||
|
|
||||||
|
@app.post("/summary/long", response_model=BaseResponse, status_code=HTTP_201_CREATED)
|
||||||
|
async def long_summary(input_json: InputSummaryType, background_tasks: BackgroundTasks,
|
||||||
|
request: Request) -> BaseResponse:
|
||||||
|
if database.check_client(client_id=request.headers.get('Client-Id'),
|
||||||
|
client_password=request.headers.get('Client-Password')) and long_model_name != "":
|
||||||
|
request_id = requests.add_request_summary(input_json)
|
||||||
|
background_tasks.add_task(long_response.get_result, input_json, request_id)
|
||||||
|
return BaseResponse(status="input received successfully", id=request_id)
|
||||||
|
else:
|
||||||
|
raise HTTPException(status_code=403, detail="you are not allowed")
|
||||||
|
|
||||||
|
|
||||||
|
if __name__ == "__main__":
|
||||||
|
uvicorn.run(app, host="0.0.0.0", port=8000)
|
0
3-NLP_services/model/__init__.py
Normal file
28
3-NLP_services/model/request_models.py
Normal file
|
@ -0,0 +1,28 @@
|
||||||
|
from typing import List, Dict, Tuple
|
||||||
|
from pydantic import BaseModel
|
||||||
|
|
||||||
|
|
||||||
|
class InputElement(BaseModel):
|
||||||
|
lang: str
|
||||||
|
text: str
|
||||||
|
|
||||||
|
|
||||||
|
class NREHType(BaseModel):
|
||||||
|
pos: str
|
||||||
|
|
||||||
|
|
||||||
|
class NRETType(BaseModel):
|
||||||
|
pos: str
|
||||||
|
|
||||||
|
|
||||||
|
class InputElementNRE(BaseModel):
|
||||||
|
text: str
|
||||||
|
h: NREHType
|
||||||
|
t: NRETType
|
||||||
|
|
||||||
|
|
||||||
|
InputNerType = List[InputElement]
|
||||||
|
InputOIEType = List[InputElement]
|
||||||
|
InputNREType = List[InputElementNRE]
|
||||||
|
|
||||||
|
InputSummaryType = List[str]
|
43
3-NLP_services/model/response_models.py
Normal file
|
@ -0,0 +1,43 @@
|
||||||
|
from typing import List, Optional, Tuple
|
||||||
|
|
||||||
|
from pydantic import BaseModel
|
||||||
|
|
||||||
|
|
||||||
|
class EntityNerResponseModel(BaseModel):
|
||||||
|
entity_group: str
|
||||||
|
word: str
|
||||||
|
start: int
|
||||||
|
end: int
|
||||||
|
score: float
|
||||||
|
|
||||||
|
|
||||||
|
class EntityOIEResponseModel(BaseModel):
|
||||||
|
score: str
|
||||||
|
relation: str
|
||||||
|
arg1: str
|
||||||
|
arg2: str
|
||||||
|
|
||||||
|
|
||||||
|
class BaseResponse(BaseModel):
|
||||||
|
id: int
|
||||||
|
status: str
|
||||||
|
|
||||||
|
|
||||||
|
class ResponseNerModel(BaseModel):
|
||||||
|
progression: str
|
||||||
|
result: Optional[List[List[EntityNerResponseModel]]]
|
||||||
|
|
||||||
|
|
||||||
|
class ResponseSummaryModel(BaseModel):
|
||||||
|
progression: str
|
||||||
|
result: Optional[List[str]]
|
||||||
|
|
||||||
|
|
||||||
|
class ResponseOIEModel(BaseModel):
|
||||||
|
progression: str
|
||||||
|
result: Optional[List[EntityOIEResponseModel]]
|
||||||
|
|
||||||
|
|
||||||
|
class ResponseNREModel(BaseModel):
|
||||||
|
progression: str
|
||||||
|
result: Optional[List[Tuple[str, float]]]
|
672
3-NLP_services/openapi.json
Normal file
|
@ -0,0 +1,672 @@
|
||||||
|
{
|
||||||
|
"openapi": "3.0.2",
|
||||||
|
"info": {
|
||||||
|
"title": "FastAPI",
|
||||||
|
"version": "0.1.0"
|
||||||
|
},
|
||||||
|
"paths": {
|
||||||
|
"/": {
|
||||||
|
"get": {
|
||||||
|
"summary": "Root",
|
||||||
|
"operationId": "root__get",
|
||||||
|
"responses": {
|
||||||
|
"200": {
|
||||||
|
"description": "Successful Response",
|
||||||
|
"content": {
|
||||||
|
"application/json": {
|
||||||
|
"schema": {}
|
||||||
|
}
|
||||||
|
}
|
||||||
|
}
|
||||||
|
}
|
||||||
|
}
|
||||||
|
},
|
||||||
|
"/ner/{item_id}": {
|
||||||
|
"get": {
|
||||||
|
"summary": "Read Item",
|
||||||
|
"operationId": "read_item_ner__item_id__get",
|
||||||
|
"parameters": [
|
||||||
|
{
|
||||||
|
"required": true,
|
||||||
|
"schema": {
|
||||||
|
"title": "Item Id"
|
||||||
|
},
|
||||||
|
"name": "item_id",
|
||||||
|
"in": "path"
|
||||||
|
}
|
||||||
|
],
|
||||||
|
"responses": {
|
||||||
|
"200": {
|
||||||
|
"description": "Successful Response",
|
||||||
|
"content": {
|
||||||
|
"application/json": {
|
||||||
|
"schema": {
|
||||||
|
"$ref": "#/components/schemas/ResponseNerModel"
|
||||||
|
}
|
||||||
|
}
|
||||||
|
}
|
||||||
|
},
|
||||||
|
"422": {
|
||||||
|
"description": "Validation Error",
|
||||||
|
"content": {
|
||||||
|
"application/json": {
|
||||||
|
"schema": {
|
||||||
|
"$ref": "#/components/schemas/HTTPValidationError"
|
||||||
|
}
|
||||||
|
}
|
||||||
|
}
|
||||||
|
}
|
||||||
|
}
|
||||||
|
}
|
||||||
|
},
|
||||||
|
"/oie/{item_id}": {
|
||||||
|
"get": {
|
||||||
|
"summary": "Read Item",
|
||||||
|
"operationId": "read_item_oie__item_id__get",
|
||||||
|
"parameters": [
|
||||||
|
{
|
||||||
|
"required": true,
|
||||||
|
"schema": {
|
||||||
|
"title": "Item Id"
|
||||||
|
},
|
||||||
|
"name": "item_id",
|
||||||
|
"in": "path"
|
||||||
|
}
|
||||||
|
],
|
||||||
|
"responses": {
|
||||||
|
"200": {
|
||||||
|
"description": "Successful Response",
|
||||||
|
"content": {
|
||||||
|
"application/json": {
|
||||||
|
"schema": {
|
||||||
|
"$ref": "#/components/schemas/ResponseOIEModel"
|
||||||
|
}
|
||||||
|
}
|
||||||
|
}
|
||||||
|
},
|
||||||
|
"422": {
|
||||||
|
"description": "Validation Error",
|
||||||
|
"content": {
|
||||||
|
"application/json": {
|
||||||
|
"schema": {
|
||||||
|
"$ref": "#/components/schemas/HTTPValidationError"
|
||||||
|
}
|
||||||
|
}
|
||||||
|
}
|
||||||
|
}
|
||||||
|
}
|
||||||
|
}
|
||||||
|
},
|
||||||
|
"/nre/{item_id}": {
|
||||||
|
"get": {
|
||||||
|
"summary": "Read Item",
|
||||||
|
"operationId": "read_item_nre__item_id__get",
|
||||||
|
"parameters": [
|
||||||
|
{
|
||||||
|
"required": true,
|
||||||
|
"schema": {
|
||||||
|
"title": "Item Id"
|
||||||
|
},
|
||||||
|
"name": "item_id",
|
||||||
|
"in": "path"
|
||||||
|
}
|
||||||
|
],
|
||||||
|
"responses": {
|
||||||
|
"200": {
|
||||||
|
"description": "Successful Response",
|
||||||
|
"content": {
|
||||||
|
"application/json": {
|
||||||
|
"schema": {
|
||||||
|
"$ref": "#/components/schemas/ResponseNREModel"
|
||||||
|
}
|
||||||
|
}
|
||||||
|
}
|
||||||
|
},
|
||||||
|
"422": {
|
||||||
|
"description": "Validation Error",
|
||||||
|
"content": {
|
||||||
|
"application/json": {
|
||||||
|
"schema": {
|
||||||
|
"$ref": "#/components/schemas/HTTPValidationError"
|
||||||
|
}
|
||||||
|
}
|
||||||
|
}
|
||||||
|
}
|
||||||
|
}
|
||||||
|
}
|
||||||
|
},
|
||||||
|
"/summary/{item_id}": {
|
||||||
|
"get": {
|
||||||
|
"summary": "Read Item",
|
||||||
|
"operationId": "read_item_summary__item_id__get",
|
||||||
|
"parameters": [
|
||||||
|
{
|
||||||
|
"required": true,
|
||||||
|
"schema": {
|
||||||
|
"title": "Item Id"
|
||||||
|
},
|
||||||
|
"name": "item_id",
|
||||||
|
"in": "path"
|
||||||
|
}
|
||||||
|
],
|
||||||
|
"responses": {
|
||||||
|
"200": {
|
||||||
|
"description": "Successful Response",
|
||||||
|
"content": {
|
||||||
|
"application/json": {
|
||||||
|
"schema": {
|
||||||
|
"$ref": "#/components/schemas/ResponseSummaryModel"
|
||||||
|
}
|
||||||
|
}
|
||||||
|
}
|
||||||
|
},
|
||||||
|
"422": {
|
||||||
|
"description": "Validation Error",
|
||||||
|
"content": {
|
||||||
|
"application/json": {
|
||||||
|
"schema": {
|
||||||
|
"$ref": "#/components/schemas/HTTPValidationError"
|
||||||
|
}
|
||||||
|
}
|
||||||
|
}
|
||||||
|
}
|
||||||
|
}
|
||||||
|
}
|
||||||
|
},
|
||||||
|
"/ner": {
|
||||||
|
"post": {
|
||||||
|
"summary": "Ner",
|
||||||
|
"operationId": "ner_ner_post",
|
||||||
|
"requestBody": {
|
||||||
|
"content": {
|
||||||
|
"application/json": {
|
||||||
|
"schema": {
|
||||||
|
"title": "Input Json",
|
||||||
|
"type": "array",
|
||||||
|
"items": {
|
||||||
|
"$ref": "#/components/schemas/InputElement"
|
||||||
|
}
|
||||||
|
}
|
||||||
|
}
|
||||||
|
},
|
||||||
|
"required": true
|
||||||
|
},
|
||||||
|
"responses": {
|
||||||
|
"201": {
|
||||||
|
"description": "Successful Response",
|
||||||
|
"content": {
|
||||||
|
"application/json": {
|
||||||
|
"schema": {
|
||||||
|
"$ref": "#/components/schemas/BaseResponse"
|
||||||
|
}
|
||||||
|
}
|
||||||
|
}
|
||||||
|
},
|
||||||
|
"422": {
|
||||||
|
"description": "Validation Error",
|
||||||
|
"content": {
|
||||||
|
"application/json": {
|
||||||
|
"schema": {
|
||||||
|
"$ref": "#/components/schemas/HTTPValidationError"
|
||||||
|
}
|
||||||
|
}
|
||||||
|
}
|
||||||
|
}
|
||||||
|
}
|
||||||
|
}
|
||||||
|
},
|
||||||
|
"/oie": {
|
||||||
|
"post": {
|
||||||
|
"summary": "Oie",
|
||||||
|
"operationId": "oie_oie_post",
|
||||||
|
"requestBody": {
|
||||||
|
"content": {
|
||||||
|
"application/json": {
|
||||||
|
"schema": {
|
||||||
|
"title": "Input Json",
|
||||||
|
"type": "array",
|
||||||
|
"items": {
|
||||||
|
"$ref": "#/components/schemas/InputElement"
|
||||||
|
}
|
||||||
|
}
|
||||||
|
}
|
||||||
|
},
|
||||||
|
"required": true
|
||||||
|
},
|
||||||
|
"responses": {
|
||||||
|
"201": {
|
||||||
|
"description": "Successful Response",
|
||||||
|
"content": {
|
||||||
|
"application/json": {
|
||||||
|
"schema": {
|
||||||
|
"$ref": "#/components/schemas/BaseResponse"
|
||||||
|
}
|
||||||
|
}
|
||||||
|
}
|
||||||
|
},
|
||||||
|
"422": {
|
||||||
|
"description": "Validation Error",
|
||||||
|
"content": {
|
||||||
|
"application/json": {
|
||||||
|
"schema": {
|
||||||
|
"$ref": "#/components/schemas/HTTPValidationError"
|
||||||
|
}
|
||||||
|
}
|
||||||
|
}
|
||||||
|
}
|
||||||
|
}
|
||||||
|
}
|
||||||
|
},
|
||||||
|
"/nre": {
|
||||||
|
"post": {
|
||||||
|
"summary": "Oie",
|
||||||
|
"operationId": "oie_nre_post",
|
||||||
|
"requestBody": {
|
||||||
|
"content": {
|
||||||
|
"application/json": {
|
||||||
|
"schema": {
|
||||||
|
"title": "Input Json",
|
||||||
|
"type": "array",
|
||||||
|
"items": {
|
||||||
|
"$ref": "#/components/schemas/InputElementNRE"
|
||||||
|
}
|
||||||
|
}
|
||||||
|
}
|
||||||
|
},
|
||||||
|
"required": true
|
||||||
|
},
|
||||||
|
"responses": {
|
||||||
|
"201": {
|
||||||
|
"description": "Successful Response",
|
||||||
|
"content": {
|
||||||
|
"application/json": {
|
||||||
|
"schema": {
|
||||||
|
"$ref": "#/components/schemas/BaseResponse"
|
||||||
|
}
|
||||||
|
}
|
||||||
|
}
|
||||||
|
},
|
||||||
|
"422": {
|
||||||
|
"description": "Validation Error",
|
||||||
|
"content": {
|
||||||
|
"application/json": {
|
||||||
|
"schema": {
|
||||||
|
"$ref": "#/components/schemas/HTTPValidationError"
|
||||||
|
}
|
||||||
|
}
|
||||||
|
}
|
||||||
|
}
|
||||||
|
}
|
||||||
|
}
|
||||||
|
},
|
||||||
|
"/summary/short": {
|
||||||
|
"post": {
|
||||||
|
"summary": "Short Summary",
|
||||||
|
"operationId": "short_summary_summary_short_post",
|
||||||
|
"requestBody": {
|
||||||
|
"content": {
|
||||||
|
"application/json": {
|
||||||
|
"schema": {
|
||||||
|
"title": "Input Json",
|
||||||
|
"type": "array",
|
||||||
|
"items": {
|
||||||
|
"type": "string"
|
||||||
|
}
|
||||||
|
}
|
||||||
|
}
|
||||||
|
},
|
||||||
|
"required": true
|
||||||
|
},
|
||||||
|
"responses": {
|
||||||
|
"201": {
|
||||||
|
"description": "Successful Response",
|
||||||
|
"content": {
|
||||||
|
"application/json": {
|
||||||
|
"schema": {
|
||||||
|
"$ref": "#/components/schemas/BaseResponse"
|
||||||
|
}
|
||||||
|
}
|
||||||
|
}
|
||||||
|
},
|
||||||
|
"422": {
|
||||||
|
"description": "Validation Error",
|
||||||
|
"content": {
|
||||||
|
"application/json": {
|
||||||
|
"schema": {
|
||||||
|
"$ref": "#/components/schemas/HTTPValidationError"
|
||||||
|
}
|
||||||
|
}
|
||||||
|
}
|
||||||
|
}
|
||||||
|
}
|
||||||
|
}
|
||||||
|
},
|
||||||
|
"/summary/long": {
|
||||||
|
"post": {
|
||||||
|
"summary": "Long Summary",
|
||||||
|
"operationId": "long_summary_summary_long_post",
|
||||||
|
"requestBody": {
|
||||||
|
"content": {
|
||||||
|
"application/json": {
|
||||||
|
"schema": {
|
||||||
|
"title": "Input Json",
|
||||||
|
"type": "array",
|
||||||
|
"items": {
|
||||||
|
"type": "string"
|
||||||
|
}
|
||||||
|
}
|
||||||
|
}
|
||||||
|
},
|
||||||
|
"required": true
|
||||||
|
},
|
||||||
|
"responses": {
|
||||||
|
"201": {
|
||||||
|
"description": "Successful Response",
|
||||||
|
"content": {
|
||||||
|
"application/json": {
|
||||||
|
"schema": {
|
||||||
|
"$ref": "#/components/schemas/BaseResponse"
|
||||||
|
}
|
||||||
|
}
|
||||||
|
}
|
||||||
|
},
|
||||||
|
"422": {
|
||||||
|
"description": "Validation Error",
|
||||||
|
"content": {
|
||||||
|
"application/json": {
|
||||||
|
"schema": {
|
||||||
|
"$ref": "#/components/schemas/HTTPValidationError"
|
||||||
|
}
|
||||||
|
}
|
||||||
|
}
|
||||||
|
}
|
||||||
|
}
|
||||||
|
}
|
||||||
|
}
|
||||||
|
},
|
||||||
|
"components": {
|
||||||
|
"schemas": {
|
||||||
|
"BaseResponse": {
|
||||||
|
"title": "BaseResponse",
|
||||||
|
"required": [
|
||||||
|
"id",
|
||||||
|
"status"
|
||||||
|
],
|
||||||
|
"type": "object",
|
||||||
|
"properties": {
|
||||||
|
"id": {
|
||||||
|
"title": "Id",
|
||||||
|
"type": "integer"
|
||||||
|
},
|
||||||
|
"status": {
|
||||||
|
"title": "Status",
|
||||||
|
"type": "string"
|
||||||
|
}
|
||||||
|
}
|
||||||
|
},
|
||||||
|
"EntityNerResponseModel": {
|
||||||
|
"title": "EntityNerResponseModel",
|
||||||
|
"required": [
|
||||||
|
"entity_group",
|
||||||
|
"word",
|
||||||
|
"start",
|
||||||
|
"end",
|
||||||
|
"score"
|
||||||
|
],
|
||||||
|
"type": "object",
|
||||||
|
"properties": {
|
||||||
|
"entity_group": {
|
||||||
|
"title": "Entity Group",
|
||||||
|
"type": "string"
|
||||||
|
},
|
||||||
|
"word": {
|
||||||
|
"title": "Word",
|
||||||
|
"type": "string"
|
||||||
|
},
|
||||||
|
"start": {
|
||||||
|
"title": "Start",
|
||||||
|
"type": "integer"
|
||||||
|
},
|
||||||
|
"end": {
|
||||||
|
"title": "End",
|
||||||
|
"type": "integer"
|
||||||
|
},
|
||||||
|
"score": {
|
||||||
|
"title": "Score",
|
||||||
|
"type": "number"
|
||||||
|
}
|
||||||
|
}
|
||||||
|
},
|
||||||
|
"EntityOIEResponseModel": {
|
||||||
|
"title": "EntityOIEResponseModel",
|
||||||
|
"required": [
|
||||||
|
"score",
|
||||||
|
"relation",
|
||||||
|
"arg1",
|
||||||
|
"arg2"
|
||||||
|
],
|
||||||
|
"type": "object",
|
||||||
|
"properties": {
|
||||||
|
"score": {
|
||||||
|
"title": "Score",
|
||||||
|
"type": "string"
|
||||||
|
},
|
||||||
|
"relation": {
|
||||||
|
"title": "Relation",
|
||||||
|
"type": "string"
|
||||||
|
},
|
||||||
|
"arg1": {
|
||||||
|
"title": "Arg1",
|
||||||
|
"type": "string"
|
||||||
|
},
|
||||||
|
"arg2": {
|
||||||
|
"title": "Arg2",
|
||||||
|
"type": "string"
|
||||||
|
}
|
||||||
|
}
|
||||||
|
},
|
||||||
|
"HTTPValidationError": {
|
||||||
|
"title": "HTTPValidationError",
|
||||||
|
"type": "object",
|
||||||
|
"properties": {
|
||||||
|
"detail": {
|
||||||
|
"title": "Detail",
|
||||||
|
"type": "array",
|
||||||
|
"items": {
|
||||||
|
"$ref": "#/components/schemas/ValidationError"
|
||||||
|
}
|
||||||
|
}
|
||||||
|
}
|
||||||
|
},
|
||||||
|
"InputElement": {
|
||||||
|
"title": "InputElement",
|
||||||
|
"required": [
|
||||||
|
"lang",
|
||||||
|
"text"
|
||||||
|
],
|
||||||
|
"type": "object",
|
||||||
|
"properties": {
|
||||||
|
"lang": {
|
||||||
|
"title": "Lang",
|
||||||
|
"type": "string"
|
||||||
|
},
|
||||||
|
"text": {
|
||||||
|
"title": "Text",
|
||||||
|
"type": "string"
|
||||||
|
}
|
||||||
|
}
|
||||||
|
},
|
||||||
|
"InputElementNRE": {
|
||||||
|
"title": "InputElementNRE",
|
||||||
|
"required": [
|
||||||
|
"text",
|
||||||
|
"h",
|
||||||
|
"t"
|
||||||
|
],
|
||||||
|
"type": "object",
|
||||||
|
"properties": {
|
||||||
|
"text": {
|
||||||
|
"title": "Text",
|
||||||
|
"type": "string"
|
||||||
|
},
|
||||||
|
"h": {
|
||||||
|
"$ref": "#/components/schemas/NREHType"
|
||||||
|
},
|
||||||
|
"t": {
|
||||||
|
"$ref": "#/components/schemas/NRETType"
|
||||||
|
}
|
||||||
|
}
|
||||||
|
},
|
||||||
|
"NREHType": {
|
||||||
|
"title": "NREHType",
|
||||||
|
"required": [
|
||||||
|
"pos"
|
||||||
|
],
|
||||||
|
"type": "object",
|
||||||
|
"properties": {
|
||||||
|
"pos": {
|
||||||
|
"title": "Pos",
|
||||||
|
"type": "string"
|
||||||
|
}
|
||||||
|
}
|
||||||
|
},
|
||||||
|
"NRETType": {
|
||||||
|
"title": "NRETType",
|
||||||
|
"required": [
|
||||||
|
"pos"
|
||||||
|
],
|
||||||
|
"type": "object",
|
||||||
|
"properties": {
|
||||||
|
"pos": {
|
||||||
|
"title": "Pos",
|
||||||
|
"type": "string"
|
||||||
|
}
|
||||||
|
}
|
||||||
|
},
|
||||||
|
"ResponseNREModel": {
|
||||||
|
"title": "ResponseNREModel",
|
||||||
|
"required": [
|
||||||
|
"progression"
|
||||||
|
],
|
||||||
|
"type": "object",
|
||||||
|
"properties": {
|
||||||
|
"progression": {
|
||||||
|
"title": "Progression",
|
||||||
|
"type": "string"
|
||||||
|
},
|
||||||
|
"result": {
|
||||||
|
"title": "Result",
|
||||||
|
"type": "array",
|
||||||
|
"items": {
|
||||||
|
"type": "array",
|
||||||
|
"items": [
|
||||||
|
{
|
||||||
|
"type": "string"
|
||||||
|
},
|
||||||
|
{
|
||||||
|
"type": "number"
|
||||||
|
}
|
||||||
|
]
|
||||||
|
}
|
||||||
|
}
|
||||||
|
}
|
||||||
|
},
|
||||||
|
"ResponseNerModel": {
|
||||||
|
"title": "ResponseNerModel",
|
||||||
|
"required": [
|
||||||
|
"progression"
|
||||||
|
],
|
||||||
|
"type": "object",
|
||||||
|
"properties": {
|
||||||
|
"progression": {
|
||||||
|
"title": "Progression",
|
||||||
|
"type": "string"
|
||||||
|
},
|
||||||
|
"result": {
|
||||||
|
"title": "Result",
|
||||||
|
"type": "array",
|
||||||
|
"items": {
|
||||||
|
"type": "array",
|
||||||
|
"items": {
|
||||||
|
"$ref": "#/components/schemas/EntityNerResponseModel"
|
||||||
|
}
|
||||||
|
}
|
||||||
|
}
|
||||||
|
}
|
||||||
|
},
|
||||||
|
"ResponseOIEModel": {
|
||||||
|
"title": "ResponseOIEModel",
|
||||||
|
"required": [
|
||||||
|
"progression"
|
||||||
|
],
|
||||||
|
"type": "object",
|
||||||
|
"properties": {
|
||||||
|
"progression": {
|
||||||
|
"title": "Progression",
|
||||||
|
"type": "string"
|
||||||
|
},
|
||||||
|
"result": {
|
||||||
|
"title": "Result",
|
||||||
|
"type": "array",
|
||||||
|
"items": {
|
||||||
|
"$ref": "#/components/schemas/EntityOIEResponseModel"
|
||||||
|
}
|
||||||
|
}
|
||||||
|
}
|
||||||
|
},
|
||||||
|
"ResponseSummaryModel": {
|
||||||
|
"title": "ResponseSummaryModel",
|
||||||
|
"required": [
|
||||||
|
"progression"
|
||||||
|
],
|
||||||
|
"type": "object",
|
||||||
|
"properties": {
|
||||||
|
"progression": {
|
||||||
|
"title": "Progression",
|
||||||
|
"type": "string"
|
||||||
|
},
|
||||||
|
"result": {
|
||||||
|
"title": "Result",
|
||||||
|
"type": "array",
|
||||||
|
"items": {
|
||||||
|
"type": "string"
|
||||||
|
}
|
||||||
|
}
|
||||||
|
}
|
||||||
|
},
|
||||||
|
"ValidationError": {
|
||||||
|
"title": "ValidationError",
|
||||||
|
"required": [
|
||||||
|
"loc",
|
||||||
|
"msg",
|
||||||
|
"type"
|
||||||
|
],
|
||||||
|
"type": "object",
|
||||||
|
"properties": {
|
||||||
|
"loc": {
|
||||||
|
"title": "Location",
|
||||||
|
"type": "array",
|
||||||
|
"items": {
|
||||||
|
"anyOf": [
|
||||||
|
{
|
||||||
|
"type": "string"
|
||||||
|
},
|
||||||
|
{
|
||||||
|
"type": "integer"
|
||||||
|
}
|
||||||
|
]
|
||||||
|
}
|
||||||
|
},
|
||||||
|
"msg": {
|
||||||
|
"title": "Message",
|
||||||
|
"type": "string"
|
||||||
|
},
|
||||||
|
"type": {
|
||||||
|
"title": "Error Type",
|
||||||
|
"type": "string"
|
||||||
|
}
|
||||||
|
}
|
||||||
|
}
|
||||||
|
}
|
||||||
|
}
|
||||||
|
}
|
1
3-NLP_services/procfile
Normal file
|
@ -0,0 +1 @@
|
||||||
|
web : gunicorn -w 4 -k uvicorn.workers.UvicornWorker main:app
|
14
3-NLP_services/requirements.txt
Normal file
|
@ -0,0 +1,14 @@
|
||||||
|
requests==2.28.0
|
||||||
|
flair==0.10
|
||||||
|
langdetect==1.0.9
|
||||||
|
transformers
|
||||||
|
pydantic==1.8.2
|
||||||
|
uvicorn==0.17.6
|
||||||
|
torch>=1.12.1+cu116
|
||||||
|
fastapi==0.78.0
|
||||||
|
protobuf==3.20.0
|
||||||
|
sacremoses
|
||||||
|
nltk>=3.3
|
||||||
|
tqdm>=4.64.0
|
||||||
|
setuptools>=62.6.0
|
||||||
|
starlette>=0.19.1
|
21
3-NLP_services/src/Multi2OIE/LICENSE
Normal file
|
@ -0,0 +1,21 @@
|
||||||
|
MIT License
|
||||||
|
|
||||||
|
Copyright (c) 2020 Youngbin Ro
|
||||||
|
|
||||||
|
Permission is hereby granted, free of charge, to any person obtaining a copy
|
||||||
|
of this software and associated documentation files (the "Software"), to deal
|
||||||
|
in the Software without restriction, including without limitation the rights
|
||||||
|
to use, copy, modify, merge, publish, distribute, sublicense, and/or sell
|
||||||
|
copies of the Software, and to permit persons to whom the Software is
|
||||||
|
furnished to do so, subject to the following conditions:
|
||||||
|
|
||||||
|
The above copyright notice and this permission notice shall be included in all
|
||||||
|
copies or substantial portions of the Software.
|
||||||
|
|
||||||
|
THE SOFTWARE IS PROVIDED "AS IS", WITHOUT WARRANTY OF ANY KIND, EXPRESS OR
|
||||||
|
IMPLIED, INCLUDING BUT NOT LIMITED TO THE WARRANTIES OF MERCHANTABILITY,
|
||||||
|
FITNESS FOR A PARTICULAR PURPOSE AND NONINFRINGEMENT. IN NO EVENT SHALL THE
|
||||||
|
AUTHORS OR COPYRIGHT HOLDERS BE LIABLE FOR ANY CLAIM, DAMAGES OR OTHER
|
||||||
|
LIABILITY, WHETHER IN AN ACTION OF CONTRACT, TORT OR OTHERWISE, ARISING FROM,
|
||||||
|
OUT OF OR IN CONNECTION WITH THE SOFTWARE OR THE USE OR OTHER DEALINGS IN THE
|
||||||
|
SOFTWARE.
|
372
3-NLP_services/src/Multi2OIE/OIE.py
Normal file
|
@ -0,0 +1,372 @@
|
||||||
|
import torch
|
||||||
|
import torch
|
||||||
|
import torch.nn as nn
|
||||||
|
import torch.nn.functional as F
|
||||||
|
from transformers import BertModel
|
||||||
|
|
||||||
|
from torch.nn.modules.container import ModuleList
|
||||||
|
import copy
|
||||||
|
|
||||||
|
# from dataset import load_data
|
||||||
|
from transformers import BertTokenizer
|
||||||
|
|
||||||
|
from torch.utils.data import DataLoader
|
||||||
|
from torch.utils.data import Dataset
|
||||||
|
|
||||||
|
from tqdm import tqdm
|
||||||
|
import numpy as np
|
||||||
|
import src.Multi2OIE.utils.bio as bio
|
||||||
|
|
||||||
|
|
||||||
|
# from extract import extract
|
||||||
|
|
||||||
|
def _get_clones(module, n):
|
||||||
|
return ModuleList([copy.deepcopy(module) for _ in range(n)])
|
||||||
|
|
||||||
|
|
||||||
|
def _get_position_idxs(pred_mask, input_ids):
|
||||||
|
position_idxs = torch.zeros(pred_mask.shape, dtype=int, device=pred_mask.device)
|
||||||
|
for mask_idx, cur_mask in enumerate(pred_mask):
|
||||||
|
position_idxs[mask_idx, :] += 2
|
||||||
|
cur_nonzero = (cur_mask == 0).nonzero()
|
||||||
|
start = torch.min(cur_nonzero).item()
|
||||||
|
end = torch.max(cur_nonzero).item()
|
||||||
|
position_idxs[mask_idx, start:end + 1] = 1
|
||||||
|
pad_start = max(input_ids[mask_idx].nonzero()).item() + 1
|
||||||
|
position_idxs[mask_idx, pad_start:] = 0
|
||||||
|
return position_idxs
|
||||||
|
|
||||||
|
|
||||||
|
def _get_pred_feature(pred_hidden, pred_mask):
|
||||||
|
B, L, D = pred_hidden.shape
|
||||||
|
pred_features = torch.zeros((B, L, D), device=pred_mask.device)
|
||||||
|
for mask_idx, cur_mask in enumerate(pred_mask):
|
||||||
|
pred_position = (cur_mask == 0).nonzero().flatten()
|
||||||
|
pred_feature = torch.mean(pred_hidden[mask_idx, pred_position], dim=0)
|
||||||
|
pred_feature = torch.cat(L * [pred_feature.unsqueeze(0)])
|
||||||
|
pred_features[mask_idx, :, :] = pred_feature
|
||||||
|
return pred_features
|
||||||
|
|
||||||
|
|
||||||
|
def _get_activation_fn(activation):
|
||||||
|
if activation == "relu":
|
||||||
|
return F.relu
|
||||||
|
elif activation == "gelu":
|
||||||
|
return F.gelu
|
||||||
|
else:
|
||||||
|
raise RuntimeError("activation should be relu/gelu, not %s." % activation)
|
||||||
|
|
||||||
|
|
||||||
|
class ArgExtractorLayer(nn.Module):
|
||||||
|
def __init__(self,
|
||||||
|
d_model=768,
|
||||||
|
n_heads=8,
|
||||||
|
d_feedforward=2048,
|
||||||
|
dropout=0.1,
|
||||||
|
activation='relu'):
|
||||||
|
"""
|
||||||
|
A layer similar to Transformer decoder without decoder self-attention.
|
||||||
|
(only encoder-decoder multi-head attention followed by feed-forward layers)
|
||||||
|
|
||||||
|
:param d_model: model dimensionality (default=768 from BERT-base)
|
||||||
|
:param n_heads: number of heads in multi-head attention layer
|
||||||
|
:param d_feedforward: dimensionality of point-wise feed-forward layer
|
||||||
|
:param dropout: drop rate of all layers
|
||||||
|
:param activation: activation function after first feed-forward layer
|
||||||
|
"""
|
||||||
|
super(ArgExtractorLayer, self).__init__()
|
||||||
|
self.multihead_attn = nn.MultiheadAttention(d_model, n_heads, dropout=dropout)
|
||||||
|
self.linear1 = nn.Linear(d_model, d_feedforward)
|
||||||
|
self.dropout1 = nn.Dropout(dropout)
|
||||||
|
self.linear2 = nn.Linear(d_feedforward, d_model)
|
||||||
|
|
||||||
|
self.norm1 = nn.LayerNorm(d_model)
|
||||||
|
self.norm2 = nn.LayerNorm(d_model)
|
||||||
|
self.dropout2 = nn.Dropout(dropout)
|
||||||
|
self.dropout3 = nn.Dropout(dropout)
|
||||||
|
self.activation = _get_activation_fn(activation)
|
||||||
|
|
||||||
|
def forward(self, target, source, key_mask=None):
|
||||||
|
"""
|
||||||
|
Single Transformer Decoder layer without self-attention
|
||||||
|
|
||||||
|
:param target: a tensor which takes a role as a query
|
||||||
|
:param source: a tensor which takes a role as a key & value
|
||||||
|
:param key_mask: key mask tensor with the shape of (batch_size, sequence_length)
|
||||||
|
"""
|
||||||
|
# Multi-head attention layer (+ add & norm)
|
||||||
|
attended = self.multihead_attn(
|
||||||
|
target, source, source,
|
||||||
|
key_padding_mask=key_mask)[0]
|
||||||
|
skipped = target + self.dropout1(attended)
|
||||||
|
normed = self.norm1(skipped)
|
||||||
|
|
||||||
|
# Point-wise feed-forward layer (+ add & norm)
|
||||||
|
projected = self.linear2(self.dropout2(self.activation(self.linear1(normed))))
|
||||||
|
skipped = normed + self.dropout1(projected)
|
||||||
|
normed = self.norm2(skipped)
|
||||||
|
return normed
|
||||||
|
|
||||||
|
|
||||||
|
class ArgModule(nn.Module):
|
||||||
|
def __init__(self, arg_layer, n_layers):
|
||||||
|
"""
|
||||||
|
Module for extracting arguments based on given encoder output and predicates.
|
||||||
|
It uses ArgExtractorLayer as a base block and repeat the block N('n_layers') times
|
||||||
|
|
||||||
|
:param arg_layer: an instance of the ArgExtractorLayer() class (required)
|
||||||
|
:param n_layers: the number of sub-layers in the ArgModule (required).
|
||||||
|
"""
|
||||||
|
super(ArgModule, self).__init__()
|
||||||
|
self.layers = _get_clones(arg_layer, n_layers)
|
||||||
|
self.n_layers = n_layers
|
||||||
|
|
||||||
|
def forward(self, encoded, predicate, pred_mask=None):
|
||||||
|
"""
|
||||||
|
:param encoded: output from sentence encoder with the shape of (L, B, D),
|
||||||
|
where L is the sequence length, B is the batch size, D is the embedding dimension
|
||||||
|
:param predicate: output from predicate module with the shape of (L, B, D)
|
||||||
|
:param pred_mask: mask that prevents attention to tokens which are not predicates
|
||||||
|
with the shape of (B, L)
|
||||||
|
:return: tensor like Transformer Decoder Layer Output
|
||||||
|
"""
|
||||||
|
output = encoded
|
||||||
|
for layer_idx in range(self.n_layers):
|
||||||
|
output = self.layers[layer_idx](
|
||||||
|
target=output, source=predicate, key_mask=pred_mask)
|
||||||
|
return output
|
||||||
|
|
||||||
|
|
||||||
|
class Multi2OIE(nn.Module):
|
||||||
|
def __init__(self,
|
||||||
|
bert_config,
|
||||||
|
mh_dropout=0.1,
|
||||||
|
pred_clf_dropout=0.,
|
||||||
|
arg_clf_dropout=0.3,
|
||||||
|
n_arg_heads=8,
|
||||||
|
n_arg_layers=4,
|
||||||
|
pos_emb_dim=64,
|
||||||
|
pred_n_labels=3,
|
||||||
|
arg_n_labels=9):
|
||||||
|
super(Multi2OIE, self).__init__()
|
||||||
|
self.pred_n_labels = pred_n_labels
|
||||||
|
self.arg_n_labels = arg_n_labels
|
||||||
|
|
||||||
|
self.bert = BertModel.from_pretrained(
|
||||||
|
bert_config,
|
||||||
|
output_hidden_states=True)
|
||||||
|
d_model = self.bert.config.hidden_size
|
||||||
|
self.pred_dropout = nn.Dropout(pred_clf_dropout)
|
||||||
|
self.pred_classifier = nn.Linear(d_model, self.pred_n_labels)
|
||||||
|
|
||||||
|
self.position_emb = nn.Embedding(3, pos_emb_dim, padding_idx=0)
|
||||||
|
d_model += (d_model + pos_emb_dim)
|
||||||
|
arg_layer = ArgExtractorLayer(
|
||||||
|
d_model=d_model,
|
||||||
|
n_heads=n_arg_heads,
|
||||||
|
dropout=mh_dropout)
|
||||||
|
self.arg_module = ArgModule(arg_layer, n_arg_layers)
|
||||||
|
self.arg_dropout = nn.Dropout(arg_clf_dropout)
|
||||||
|
self.arg_classifier = nn.Linear(d_model, arg_n_labels)
|
||||||
|
|
||||||
|
def forward(self,
|
||||||
|
input_ids,
|
||||||
|
attention_mask,
|
||||||
|
predicate_mask=None,
|
||||||
|
predicate_hidden=None,
|
||||||
|
total_pred_labels=None,
|
||||||
|
arg_labels=None):
|
||||||
|
|
||||||
|
# predicate extraction
|
||||||
|
bert_hidden = self.bert(input_ids, attention_mask)[0]
|
||||||
|
pred_logit = self.pred_classifier(self.pred_dropout(bert_hidden))
|
||||||
|
|
||||||
|
# predicate loss
|
||||||
|
if total_pred_labels is not None:
|
||||||
|
loss_fct = nn.CrossEntropyLoss()
|
||||||
|
active_loss = attention_mask.view(-1) == 1
|
||||||
|
active_logits = pred_logit.view(-1, self.pred_n_labels)
|
||||||
|
active_labels = torch.where(
|
||||||
|
active_loss, total_pred_labels.view(-1),
|
||||||
|
torch.tensor(loss_fct.ignore_index).type_as(total_pred_labels))
|
||||||
|
pred_loss = loss_fct(active_logits, active_labels)
|
||||||
|
|
||||||
|
# inputs for argument extraction
|
||||||
|
pred_feature = _get_pred_feature(bert_hidden, predicate_mask)
|
||||||
|
position_vectors = self.position_emb(_get_position_idxs(predicate_mask, input_ids))
|
||||||
|
bert_hidden = torch.cat([bert_hidden, pred_feature, position_vectors], dim=2)
|
||||||
|
bert_hidden = bert_hidden.transpose(0, 1)
|
||||||
|
|
||||||
|
# argument extraction
|
||||||
|
arg_hidden = self.arg_module(bert_hidden, bert_hidden, predicate_mask)
|
||||||
|
arg_hidden = arg_hidden.transpose(0, 1)
|
||||||
|
arg_logit = self.arg_classifier(self.arg_dropout(arg_hidden))
|
||||||
|
|
||||||
|
# argument loss
|
||||||
|
if arg_labels is not None:
|
||||||
|
loss_fct = nn.CrossEntropyLoss()
|
||||||
|
active_loss = attention_mask.view(-1) == 1
|
||||||
|
active_logits = arg_logit.view(-1, self.arg_n_labels)
|
||||||
|
active_labels = torch.where(
|
||||||
|
active_loss, arg_labels.view(-1),
|
||||||
|
torch.tensor(loss_fct.ignore_index).type_as(arg_labels))
|
||||||
|
arg_loss = loss_fct(active_logits, active_labels)
|
||||||
|
|
||||||
|
# total loss
|
||||||
|
batch_loss = pred_loss + arg_loss
|
||||||
|
outputs = (batch_loss, pred_loss, arg_loss)
|
||||||
|
return outputs
|
||||||
|
|
||||||
|
def extract_predicate(self,
|
||||||
|
input_ids,
|
||||||
|
attention_mask):
|
||||||
|
bert_hidden = self.bert(input_ids, attention_mask)[0]
|
||||||
|
pred_logit = self.pred_classifier(bert_hidden)
|
||||||
|
return pred_logit, bert_hidden
|
||||||
|
|
||||||
|
def extract_argument(self,
|
||||||
|
input_ids,
|
||||||
|
predicate_hidden,
|
||||||
|
predicate_mask):
|
||||||
|
pred_feature = _get_pred_feature(predicate_hidden, predicate_mask)
|
||||||
|
position_vectors = self.position_emb(_get_position_idxs(predicate_mask, input_ids))
|
||||||
|
arg_input = torch.cat([predicate_hidden, pred_feature, position_vectors], dim=2)
|
||||||
|
arg_input = arg_input.transpose(0, 1)
|
||||||
|
arg_hidden = self.arg_module(arg_input, arg_input, predicate_mask)
|
||||||
|
arg_hidden = arg_hidden.transpose(0, 1)
|
||||||
|
return self.arg_classifier(arg_hidden)
|
||||||
|
|
||||||
|
|
||||||
|
class OieEvalDataset(Dataset):
|
||||||
|
def __init__(self, sentences, max_len, tokenizer_config):
|
||||||
|
self.sentences = sentences
|
||||||
|
self.tokenizer = BertTokenizer.from_pretrained(tokenizer_config)
|
||||||
|
self.vocab = self.tokenizer.vocab
|
||||||
|
self.max_len = max_len
|
||||||
|
|
||||||
|
self.pad_idx = self.vocab['[PAD]']
|
||||||
|
self.cls_idx = self.vocab['[CLS]']
|
||||||
|
self.sep_idx = self.vocab['[SEP]']
|
||||||
|
self.mask_idx = self.vocab['[MASK]']
|
||||||
|
|
||||||
|
def add_pad(self, token_ids):
|
||||||
|
diff = self.max_len - len(token_ids)
|
||||||
|
if diff > 0:
|
||||||
|
token_ids += [self.pad_idx] * diff
|
||||||
|
else:
|
||||||
|
token_ids = token_ids[:self.max_len - 1] + [self.sep_idx]
|
||||||
|
return token_ids
|
||||||
|
|
||||||
|
def idx2mask(self, token_ids):
|
||||||
|
return [token_id != self.pad_idx for token_id in token_ids]
|
||||||
|
|
||||||
|
def __len__(self):
|
||||||
|
return len(self.sentences)
|
||||||
|
|
||||||
|
def __getitem__(self, idx):
|
||||||
|
token_ids = self.add_pad(self.tokenizer.encode(self.sentences[idx]))
|
||||||
|
att_mask = self.idx2mask(token_ids)
|
||||||
|
token_strs = self.tokenizer.convert_ids_to_tokens(token_ids)
|
||||||
|
sentence = self.sentences[idx]
|
||||||
|
|
||||||
|
assert len(token_ids) == self.max_len
|
||||||
|
assert len(att_mask) == self.max_len
|
||||||
|
assert len(token_strs) == self.max_len
|
||||||
|
batch = [
|
||||||
|
torch.tensor(token_ids),
|
||||||
|
torch.tensor(att_mask),
|
||||||
|
token_strs,
|
||||||
|
sentence
|
||||||
|
]
|
||||||
|
return batch
|
||||||
|
|
||||||
|
|
||||||
|
def extract(model, loader, device, tokenizer):
|
||||||
|
|
||||||
|
# model.eval()
|
||||||
|
result = []
|
||||||
|
for step, batch in tqdm(enumerate(loader), desc='eval_steps', total=len(loader)):
|
||||||
|
token_strs = [[word for word in sent] for sent in np.asarray(batch[-2]).T]
|
||||||
|
sentences = batch[-1]
|
||||||
|
print(sentences)
|
||||||
|
token_ids, att_mask = map(lambda x: x.to(device), batch[:-2])
|
||||||
|
|
||||||
|
with torch.no_grad():
|
||||||
|
"""
|
||||||
|
We will iterate B(batch_size) times
|
||||||
|
because there are more than one predicate in one batch.
|
||||||
|
In feeding to argument extractor, # of predicates takes a role as batch size.
|
||||||
|
|
||||||
|
pred_logit: (B, L, 3)
|
||||||
|
pred_hidden: (B, L, D)
|
||||||
|
pred_tags: (B, P, L) ~ list of tensors, where P is # of predicate in each batch
|
||||||
|
"""
|
||||||
|
pred_logit, pred_hidden = model.extract_predicate(
|
||||||
|
input_ids=token_ids, attention_mask=att_mask)
|
||||||
|
pred_tags = torch.argmax(pred_logit, 2)
|
||||||
|
pred_tags = bio.filter_pred_tags(pred_tags, token_strs)
|
||||||
|
pred_tags = bio.get_single_predicate_idxs(pred_tags)
|
||||||
|
pred_probs = torch.nn.Softmax(2)(pred_logit)
|
||||||
|
|
||||||
|
# iterate B times (one iteration means extraction for one sentence)
|
||||||
|
for cur_pred_tags, cur_pred_hidden, cur_att_mask, cur_token_id, cur_pred_probs, token_str, sentence \
|
||||||
|
in zip(pred_tags, pred_hidden, att_mask, token_ids, pred_probs, token_strs, sentences):
|
||||||
|
|
||||||
|
# generate temporary batch for this sentence and feed to argument module
|
||||||
|
cur_pred_masks = bio.get_pred_mask(cur_pred_tags).to(device)
|
||||||
|
n_predicates = cur_pred_masks.shape[0]
|
||||||
|
if n_predicates == 0:
|
||||||
|
continue # if there is no predicate, we cannot extract.
|
||||||
|
cur_pred_hidden = torch.cat(n_predicates * [cur_pred_hidden.unsqueeze(0)])
|
||||||
|
cur_token_id = torch.cat(n_predicates * [cur_token_id.unsqueeze(0)])
|
||||||
|
cur_arg_logit = model.extract_argument(
|
||||||
|
input_ids=cur_token_id,
|
||||||
|
predicate_hidden=cur_pred_hidden,
|
||||||
|
predicate_mask=cur_pred_masks)
|
||||||
|
|
||||||
|
# filter and get argument tags with highest probability
|
||||||
|
cur_arg_tags = torch.argmax(cur_arg_logit, 2)
|
||||||
|
cur_arg_probs = torch.nn.Softmax(2)(cur_arg_logit)
|
||||||
|
cur_arg_tags = bio.filter_arg_tags(cur_arg_tags, cur_pred_tags, token_str)
|
||||||
|
|
||||||
|
# get string tuples and write results
|
||||||
|
cur_extractions, cur_extraction_idxs = bio.get_tuple(sentence, cur_pred_tags, cur_arg_tags, tokenizer)
|
||||||
|
cur_confidences = bio.get_confidence_score(cur_pred_probs, cur_arg_probs, cur_extraction_idxs)
|
||||||
|
for extraction, confidence in zip(cur_extractions, cur_confidences):
|
||||||
|
# print('\n')
|
||||||
|
# print("\t".join([sentence] + [str(1.0)] + extraction[:3]))
|
||||||
|
res_dict = {
|
||||||
|
'score': str(confidence),
|
||||||
|
'relation': extraction[0],
|
||||||
|
'arg1': extraction[1],
|
||||||
|
'arg2': extraction[2]
|
||||||
|
}
|
||||||
|
result.append(res_dict)
|
||||||
|
# print("\nExtraction Done.\n")
|
||||||
|
return result
|
||||||
|
|
||||||
|
|
||||||
|
class OIE:
|
||||||
|
def __init__(self, model_path, BERT, batch_size):
|
||||||
|
if torch.cuda.is_available():
|
||||||
|
self.device = torch.device("cuda")
|
||||||
|
else:
|
||||||
|
self.device = torch.device("cpu")
|
||||||
|
self.model = Multi2OIE(bert_config=BERT).to(self.device)
|
||||||
|
model_weights = torch.load(model_path, map_location=torch.device(self.device))
|
||||||
|
self.model.load_state_dict(model_weights)
|
||||||
|
self.max_len = 64
|
||||||
|
self.batch_size = batch_size
|
||||||
|
self.tokenizer_config = BERT
|
||||||
|
self.tokenizer = BertTokenizer.from_pretrained(BERT)
|
||||||
|
|
||||||
|
def predict(self, sentences):
|
||||||
|
loader = DataLoader(
|
||||||
|
dataset=OieEvalDataset(
|
||||||
|
sentences,
|
||||||
|
self.max_len,
|
||||||
|
self.tokenizer_config),
|
||||||
|
batch_size=self.batch_size,
|
||||||
|
num_workers=4,
|
||||||
|
pin_memory=True)
|
||||||
|
return extract(model=self.model, loader=loader, tokenizer=self.tokenizer, device=self.device)
|
182
3-NLP_services/src/Multi2OIE/README.md
Normal file
|
@ -0,0 +1,182 @@
|
||||||
|
# Multi^2OIE: <u>Multi</u>lingual Open Information Extraction Based on <u>Multi</u>-Head Attention with BERT
|
||||||
|
|
||||||
|
> Source code for learning Multi^2OIE for (multilingual) open information extraction.
|
||||||
|
|
||||||
|
## Paper
|
||||||
|
[**Multi^2OIE: <u>Multi</u>lingual Open Information Extraction Based on <u>Multi</u>-Head Attention with BERT**](https://arxiv.org/abs/2009.08128)<br>
|
||||||
|
[Youngbin Ro](https://github.com/youngbin-ro), [Yukyung Lee](https://github.com/yukyunglee), and [Pilsung Kang](https://github.com/pilsung-kang)*<br>
|
||||||
|
Accepted to Findings of ACL: EMNLP 2020. (*corresponding author)
|
||||||
|
|
||||||
|
<br>
|
||||||
|
|
||||||
|
## Overview
|
||||||
|
### What is Open Information Extraction (Open IE)?
|
||||||
|
[Niklaus et al. (2018)](https://www.aclweb.org/anthology/C18-1326/) describes Open IE as follows:
|
||||||
|
|
||||||
|
> Information extraction (IE) **<u>turns the unstructured information expressed in natural language text into a structured representation</u>** in the form of relational tuples consisting of a set of arguments and a phrase denoting a semantic relation between them: <arg1; rel; arg2>. (...) Unlike traditional IE methods, Open IE is **<u>not limited to a small set of target relations</u>** known in advance, but rather extracts all types of relations found in a text.
|
||||||
|
|
||||||
|
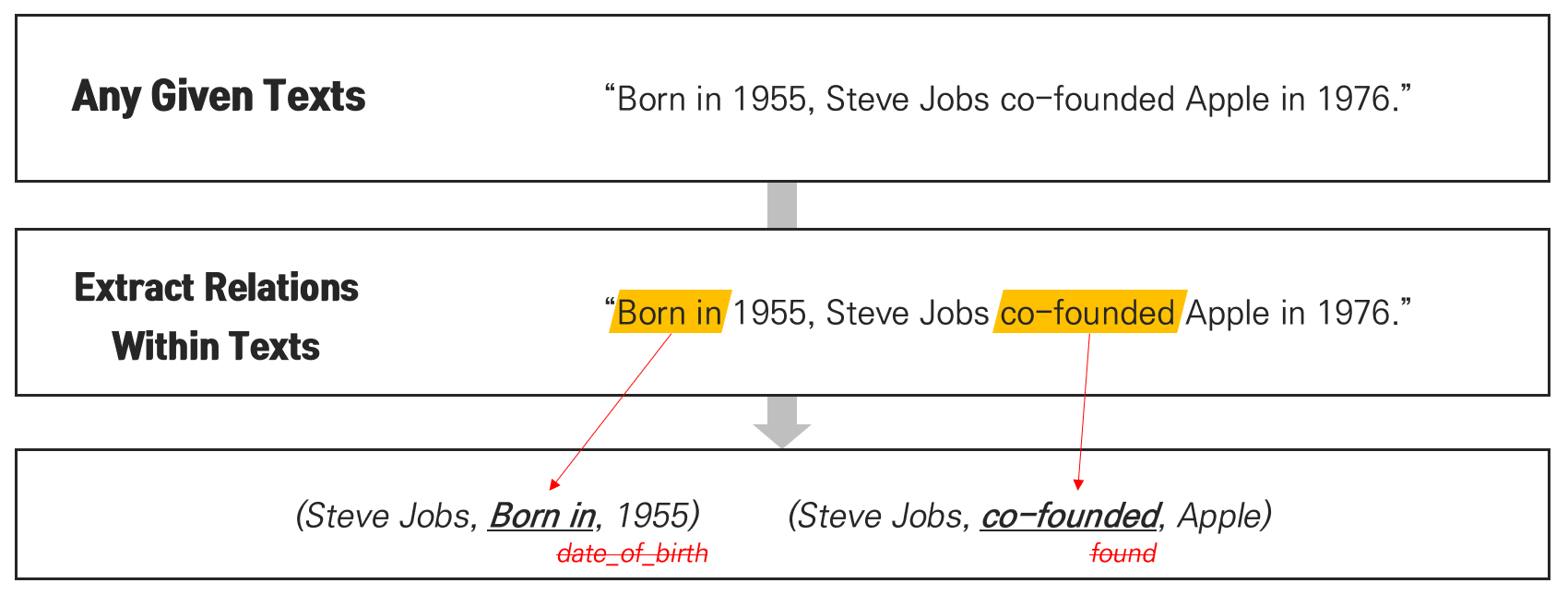
|
||||||
|
|
||||||
|
#### Note
|
||||||
|
- Systems adopting sequence generation scheme ([Cui et al., 2018](https://www.aclweb.org/anthology/P18-2065/); [Kolluru et al., 2020](https://www.aclweb.org/anthology/2020.acl-main.521/)) can extract (actually generate) relations outside of given texts.
|
||||||
|
- Multi^2OIE, however, is adopting sequence labeling scheme ([Stanovsky et al., 2018](https://www.aclweb.org/anthology/N18-1081/)) for computational efficiency and multilingual ability
|
||||||
|
|
||||||
|
|
||||||
|
|
||||||
|
### Our Approach
|
||||||
|
|
||||||
|
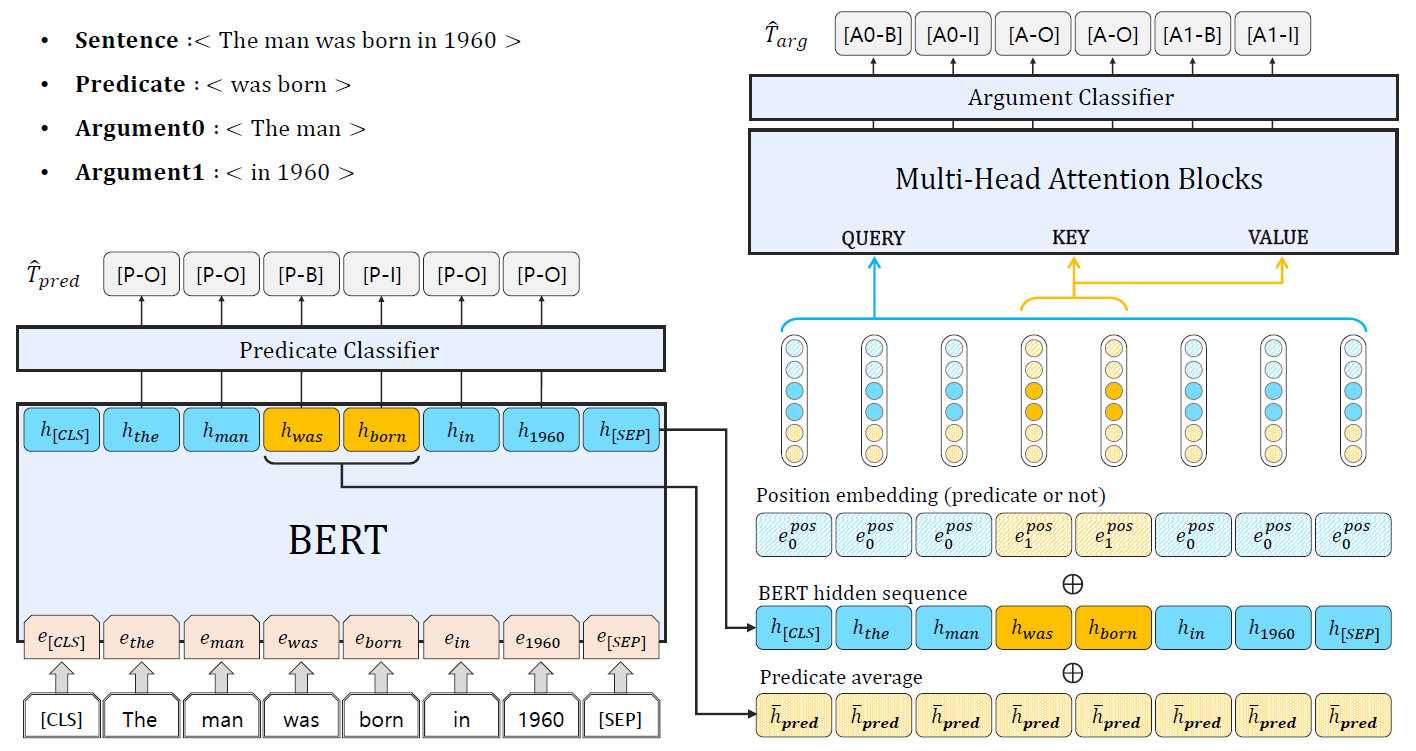
|
||||||
|
|
||||||
|
#### Step 1: Extract predicates (relations) from the input sentence using BERT
|
||||||
|
- Conduct token-level classification on the BERT output sequence
|
||||||
|
- Use BIO Tagging for representing arguments and predicates
|
||||||
|
|
||||||
|
#### Step 2: Extract arguments using multi-head attention blocks
|
||||||
|
- Concatenate BERT whole hidden sequence, average vector of hidden sequence at predicate position, and binary embedding vector indicating the token is included in predicate span.
|
||||||
|
- Apply multi-head attention operation over N times
|
||||||
|
- Query: whole hidden sequence
|
||||||
|
- Key-Value pairs: hidden states of predicate positions
|
||||||
|
- Conduct token-level classification on the multi-head attention output sequence
|
||||||
|
|
||||||
|
#### Multilingual Extraction
|
||||||
|
|
||||||
|
- Replace English BERT to Multilingual BERT
|
||||||
|
- Train the model only with English data
|
||||||
|
- Test the model in three difference languages (English, Spanish, and Portuguese) in zero-shot manner.
|
||||||
|
|
||||||
|
<br>
|
||||||
|
|
||||||
|
|
||||||
|
## Usage
|
||||||
|
|
||||||
|
### Prerequisites
|
||||||
|
|
||||||
|
- Python 3.7
|
||||||
|
|
||||||
|
- CUDA 10.0 or above
|
||||||
|
|
||||||
|
### Environmental Setup
|
||||||
|
|
||||||
|
#### Install
|
||||||
|
##### using 'conda' command,
|
||||||
|
~~~~
|
||||||
|
# this makes a new conda environment
|
||||||
|
conda env create -f environment.yml
|
||||||
|
conda activate multi2oie
|
||||||
|
~~~~
|
||||||
|
|
||||||
|
##### using 'pip' command,
|
||||||
|
~~~~
|
||||||
|
pip install -r requirements.txt
|
||||||
|
~~~~
|
||||||
|
|
||||||
|
#### NLTK setup
|
||||||
|
```
|
||||||
|
python -c "import nltk; nltk.download('stopwords')"
|
||||||
|
```
|
||||||
|
|
||||||
|
### Datasets
|
||||||
|
|
||||||
|
#### Dataset Released
|
||||||
|
- `openie4_train.pkl`: https://drive.google.com/file/d/1DrWj1CjLFIno-UBfLI3_uIratN4QY6Y3/view?usp=sharing
|
||||||
|
|
||||||
|
#### Do-it-yourself
|
||||||
|
Original data file (bootstrapped sample from OpenIE4; used in SpanOIE) can be downloaded from [here](https://drive.google.com/file/d/1AEfwbh3BQnsv2VM977cS4tEoldrayKB6/view).
|
||||||
|
Following download, put the downloaded data in './datasets' and use preprocess.py to convert the data into the format suitable for Multi^2OIE.
|
||||||
|
|
||||||
|
~~~~
|
||||||
|
cd utils
|
||||||
|
python preprocess.py \
|
||||||
|
--mode 'train' \
|
||||||
|
--data '../datasets/structured_data.json' \
|
||||||
|
--save_path '../datasets/openie4_train.pkl' \
|
||||||
|
--bert_config 'bert-base-cased' \
|
||||||
|
--max_len 64
|
||||||
|
~~~~
|
||||||
|
|
||||||
|
For multilingual training data, set **'bert_config'** as **'bert-base-multilingual-cased'**.
|
||||||
|
|
||||||
|
|
||||||
|
|
||||||
|
|
||||||
|
### Run the Code
|
||||||
|
|
||||||
|
#### Model Released
|
||||||
|
- English Model: https://drive.google.com/file/d/11BaLuGjMVVB16WHcyaHWLgL6dg0_9xHQ/view?usp=sharing
|
||||||
|
- Multilingual Model: https://drive.google.com/file/d/1lHQeetbacFOqvyPQ3ZzVUGPgn-zwTRA_/view?usp=sharing
|
||||||
|
|
||||||
|
We used TITAN RTX GPU for training, and the use of other GPU can make the final performance different.
|
||||||
|
|
||||||
|
##### for training,
|
||||||
|
|
||||||
|
~~~~
|
||||||
|
python main.py [--FLAGS]
|
||||||
|
~~~~
|
||||||
|
|
||||||
|
##### for testing,
|
||||||
|
|
||||||
|
~~~~
|
||||||
|
python test.py [--FLAGS]
|
||||||
|
~~~~
|
||||||
|
|
||||||
|
<br>
|
||||||
|
|
||||||
|
## Model Configurations
|
||||||
|
|
||||||
|
### # of Parameters
|
||||||
|
|
||||||
|
- Original BERT: 110M
|
||||||
|
- \+ Multi-Head Attention Blocks: 66M
|
||||||
|
|
||||||
|
|
||||||
|
|
||||||
|
### Hyper-parameters {& searching bounds}
|
||||||
|
|
||||||
|
- epochs: 1 {**1**, 2, 3}
|
||||||
|
- dropout rate for multi-head attention blocks: 0.2 {0.0, 0.1, **0.2**}
|
||||||
|
- dropout rate for argument classifier: 0.2 {0.0, 0.1, **0.2**, 0.3}
|
||||||
|
- batch size: 128 {64, **128**, 256, 512}
|
||||||
|
- learning rate: 3e-5 {2e-5, **3e-5**, 5e-5}
|
||||||
|
- number of multi-head attention heads: 8 {4, **8**}
|
||||||
|
- number of multi-head attention blocks: 4 {2, **4**, 8}
|
||||||
|
- position embedding dimension: 64 {**64**, 128, 256}
|
||||||
|
- gradient clipping norm: 1.0 (not tuned)
|
||||||
|
- learning rate warm-up steps: 10% of total steps (not tuned)
|
||||||
|
- for other unspecified parameters, the default values can be used.
|
||||||
|
<br>
|
||||||
|
|
||||||
|
## Expected Results
|
||||||
|
|
||||||
|
### Development set
|
||||||
|
|
||||||
|
#### OIE2016
|
||||||
|
|
||||||
|
- F1: 71.7
|
||||||
|
- AUC: 55.4
|
||||||
|
|
||||||
|
#### CaRB
|
||||||
|
|
||||||
|
- F1: 54.3
|
||||||
|
- AUC: 34.8
|
||||||
|
|
||||||
|
|
||||||
|
|
||||||
|
### Testing set
|
||||||
|
|
||||||
|
#### Re-OIE2016
|
||||||
|
|
||||||
|
- F1: 83.9
|
||||||
|
- AUC: 74.6
|
||||||
|
|
||||||
|
#### CaRB
|
||||||
|
|
||||||
|
- F1: 52.3
|
||||||
|
- AUC: 32.6
|
||||||
|
|
||||||
|
<br>
|
||||||
|
|
||||||
|
## References
|
||||||
|
|
||||||
|
- https://github.com/gabrielStanovsky/oie-benchmark
|
||||||
|
- https://github.com/dair-iitd/CaRB
|
||||||
|
- https://github.com/zhanjunlang/Span_OIE
|
0
3-NLP_services/src/Multi2OIE/__init__.py
Normal file
2548
3-NLP_services/src/Multi2OIE/carb/CaRB_dev.tsv
Normal file
2715
3-NLP_services/src/Multi2OIE/carb/CaRB_test.tsv
Normal file
0
3-NLP_services/src/Multi2OIE/carb/__init__.py
Normal file
21
3-NLP_services/src/Multi2OIE/carb/argument.py
Normal file
|
@ -0,0 +1,21 @@
|
||||||
|
import nltk
|
||||||
|
from operator import itemgetter
|
||||||
|
|
||||||
|
class Argument:
|
||||||
|
def __init__(self, arg):
|
||||||
|
self.words = [x for x in arg[0].strip().split(' ') if x]
|
||||||
|
self.posTags = map(itemgetter(1), nltk.pos_tag(self.words))
|
||||||
|
self.indices = arg[1]
|
||||||
|
self.feats = {}
|
||||||
|
|
||||||
|
def __str__(self):
|
||||||
|
return "({})".format('\t'.join(map(str,
|
||||||
|
[escape_special_chars(' '.join(self.words)),
|
||||||
|
str(self.indices)])))
|
||||||
|
|
||||||
|
COREF = 'coref'
|
||||||
|
|
||||||
|
## Helper functions
|
||||||
|
def escape_special_chars(s):
|
||||||
|
return s.replace('\t', '\\t')
|
||||||
|
|
380
3-NLP_services/src/Multi2OIE/carb/carb.py
Normal file
|
@ -0,0 +1,380 @@
|
||||||
|
'''
|
||||||
|
Usage:
|
||||||
|
benchmark --gold=GOLD_OIE --out=OUTPUT_FILE (--openiefive=OPENIE5 | --stanford=STANFORD_OIE | --ollie=OLLIE_OIE |--reverb=REVERB_OIE | --clausie=CLAUSIE_OIE | --openiefour=OPENIEFOUR_OIE | --props=PROPS_OIE | --tabbed=TABBED_OIE | --benchmarkGold=BENCHMARK_GOLD | --allennlp=ALLENNLP_OIE ) [--exactMatch | --predMatch | --lexicalMatch | --binaryMatch | --simpleMatch | --strictMatch] [--error-file=ERROR_FILE] [--binary]
|
||||||
|
|
||||||
|
Options:
|
||||||
|
--gold=GOLD_OIE The gold reference Open IE file (by default, it should be under ./oie_corpus/all.oie).
|
||||||
|
--benchmarkgold=GOLD_OIE The benchmark's gold reference.
|
||||||
|
--out-OUTPUT_FILE The output file, into which the precision recall curve will be written.
|
||||||
|
--clausie=CLAUSIE_OIE Read ClausIE format from file CLAUSIE_OIE.
|
||||||
|
--ollie=OLLIE_OIE Read OLLIE format from file OLLIE_OIE.
|
||||||
|
--openiefour=OPENIEFOUR_OIE Read Open IE 4 format from file OPENIEFOUR_OIE.
|
||||||
|
--openiefive=OPENIE5 Read Open IE 5 format from file OPENIE5.
|
||||||
|
--props=PROPS_OIE Read PropS format from file PROPS_OIE
|
||||||
|
--reverb=REVERB_OIE Read ReVerb format from file REVERB_OIE
|
||||||
|
--stanford=STANFORD_OIE Read Stanford format from file STANFORD_OIE
|
||||||
|
--tabbed=TABBED_OIE Read simple tab format file, where each line consists of:
|
||||||
|
sent, prob, pred,arg1, arg2, ...
|
||||||
|
--exactmatch Use exact match when judging whether an extraction is correct.
|
||||||
|
'''
|
||||||
|
from __future__ import division
|
||||||
|
import docopt
|
||||||
|
import string
|
||||||
|
import numpy as np
|
||||||
|
from sklearn.metrics import precision_recall_curve
|
||||||
|
from sklearn.metrics import auc, roc_auc_score
|
||||||
|
import re
|
||||||
|
import logging
|
||||||
|
import pdb
|
||||||
|
import ipdb
|
||||||
|
from _collections import defaultdict
|
||||||
|
from carb.goldReader import GoldReader
|
||||||
|
from carb.gold_relabel import Relabel_GoldReader
|
||||||
|
logging.basicConfig(level = logging.INFO)
|
||||||
|
|
||||||
|
from operator import itemgetter
|
||||||
|
import pprint
|
||||||
|
from copy import copy
|
||||||
|
pp = pprint.PrettyPrinter(indent=4)
|
||||||
|
|
||||||
|
class Benchmark:
|
||||||
|
''' Compare the gold OIE dataset against a predicted equivalent '''
|
||||||
|
def __init__(self, gold_fn):
|
||||||
|
''' Load gold Open IE, this will serve to compare against using the compare function '''
|
||||||
|
|
||||||
|
if 'Re-OIE2016' in gold_fn:
|
||||||
|
gr = Relabel_GoldReader()
|
||||||
|
else:
|
||||||
|
gr = GoldReader()
|
||||||
|
gr.read(gold_fn)
|
||||||
|
self.gold = gr.oie
|
||||||
|
|
||||||
|
def compare(self, predicted, matchingFunc, output_fn, error_file = None, binary=False):
|
||||||
|
''' Compare gold against predicted using a specified matching function.
|
||||||
|
Outputs PR curve to output_fn '''
|
||||||
|
|
||||||
|
y_true = []
|
||||||
|
y_scores = []
|
||||||
|
errors = []
|
||||||
|
correct = 0
|
||||||
|
incorrect = 0
|
||||||
|
|
||||||
|
correctTotal = 0
|
||||||
|
unmatchedCount = 0
|
||||||
|
predicted = Benchmark.normalizeDict(predicted)
|
||||||
|
gold = Benchmark.normalizeDict(self.gold)
|
||||||
|
if binary:
|
||||||
|
predicted = Benchmark.binarize(predicted)
|
||||||
|
gold = Benchmark.binarize(gold)
|
||||||
|
#gold = self.gold
|
||||||
|
|
||||||
|
# taking all distinct values of confidences as thresholds
|
||||||
|
confidence_thresholds = set()
|
||||||
|
for sent in predicted:
|
||||||
|
for predicted_ex in predicted[sent]:
|
||||||
|
confidence_thresholds.add(predicted_ex.confidence)
|
||||||
|
|
||||||
|
confidence_thresholds = sorted(list(confidence_thresholds))
|
||||||
|
num_conf = len(confidence_thresholds)
|
||||||
|
|
||||||
|
results = {}
|
||||||
|
p = np.zeros(num_conf)
|
||||||
|
pl = np.zeros(num_conf)
|
||||||
|
r = np.zeros(num_conf)
|
||||||
|
rl = np.zeros(num_conf)
|
||||||
|
|
||||||
|
for sent, goldExtractions in gold.items():
|
||||||
|
|
||||||
|
if sent in predicted:
|
||||||
|
predictedExtractions = predicted[sent]
|
||||||
|
else:
|
||||||
|
predictedExtractions = []
|
||||||
|
|
||||||
|
scores = [[None for _ in predictedExtractions] for __ in goldExtractions]
|
||||||
|
|
||||||
|
# print("***Gold Extractions***")
|
||||||
|
# print("\n".join([goldExtractions[i].pred + ' ' + " ".join(goldExtractions[i].args) for i in range(len(goldExtractions))]))
|
||||||
|
# print("***Predicted Extractions***")
|
||||||
|
# print("\n".join([predictedExtractions[i].pred+ " ".join(predictedExtractions[i].args) for i in range(len(predictedExtractions))]))
|
||||||
|
|
||||||
|
for i, goldEx in enumerate(goldExtractions):
|
||||||
|
for j, predictedEx in enumerate(predictedExtractions):
|
||||||
|
score = matchingFunc(goldEx, predictedEx,ignoreStopwords = True,ignoreCase = True)
|
||||||
|
scores[i][j] = score
|
||||||
|
|
||||||
|
|
||||||
|
# OPTIMISED GLOBAL MATCH
|
||||||
|
sent_confidences = [extraction.confidence for extraction in predictedExtractions]
|
||||||
|
sent_confidences.sort()
|
||||||
|
prev_c = 0
|
||||||
|
for conf in sent_confidences:
|
||||||
|
c = confidence_thresholds.index(conf)
|
||||||
|
ext_indices = []
|
||||||
|
for ext_indx, extraction in enumerate(predictedExtractions):
|
||||||
|
if extraction.confidence >= conf:
|
||||||
|
ext_indices.append(ext_indx)
|
||||||
|
|
||||||
|
recall_numerator = 0
|
||||||
|
for i, row in enumerate(scores):
|
||||||
|
max_recall_row = max([row[ext_indx][1] for ext_indx in ext_indices ], default=0)
|
||||||
|
recall_numerator += max_recall_row
|
||||||
|
|
||||||
|
precision_numerator = 0
|
||||||
|
|
||||||
|
selected_rows = []
|
||||||
|
selected_cols = []
|
||||||
|
num_precision_matches = min(len(scores), len(ext_indices))
|
||||||
|
for t in range(num_precision_matches):
|
||||||
|
matched_row = -1
|
||||||
|
matched_col = -1
|
||||||
|
matched_precision = -1 # initialised to <0 so that it updates whenever precision is 0 as well
|
||||||
|
for i in range(len(scores)):
|
||||||
|
if i in selected_rows:
|
||||||
|
continue
|
||||||
|
for ext_indx in ext_indices:
|
||||||
|
if ext_indx in selected_cols:
|
||||||
|
continue
|
||||||
|
if scores[i][ext_indx][0] > matched_precision:
|
||||||
|
matched_precision = scores[i][ext_indx][0]
|
||||||
|
matched_row = i
|
||||||
|
matched_col = ext_indx
|
||||||
|
|
||||||
|
selected_rows.append(matched_row)
|
||||||
|
selected_cols.append(matched_col)
|
||||||
|
precision_numerator += scores[matched_row][matched_col][0]
|
||||||
|
|
||||||
|
p[prev_c:c+1] += precision_numerator
|
||||||
|
pl[prev_c:c+1] += len(ext_indices)
|
||||||
|
r[prev_c:c+1] += recall_numerator
|
||||||
|
rl[prev_c:c+1] += len(scores)
|
||||||
|
|
||||||
|
prev_c = c+1
|
||||||
|
|
||||||
|
# for indices beyond the maximum sentence confidence, len(scores) has to be added to the denominator of recall
|
||||||
|
rl[prev_c:] += len(scores)
|
||||||
|
|
||||||
|
prec_scores = [a/b if b>0 else 1 for a,b in zip(p,pl) ]
|
||||||
|
rec_scores = [a/b if b>0 else 0 for a,b in zip(r,rl)]
|
||||||
|
|
||||||
|
f1s = [Benchmark.f1(p,r) for p,r in zip(prec_scores, rec_scores)]
|
||||||
|
try:
|
||||||
|
optimal_idx = np.nanargmax(f1s)
|
||||||
|
optimal = (prec_scores[optimal_idx], rec_scores[optimal_idx], f1s[optimal_idx])
|
||||||
|
except ValueError:
|
||||||
|
# When there is no prediction
|
||||||
|
optimal = (0,0,0)
|
||||||
|
|
||||||
|
# In order to calculate auc, we need to add the point corresponding to precision=1 , recall=0 to the PR-curve
|
||||||
|
temp_rec_scores = rec_scores.copy()
|
||||||
|
temp_prec_scores = prec_scores.copy()
|
||||||
|
temp_rec_scores.append(0)
|
||||||
|
temp_prec_scores.append(1)
|
||||||
|
# print("AUC: {}\t Optimal (precision, recall, F1): {}".format( np.round(auc(temp_rec_scores, temp_prec_scores),3), np.round(optimal,3) ))
|
||||||
|
|
||||||
|
with open(output_fn, 'w') as fout:
|
||||||
|
fout.write('{0}\t{1}\t{2}\n'.format("Precision", "Recall", "Confidence"))
|
||||||
|
for cur_p, cur_r, cur_conf in sorted(zip(prec_scores, rec_scores, confidence_thresholds), key = lambda cur: cur[1]):
|
||||||
|
fout.write('{0}\t{1}\t{2}\n'.format(cur_p, cur_r, cur_conf))
|
||||||
|
|
||||||
|
if len(f1s)>0:
|
||||||
|
rec_prec_dict = {rec: prec for rec, prec in zip(temp_rec_scores, temp_prec_scores)}
|
||||||
|
rec_prec_dict = sorted(rec_prec_dict.items(), key=lambda x: x[0])
|
||||||
|
temp_rec_scores = [rec for rec, _ in rec_prec_dict]
|
||||||
|
temp_prec_scores = [prec for _, prec in rec_prec_dict]
|
||||||
|
return np.round(auc(temp_rec_scores, temp_prec_scores),5), np.round(optimal,5)
|
||||||
|
else:
|
||||||
|
# When there is no prediction
|
||||||
|
return 0, (0,0,0)
|
||||||
|
|
||||||
|
@staticmethod
|
||||||
|
def binarize(extrs):
|
||||||
|
res = defaultdict(lambda: [])
|
||||||
|
for sent,extr in extrs.items():
|
||||||
|
for ex in extr:
|
||||||
|
#Add (a1, r, a2)
|
||||||
|
temp = copy(ex)
|
||||||
|
temp.args = ex.args[:2]
|
||||||
|
res[sent].append(temp)
|
||||||
|
|
||||||
|
if len(ex.args) <= 2:
|
||||||
|
continue
|
||||||
|
|
||||||
|
#Add (a1, r a2 , a3 ...)
|
||||||
|
for arg in ex.args[2:]:
|
||||||
|
temp.args = [ex.args[0]]
|
||||||
|
temp.pred = ex.pred + ' ' + ex.args[1]
|
||||||
|
words = arg.split()
|
||||||
|
|
||||||
|
#Add preposition of arg to rel
|
||||||
|
if words[0].lower() in Benchmark.PREPS:
|
||||||
|
temp.pred += ' ' + words[0]
|
||||||
|
words = words[1:]
|
||||||
|
temp.args.append(' '.join(words))
|
||||||
|
res[sent].append(temp)
|
||||||
|
|
||||||
|
return res
|
||||||
|
|
||||||
|
@staticmethod
|
||||||
|
def f1(prec, rec):
|
||||||
|
try:
|
||||||
|
return 2*prec*rec / (prec+rec)
|
||||||
|
except ZeroDivisionError:
|
||||||
|
return 0
|
||||||
|
|
||||||
|
@staticmethod
|
||||||
|
def aggregate_scores_greedily(scores):
|
||||||
|
# Greedy match: pick the prediction/gold match with the best f1 and exclude
|
||||||
|
# them both, until nothing left matches. Each input square is a [prec, rec]
|
||||||
|
# pair. Returns precision and recall as score-and-denominator pairs.
|
||||||
|
matches = []
|
||||||
|
while True:
|
||||||
|
max_s = 0
|
||||||
|
gold, pred = None, None
|
||||||
|
for i, gold_ss in enumerate(scores):
|
||||||
|
if i in [m[0] for m in matches]:
|
||||||
|
# Those are already taken rows
|
||||||
|
continue
|
||||||
|
for j, pred_s in enumerate(scores[i]):
|
||||||
|
if j in [m[1] for m in matches]:
|
||||||
|
# Those are used columns
|
||||||
|
continue
|
||||||
|
if pred_s and Benchmark.f1(*pred_s) > max_s:
|
||||||
|
max_s = Benchmark.f1(*pred_s)
|
||||||
|
gold = i
|
||||||
|
pred = j
|
||||||
|
if max_s == 0:
|
||||||
|
break
|
||||||
|
matches.append([gold, pred])
|
||||||
|
# Now that matches are determined, compute final scores.
|
||||||
|
prec_scores = [scores[i][j][0] for i,j in matches]
|
||||||
|
rec_scores = [scores[i][j][1] for i,j in matches]
|
||||||
|
total_prec = sum(prec_scores)
|
||||||
|
total_rec = sum(rec_scores)
|
||||||
|
scoring_metrics = {"precision" : [total_prec, len(scores[0])],
|
||||||
|
"recall" : [total_rec, len(scores)],
|
||||||
|
"precision_of_matches" : prec_scores,
|
||||||
|
"recall_of_matches" : rec_scores
|
||||||
|
}
|
||||||
|
return scoring_metrics
|
||||||
|
|
||||||
|
# Helper functions:
|
||||||
|
@staticmethod
|
||||||
|
def normalizeDict(d):
|
||||||
|
return dict([(Benchmark.normalizeKey(k), v) for k, v in d.items()])
|
||||||
|
|
||||||
|
@staticmethod
|
||||||
|
def normalizeKey(k):
|
||||||
|
# return Benchmark.removePunct(unicode(Benchmark.PTB_unescape(k.replace(' ','')), errors = 'ignore'))
|
||||||
|
return Benchmark.removePunct(str(Benchmark.PTB_unescape(k.replace(' ',''))))
|
||||||
|
|
||||||
|
@staticmethod
|
||||||
|
def PTB_escape(s):
|
||||||
|
for u, e in Benchmark.PTB_ESCAPES:
|
||||||
|
s = s.replace(u, e)
|
||||||
|
return s
|
||||||
|
|
||||||
|
@staticmethod
|
||||||
|
def PTB_unescape(s):
|
||||||
|
for u, e in Benchmark.PTB_ESCAPES:
|
||||||
|
s = s.replace(e, u)
|
||||||
|
return s
|
||||||
|
|
||||||
|
@staticmethod
|
||||||
|
def removePunct(s):
|
||||||
|
return Benchmark.regex.sub('', s)
|
||||||
|
|
||||||
|
# CONSTANTS
|
||||||
|
regex = re.compile('[%s]' % re.escape(string.punctuation))
|
||||||
|
|
||||||
|
# Penn treebank bracket escapes
|
||||||
|
# Taken from: https://github.com/nlplab/brat/blob/master/server/src/gtbtokenize.py
|
||||||
|
PTB_ESCAPES = [('(', '-LRB-'),
|
||||||
|
(')', '-RRB-'),
|
||||||
|
('[', '-LSB-'),
|
||||||
|
(']', '-RSB-'),
|
||||||
|
('{', '-LCB-'),
|
||||||
|
('}', '-RCB-'),]
|
||||||
|
|
||||||
|
PREPS = ['above','across','against','along','among','around','at','before','behind','below','beneath','beside','between','by','for','from','in','into','near','of','off','on','to','toward','under','upon','with','within']
|
||||||
|
|
||||||
|
def f_beta(precision, recall, beta = 1):
|
||||||
|
"""
|
||||||
|
Get F_beta score from precision and recall.
|
||||||
|
"""
|
||||||
|
beta = float(beta) # Make sure that results are in float
|
||||||
|
return (1 + pow(beta, 2)) * (precision * recall) / ((pow(beta, 2) * precision) + recall)
|
||||||
|
|
||||||
|
|
||||||
|
if __name__ == '__main__':
|
||||||
|
args = docopt.docopt(__doc__)
|
||||||
|
logging.debug(args)
|
||||||
|
|
||||||
|
if args['--stanford']:
|
||||||
|
predicted = StanfordReader()
|
||||||
|
predicted.read(args['--stanford'])
|
||||||
|
|
||||||
|
if args['--props']:
|
||||||
|
predicted = PropSReader()
|
||||||
|
predicted.read(args['--props'])
|
||||||
|
|
||||||
|
if args['--ollie']:
|
||||||
|
predicted = OllieReader()
|
||||||
|
predicted.read(args['--ollie'])
|
||||||
|
|
||||||
|
if args['--reverb']:
|
||||||
|
predicted = ReVerbReader()
|
||||||
|
predicted.read(args['--reverb'])
|
||||||
|
|
||||||
|
if args['--clausie']:
|
||||||
|
predicted = ClausieReader()
|
||||||
|
predicted.read(args['--clausie'])
|
||||||
|
|
||||||
|
if args['--openiefour']:
|
||||||
|
predicted = OpenieFourReader()
|
||||||
|
predicted.read(args['--openiefour'])
|
||||||
|
|
||||||
|
if args['--openiefive']:
|
||||||
|
predicted = OpenieFiveReader()
|
||||||
|
predicted.read(args['--openiefive'])
|
||||||
|
|
||||||
|
if args['--benchmarkGold']:
|
||||||
|
predicted = BenchmarkGoldReader()
|
||||||
|
predicted.read(args['--benchmarkGold'])
|
||||||
|
|
||||||
|
if args['--tabbed']:
|
||||||
|
predicted = TabReader()
|
||||||
|
predicted.read(args['--tabbed'])
|
||||||
|
|
||||||
|
if args['--binaryMatch']:
|
||||||
|
matchingFunc = Matcher.binary_tuple_match
|
||||||
|
|
||||||
|
elif args['--simpleMatch']:
|
||||||
|
matchingFunc = Matcher.simple_tuple_match
|
||||||
|
|
||||||
|
elif args['--exactMatch']:
|
||||||
|
matchingFunc = Matcher.argMatch
|
||||||
|
|
||||||
|
elif args['--predMatch']:
|
||||||
|
matchingFunc = Matcher.predMatch
|
||||||
|
|
||||||
|
elif args['--lexicalMatch']:
|
||||||
|
matchingFunc = Matcher.lexicalMatch
|
||||||
|
|
||||||
|
elif args['--strictMatch']:
|
||||||
|
matchingFunc = Matcher.tuple_match
|
||||||
|
|
||||||
|
else:
|
||||||
|
matchingFunc = Matcher.binary_linient_tuple_match
|
||||||
|
|
||||||
|
b = Benchmark(args['--gold'])
|
||||||
|
out_filename = args['--out']
|
||||||
|
|
||||||
|
logging.info("Writing PR curve of {} to {}".format(predicted.name, out_filename))
|
||||||
|
|
||||||
|
auc, optimal_f1_point = b.compare(predicted = predicted.oie,
|
||||||
|
matchingFunc = matchingFunc,
|
||||||
|
output_fn = out_filename,
|
||||||
|
error_file = args["--error-file"],
|
||||||
|
binary = args["--binary"])
|
||||||
|
|
||||||
|
print("AUC: {}\t Optimal (precision, recall, F1): {}".format( auc, optimal_f1_point ))
|
444
3-NLP_services/src/Multi2OIE/carb/extraction.py
Normal file
|
@ -0,0 +1,444 @@
|
||||||
|
from sklearn.preprocessing.data import binarize
|
||||||
|
from carb.argument import Argument
|
||||||
|
from operator import itemgetter
|
||||||
|
from collections import defaultdict
|
||||||
|
import nltk
|
||||||
|
import itertools
|
||||||
|
import logging
|
||||||
|
import numpy as np
|
||||||
|
import pdb
|
||||||
|
|
||||||
|
class Extraction:
|
||||||
|
"""
|
||||||
|
Stores sentence, single predicate and corresponding arguments.
|
||||||
|
"""
|
||||||
|
def __init__(self, pred, head_pred_index, sent, confidence, question_dist = '', index = -1):
|
||||||
|
self.pred = pred
|
||||||
|
self.head_pred_index = head_pred_index
|
||||||
|
self.sent = sent
|
||||||
|
self.args = []
|
||||||
|
self.confidence = confidence
|
||||||
|
self.matched = []
|
||||||
|
self.questions = {}
|
||||||
|
self.indsForQuestions = defaultdict(lambda: set())
|
||||||
|
self.is_mwp = False
|
||||||
|
self.question_dist = question_dist
|
||||||
|
self.index = index
|
||||||
|
|
||||||
|
def distArgFromPred(self, arg):
|
||||||
|
assert(len(self.pred) == 2)
|
||||||
|
dists = []
|
||||||
|
for x in self.pred[1]:
|
||||||
|
for y in arg.indices:
|
||||||
|
dists.append(abs(x - y))
|
||||||
|
|
||||||
|
return min(dists)
|
||||||
|
|
||||||
|
def argsByDistFromPred(self, question):
|
||||||
|
return sorted(self.questions[question], key = lambda arg: self.distArgFromPred(arg))
|
||||||
|
|
||||||
|
def addArg(self, arg, question = None):
|
||||||
|
self.args.append(arg)
|
||||||
|
if question:
|
||||||
|
self.questions[question] = self.questions.get(question,[]) + [Argument(arg)]
|
||||||
|
|
||||||
|
def noPronounArgs(self):
|
||||||
|
"""
|
||||||
|
Returns True iff all of this extraction's arguments are not pronouns.
|
||||||
|
"""
|
||||||
|
for (a, _) in self.args:
|
||||||
|
tokenized_arg = nltk.word_tokenize(a)
|
||||||
|
if len(tokenized_arg) == 1:
|
||||||
|
_, pos_tag = nltk.pos_tag(tokenized_arg)[0]
|
||||||
|
if ('PRP' in pos_tag):
|
||||||
|
return False
|
||||||
|
return True
|
||||||
|
|
||||||
|
def isContiguous(self):
|
||||||
|
return all([indices for (_, indices) in self.args])
|
||||||
|
|
||||||
|
def toBinary(self):
|
||||||
|
''' Try to represent this extraction's arguments as binary
|
||||||
|
If fails, this function will return an empty list. '''
|
||||||
|
|
||||||
|
ret = [self.elementToStr(self.pred)]
|
||||||
|
|
||||||
|
if len(self.args) == 2:
|
||||||
|
# we're in luck
|
||||||
|
return ret + [self.elementToStr(arg) for arg in self.args]
|
||||||
|
|
||||||
|
return []
|
||||||
|
|
||||||
|
if not self.isContiguous():
|
||||||
|
# give up on non contiguous arguments (as we need indexes)
|
||||||
|
return []
|
||||||
|
|
||||||
|
# otherwise, try to merge based on indices
|
||||||
|
# TODO: you can explore other methods for doing this
|
||||||
|
binarized = self.binarizeByIndex()
|
||||||
|
|
||||||
|
if binarized:
|
||||||
|
return ret + binarized
|
||||||
|
|
||||||
|
return []
|
||||||
|
|
||||||
|
|
||||||
|
def elementToStr(self, elem, print_indices = True):
|
||||||
|
''' formats an extraction element (pred or arg) as a raw string
|
||||||
|
removes indices and trailing spaces '''
|
||||||
|
if print_indices:
|
||||||
|
return str(elem)
|
||||||
|
if isinstance(elem, str):
|
||||||
|
return elem
|
||||||
|
if isinstance(elem, tuple):
|
||||||
|
ret = elem[0].rstrip().lstrip()
|
||||||
|
else:
|
||||||
|
ret = ' '.join(elem.words)
|
||||||
|
assert ret, "empty element? {0}".format(elem)
|
||||||
|
return ret
|
||||||
|
|
||||||
|
def binarizeByIndex(self):
|
||||||
|
extraction = [self.pred] + self.args
|
||||||
|
markPred = [(w, ind, i == 0) for i, (w, ind) in enumerate(extraction)]
|
||||||
|
sortedExtraction = sorted(markPred, key = lambda ws, indices, f : indices[0])
|
||||||
|
s = ' '.join(['{1} {0} {1}'.format(self.elementToStr(elem), SEP) if elem[2] else self.elementToStr(elem) for elem in sortedExtraction])
|
||||||
|
binArgs = [a for a in s.split(SEP) if a.rstrip().lstrip()]
|
||||||
|
|
||||||
|
if len(binArgs) == 2:
|
||||||
|
return binArgs
|
||||||
|
|
||||||
|
# failure
|
||||||
|
return []
|
||||||
|
|
||||||
|
def bow(self):
|
||||||
|
return ' '.join([self.elementToStr(elem) for elem in [self.pred] + self.args])
|
||||||
|
|
||||||
|
def getSortedArgs(self):
|
||||||
|
"""
|
||||||
|
Sort the list of arguments.
|
||||||
|
If a question distribution is provided - use it,
|
||||||
|
otherwise, default to the order of appearance in the sentence.
|
||||||
|
"""
|
||||||
|
if self.question_dist:
|
||||||
|
# There's a question distribtuion - use it
|
||||||
|
return self.sort_args_by_distribution()
|
||||||
|
ls = []
|
||||||
|
for q, args in self.questions.iteritems():
|
||||||
|
if (len(args) != 1):
|
||||||
|
logging.debug("Not one argument: {}".format(args))
|
||||||
|
continue
|
||||||
|
arg = args[0]
|
||||||
|
indices = list(self.indsForQuestions[q].union(arg.indices))
|
||||||
|
if not indices:
|
||||||
|
logging.debug("Empty indexes for arg {} -- backing to zero".format(arg))
|
||||||
|
indices = [0]
|
||||||
|
ls.append(((arg, q), indices))
|
||||||
|
return [a for a, _ in sorted(ls,
|
||||||
|
key = lambda _, indices: min(indices))]
|
||||||
|
|
||||||
|
def question_prob_for_loc(self, question, loc):
|
||||||
|
"""
|
||||||
|
Returns the probability of the given question leading to argument
|
||||||
|
appearing in the given location in the output slot.
|
||||||
|
"""
|
||||||
|
gen_question = generalize_question(question)
|
||||||
|
q_dist = self.question_dist[gen_question]
|
||||||
|
logging.debug("distribution of {}: {}".format(gen_question,
|
||||||
|
q_dist))
|
||||||
|
|
||||||
|
return float(q_dist.get(loc, 0)) / \
|
||||||
|
sum(q_dist.values())
|
||||||
|
|
||||||
|
def sort_args_by_distribution(self):
|
||||||
|
"""
|
||||||
|
Use this instance's question distribution (this func assumes it exists)
|
||||||
|
in determining the positioning of the arguments.
|
||||||
|
Greedy algorithm:
|
||||||
|
0. Decide on which argument will serve as the ``subject'' (first slot) of this extraction
|
||||||
|
0.1 Based on the most probable one for this spot
|
||||||
|
(special care is given to select the highly-influential subject position)
|
||||||
|
1. For all other arguments, sort arguments by the prevalance of their questions
|
||||||
|
2. For each argument:
|
||||||
|
2.1 Assign to it the most probable slot still available
|
||||||
|
2.2 If non such exist (fallback) - default to put it in the last location
|
||||||
|
"""
|
||||||
|
INF_LOC = 100 # Used as an impractical last argument
|
||||||
|
|
||||||
|
# Store arguments by slot
|
||||||
|
ret = {INF_LOC: []}
|
||||||
|
logging.debug("sorting: {}".format(self.questions))
|
||||||
|
|
||||||
|
# Find the most suitable arguemnt for the subject location
|
||||||
|
logging.debug("probs for subject: {}".format([(q, self.question_prob_for_loc(q, 0))
|
||||||
|
for (q, _) in self.questions.iteritems()]))
|
||||||
|
|
||||||
|
subj_question, subj_args = max(self.questions.iteritems(),
|
||||||
|
key = lambda q, _: self.question_prob_for_loc(q, 0))
|
||||||
|
|
||||||
|
ret[0] = [(subj_args[0], subj_question)]
|
||||||
|
|
||||||
|
# Find the rest
|
||||||
|
for (question, args) in sorted([(q, a)
|
||||||
|
for (q, a) in self.questions.iteritems() if (q not in [subj_question])],
|
||||||
|
key = lambda q, _: \
|
||||||
|
sum(self.question_dist[generalize_question(q)].values()),
|
||||||
|
reverse = True):
|
||||||
|
gen_question = generalize_question(question)
|
||||||
|
arg = args[0]
|
||||||
|
assigned_flag = False
|
||||||
|
for (loc, count) in sorted(self.question_dist[gen_question].iteritems(),
|
||||||
|
key = lambda _ , c: c,
|
||||||
|
reverse = True):
|
||||||
|
if loc not in ret:
|
||||||
|
# Found an empty slot for this item
|
||||||
|
# Place it there and break out
|
||||||
|
ret[loc] = [(arg, question)]
|
||||||
|
assigned_flag = True
|
||||||
|
break
|
||||||
|
|
||||||
|
if not assigned_flag:
|
||||||
|
# Add this argument to the non-assigned (hopefully doesn't happen much)
|
||||||
|
logging.debug("Couldn't find an open assignment for {}".format((arg, gen_question)))
|
||||||
|
ret[INF_LOC].append((arg, question))
|
||||||
|
|
||||||
|
logging.debug("Linearizing arg list: {}".format(ret))
|
||||||
|
|
||||||
|
# Finished iterating - consolidate and return a list of arguments
|
||||||
|
return [arg
|
||||||
|
for (_, arg_ls) in sorted(ret.iteritems(),
|
||||||
|
key = lambda k, v: int(k))
|
||||||
|
for arg in arg_ls]
|
||||||
|
|
||||||
|
|
||||||
|
def __str__(self):
|
||||||
|
pred_str = self.elementToStr(self.pred)
|
||||||
|
return '{}\t{}\t{}'.format(self.get_base_verb(pred_str),
|
||||||
|
self.compute_global_pred(pred_str,
|
||||||
|
self.questions.keys()),
|
||||||
|
'\t'.join([escape_special_chars(self.augment_arg_with_question(self.elementToStr(arg),
|
||||||
|
question))
|
||||||
|
for arg, question in self.getSortedArgs()]))
|
||||||
|
|
||||||
|
def get_base_verb(self, surface_pred):
|
||||||
|
"""
|
||||||
|
Given the surface pred, return the original annotated verb
|
||||||
|
"""
|
||||||
|
# Assumes that at this point the verb is always the last word
|
||||||
|
# in the surface predicate
|
||||||
|
return surface_pred.split(' ')[-1]
|
||||||
|
|
||||||
|
|
||||||
|
def compute_global_pred(self, surface_pred, questions):
|
||||||
|
"""
|
||||||
|
Given the surface pred and all instansiations of questions,
|
||||||
|
make global coherence decisions regarding the final form of the predicate
|
||||||
|
This should hopefully take care of multi word predicates and correct inflections
|
||||||
|
"""
|
||||||
|
from operator import itemgetter
|
||||||
|
split_surface = surface_pred.split(' ')
|
||||||
|
|
||||||
|
if len(split_surface) > 1:
|
||||||
|
# This predicate has a modal preceding the base verb
|
||||||
|
verb = split_surface[-1]
|
||||||
|
ret = split_surface[:-1] # get all of the elements in the modal
|
||||||
|
else:
|
||||||
|
verb = split_surface[0]
|
||||||
|
ret = []
|
||||||
|
|
||||||
|
split_questions = map(lambda question: question.split(' '),
|
||||||
|
questions)
|
||||||
|
|
||||||
|
preds = map(normalize_element,
|
||||||
|
map(itemgetter(QUESTION_TRG_INDEX),
|
||||||
|
split_questions))
|
||||||
|
if len(set(preds)) > 1:
|
||||||
|
# This predicate is appears in multiple ways, let's stick to the base form
|
||||||
|
ret.append(verb)
|
||||||
|
|
||||||
|
if len(set(preds)) == 1:
|
||||||
|
# Change the predciate to the inflected form
|
||||||
|
# if there's exactly one way in which the predicate is conveyed
|
||||||
|
ret.append(preds[0])
|
||||||
|
|
||||||
|
pps = map(normalize_element,
|
||||||
|
map(itemgetter(QUESTION_PP_INDEX),
|
||||||
|
split_questions))
|
||||||
|
|
||||||
|
obj2s = map(normalize_element,
|
||||||
|
map(itemgetter(QUESTION_OBJ2_INDEX),
|
||||||
|
split_questions))
|
||||||
|
|
||||||
|
if (len(set(pps)) == 1):
|
||||||
|
# If all questions for the predicate include the same pp attachemnt -
|
||||||
|
# assume it's a multiword predicate
|
||||||
|
self.is_mwp = True # Signal to arguments that they shouldn't take the preposition
|
||||||
|
ret.append(pps[0])
|
||||||
|
|
||||||
|
# Concat all elements in the predicate and return
|
||||||
|
return " ".join(ret).strip()
|
||||||
|
|
||||||
|
|
||||||
|
def augment_arg_with_question(self, arg, question):
|
||||||
|
"""
|
||||||
|
Decide what elements from the question to incorporate in the given
|
||||||
|
corresponding argument
|
||||||
|
"""
|
||||||
|
# Parse question
|
||||||
|
wh, aux, sbj, trg, obj1, pp, obj2 = map(normalize_element,
|
||||||
|
question.split(' ')[:-1]) # Last split is the question mark
|
||||||
|
|
||||||
|
# Place preposition in argument
|
||||||
|
# This is safer when dealing with n-ary arguments, as it's directly attaches to the
|
||||||
|
# appropriate argument
|
||||||
|
if (not self.is_mwp) and pp and (not obj2):
|
||||||
|
if not(arg.startswith("{} ".format(pp))):
|
||||||
|
# Avoid repeating the preporition in cases where both question and answer contain it
|
||||||
|
return " ".join([pp,
|
||||||
|
arg])
|
||||||
|
|
||||||
|
# Normal cases
|
||||||
|
return arg
|
||||||
|
|
||||||
|
def clusterScore(self, cluster):
|
||||||
|
"""
|
||||||
|
Calculate cluster density score as the mean distance of the maximum distance of each slot.
|
||||||
|
Lower score represents a denser cluster.
|
||||||
|
"""
|
||||||
|
logging.debug("*-*-*- Cluster: {}".format(cluster))
|
||||||
|
|
||||||
|
# Find global centroid
|
||||||
|
arr = np.array([x for ls in cluster for x in ls])
|
||||||
|
centroid = np.sum(arr)/arr.shape[0]
|
||||||
|
logging.debug("Centroid: {}".format(centroid))
|
||||||
|
|
||||||
|
# Calculate mean over all maxmimum points
|
||||||
|
return np.average([max([abs(x - centroid) for x in ls]) for ls in cluster])
|
||||||
|
|
||||||
|
def resolveAmbiguity(self):
|
||||||
|
"""
|
||||||
|
Heursitic to map the elments (argument and predicates) of this extraction
|
||||||
|
back to the indices of the sentence.
|
||||||
|
"""
|
||||||
|
## TODO: This removes arguments for which there was no consecutive span found
|
||||||
|
## Part of these are non-consecutive arguments,
|
||||||
|
## but other could be a bug in recognizing some punctuation marks
|
||||||
|
|
||||||
|
elements = [self.pred] \
|
||||||
|
+ [(s, indices)
|
||||||
|
for (s, indices)
|
||||||
|
in self.args
|
||||||
|
if indices]
|
||||||
|
logging.debug("Resolving ambiguity in: {}".format(elements))
|
||||||
|
|
||||||
|
# Collect all possible combinations of arguments and predicate indices
|
||||||
|
# (hopefully it's not too much)
|
||||||
|
all_combinations = list(itertools.product(*map(itemgetter(1), elements)))
|
||||||
|
logging.debug("Number of combinations: {}".format(len(all_combinations)))
|
||||||
|
|
||||||
|
# Choose the ones with best clustering and unfold them
|
||||||
|
resolved_elements = zip(map(itemgetter(0), elements),
|
||||||
|
min(all_combinations,
|
||||||
|
key = lambda cluster: self.clusterScore(cluster)))
|
||||||
|
logging.debug("Resolved elements = {}".format(resolved_elements))
|
||||||
|
|
||||||
|
self.pred = resolved_elements[0]
|
||||||
|
self.args = resolved_elements[1:]
|
||||||
|
|
||||||
|
def conll(self, external_feats = {}):
|
||||||
|
"""
|
||||||
|
Return a CoNLL string representation of this extraction
|
||||||
|
"""
|
||||||
|
return '\n'.join(["\t".join(map(str,
|
||||||
|
[i, w] + \
|
||||||
|
list(self.pred) + \
|
||||||
|
[self.head_pred_index] + \
|
||||||
|
external_feats + \
|
||||||
|
[self.get_label(i)]))
|
||||||
|
for (i, w)
|
||||||
|
in enumerate(self.sent.split(" "))]) + '\n'
|
||||||
|
|
||||||
|
def get_label(self, index):
|
||||||
|
"""
|
||||||
|
Given an index of a word in the sentence -- returns the appropriate BIO conll label
|
||||||
|
Assumes that ambiguation was already resolved.
|
||||||
|
"""
|
||||||
|
# Get the element(s) in which this index appears
|
||||||
|
ent = [(elem_ind, elem)
|
||||||
|
for (elem_ind, elem)
|
||||||
|
in enumerate(map(itemgetter(1),
|
||||||
|
[self.pred] + self.args))
|
||||||
|
if index in elem]
|
||||||
|
|
||||||
|
if not ent:
|
||||||
|
# index doesnt appear in any element
|
||||||
|
return "O"
|
||||||
|
|
||||||
|
if len(ent) > 1:
|
||||||
|
# The same word appears in two different answers
|
||||||
|
# In this case we choose the first one as label
|
||||||
|
logging.warn("Index {} appears in one than more element: {}".\
|
||||||
|
format(index,
|
||||||
|
"\t".join(map(str,
|
||||||
|
[ent,
|
||||||
|
self.sent,
|
||||||
|
self.pred,
|
||||||
|
self.args]))))
|
||||||
|
|
||||||
|
## Some indices appear in more than one argument (ones where the above message appears)
|
||||||
|
## From empricial observation, these seem to mostly consist of different levels of granularity:
|
||||||
|
## what had _ been taken _ _ _ ? loan commitments topping $ 3 billion
|
||||||
|
## how much had _ been taken _ _ _ ? topping $ 3 billion
|
||||||
|
## In these cases we heuristically choose the shorter answer span, hopefully creating minimal spans
|
||||||
|
## E.g., in this example two arguemnts are created: (loan commitments, topping $ 3 billion)
|
||||||
|
|
||||||
|
elem_ind, elem = min(ent, key = lambda _, ls: len(ls))
|
||||||
|
|
||||||
|
# Distinguish between predicate and arguments
|
||||||
|
prefix = "P" if elem_ind == 0 else "A{}".format(elem_ind - 1)
|
||||||
|
|
||||||
|
# Distinguish between Beginning and Inside labels
|
||||||
|
suffix = "B" if index == elem[0] else "I"
|
||||||
|
|
||||||
|
return "{}-{}".format(prefix, suffix)
|
||||||
|
|
||||||
|
def __str__(self):
|
||||||
|
return '{0}\t{1}'.format(self.elementToStr(self.pred,
|
||||||
|
print_indices = True),
|
||||||
|
'\t'.join([self.elementToStr(arg)
|
||||||
|
for arg
|
||||||
|
in self.args]))
|
||||||
|
|
||||||
|
# Flatten a list of lists
|
||||||
|
flatten = lambda l: [item for sublist in l for item in sublist]
|
||||||
|
|
||||||
|
|
||||||
|
def normalize_element(elem):
|
||||||
|
"""
|
||||||
|
Return a surface form of the given question element.
|
||||||
|
the output should be properly able to precede a predicate (or blank otherwise)
|
||||||
|
"""
|
||||||
|
return elem.replace("_", " ") \
|
||||||
|
if (elem != "_")\
|
||||||
|
else ""
|
||||||
|
|
||||||
|
## Helper functions
|
||||||
|
def escape_special_chars(s):
|
||||||
|
return s.replace('\t', '\\t')
|
||||||
|
|
||||||
|
|
||||||
|
def generalize_question(question):
|
||||||
|
"""
|
||||||
|
Given a question in the context of the sentence and the predicate index within
|
||||||
|
the question - return a generalized version which extracts only order-imposing features
|
||||||
|
"""
|
||||||
|
import nltk # Using nltk since couldn't get spaCy to agree on the tokenization
|
||||||
|
wh, aux, sbj, trg, obj1, pp, obj2 = question.split(' ')[:-1] # Last split is the question mark
|
||||||
|
return ' '.join([wh, sbj, obj1])
|
||||||
|
|
||||||
|
|
||||||
|
|
||||||
|
## CONSTANTS
|
||||||
|
SEP = ';;;'
|
||||||
|
QUESTION_TRG_INDEX = 3 # index of the predicate within the question
|
||||||
|
QUESTION_PP_INDEX = 5
|
||||||
|
QUESTION_OBJ2_INDEX = 6
|
53
3-NLP_services/src/Multi2OIE/carb/goldReader.py
Normal file
|
@ -0,0 +1,53 @@
|
||||||
|
from carb.oieReader import OieReader
|
||||||
|
from carb.extraction import Extraction
|
||||||
|
from _collections import defaultdict
|
||||||
|
import ipdb
|
||||||
|
|
||||||
|
class GoldReader(OieReader):
|
||||||
|
|
||||||
|
# Path relative to repo root folder
|
||||||
|
default_filename = './oie_corpus/all.oie'
|
||||||
|
|
||||||
|
def __init__(self):
|
||||||
|
self.name = 'Gold'
|
||||||
|
|
||||||
|
def read(self, fn):
|
||||||
|
d = defaultdict(lambda: [])
|
||||||
|
multilingual = False
|
||||||
|
for lang in ['spanish']:
|
||||||
|
if lang in fn:
|
||||||
|
multilingual = True
|
||||||
|
encoding = lang
|
||||||
|
break
|
||||||
|
if multilingual and encoding == 'spanish':
|
||||||
|
fin = open(fn, 'r', encoding='latin-1')
|
||||||
|
else:
|
||||||
|
fin = open(fn)
|
||||||
|
#with open(fn) as fin:
|
||||||
|
for line_ind, line in enumerate(fin):
|
||||||
|
data = line.strip().split('\t')
|
||||||
|
text, rel = data[:2]
|
||||||
|
args = data[2:]
|
||||||
|
confidence = 1
|
||||||
|
|
||||||
|
curExtraction = Extraction(pred = rel.strip(),
|
||||||
|
head_pred_index = None,
|
||||||
|
sent = text.strip(),
|
||||||
|
confidence = float(confidence),
|
||||||
|
index = line_ind)
|
||||||
|
for arg in args:
|
||||||
|
if "C: " in arg:
|
||||||
|
continue
|
||||||
|
curExtraction.addArg(arg.strip())
|
||||||
|
|
||||||
|
d[text.strip()].append(curExtraction)
|
||||||
|
self.oie = d
|
||||||
|
|
||||||
|
|
||||||
|
if __name__ == '__main__' :
|
||||||
|
g = GoldReader()
|
||||||
|
g.read('../oie_corpus/all.oie', includeNominal = False)
|
||||||
|
d = g.oie
|
||||||
|
e = d.items()[0]
|
||||||
|
print(e[1][0].bow())
|
||||||
|
print(g.count())
|
46
3-NLP_services/src/Multi2OIE/carb/gold_relabel.py
Normal file
|
@ -0,0 +1,46 @@
|
||||||
|
from carb.oieReader import OieReader
|
||||||
|
from carb.extraction import Extraction
|
||||||
|
from _collections import defaultdict
|
||||||
|
import json
|
||||||
|
|
||||||
|
class Relabel_GoldReader(OieReader):
|
||||||
|
|
||||||
|
# Path relative to repo root folder
|
||||||
|
default_filename = './oie_corpus/all.oie'
|
||||||
|
|
||||||
|
def __init__(self):
|
||||||
|
self.name = 'Relabel_Gold'
|
||||||
|
|
||||||
|
def read(self, fn):
|
||||||
|
d = defaultdict(lambda: [])
|
||||||
|
with open(fn) as fin:
|
||||||
|
data = json.load(fin)
|
||||||
|
for sentence in data:
|
||||||
|
tuples = data[sentence]
|
||||||
|
for t in tuples:
|
||||||
|
if t["pred"].strip() == "<be>":
|
||||||
|
rel = "[is]"
|
||||||
|
else:
|
||||||
|
rel = t["pred"].replace("<be> ","")
|
||||||
|
confidence = 1
|
||||||
|
|
||||||
|
curExtraction = Extraction(pred = rel,
|
||||||
|
head_pred_index = None,
|
||||||
|
sent = sentence,
|
||||||
|
confidence = float(confidence),
|
||||||
|
index = None)
|
||||||
|
if t["arg0"] != "":
|
||||||
|
curExtraction.addArg(t["arg0"])
|
||||||
|
if t["arg1"] != "":
|
||||||
|
curExtraction.addArg(t["arg1"])
|
||||||
|
if t["arg2"] != "":
|
||||||
|
curExtraction.addArg(t["arg2"])
|
||||||
|
if t["arg3"] != "":
|
||||||
|
curExtraction.addArg(t["arg3"])
|
||||||
|
if t["temp"] != "":
|
||||||
|
curExtraction.addArg(t["temp"])
|
||||||
|
if t["loc"] != "":
|
||||||
|
curExtraction.addArg(t["loc"])
|
||||||
|
|
||||||
|
d[sentence].append(curExtraction)
|
||||||
|
self.oie = d
|
340
3-NLP_services/src/Multi2OIE/carb/matcher.py
Normal file
|
@ -0,0 +1,340 @@
|
||||||
|
from __future__ import division
|
||||||
|
import string
|
||||||
|
from nltk.translate.bleu_score import sentence_bleu
|
||||||
|
from nltk.corpus import stopwords
|
||||||
|
from copy import copy
|
||||||
|
import ipdb
|
||||||
|
|
||||||
|
class Matcher:
|
||||||
|
@staticmethod
|
||||||
|
def bowMatch(ref, ex, ignoreStopwords, ignoreCase):
|
||||||
|
"""
|
||||||
|
A binary function testing for exact lexical match (ignoring ordering) between reference
|
||||||
|
and predicted extraction
|
||||||
|
"""
|
||||||
|
s1 = ref.bow()
|
||||||
|
s2 = ex.bow()
|
||||||
|
if ignoreCase:
|
||||||
|
s1 = s1.lower()
|
||||||
|
s2 = s2.lower()
|
||||||
|
|
||||||
|
s1Words = s1.split(' ')
|
||||||
|
s2Words = s2.split(' ')
|
||||||
|
|
||||||
|
if ignoreStopwords:
|
||||||
|
s1Words = Matcher.removeStopwords(s1Words)
|
||||||
|
s2Words = Matcher.removeStopwords(s2Words)
|
||||||
|
|
||||||
|
return sorted(s1Words) == sorted(s2Words)
|
||||||
|
|
||||||
|
@staticmethod
|
||||||
|
def predMatch(ref, ex, ignoreStopwords, ignoreCase):
|
||||||
|
"""
|
||||||
|
Return whehter gold and predicted extractions agree on the predicate
|
||||||
|
"""
|
||||||
|
s1 = ref.elementToStr(ref.pred)
|
||||||
|
s2 = ex.elementToStr(ex.pred)
|
||||||
|
if ignoreCase:
|
||||||
|
s1 = s1.lower()
|
||||||
|
s2 = s2.lower()
|
||||||
|
|
||||||
|
s1Words = s1.split(' ')
|
||||||
|
s2Words = s2.split(' ')
|
||||||
|
|
||||||
|
if ignoreStopwords:
|
||||||
|
s1Words = Matcher.removeStopwords(s1Words)
|
||||||
|
s2Words = Matcher.removeStopwords(s2Words)
|
||||||
|
|
||||||
|
return s1Words == s2Words
|
||||||
|
|
||||||
|
|
||||||
|
@staticmethod
|
||||||
|
def argMatch(ref, ex, ignoreStopwords, ignoreCase):
|
||||||
|
"""
|
||||||
|
Return whehter gold and predicted extractions agree on the arguments
|
||||||
|
"""
|
||||||
|
sRef = ' '.join([ref.elementToStr(elem) for elem in ref.args])
|
||||||
|
sEx = ' '.join([ex.elementToStr(elem) for elem in ex.args])
|
||||||
|
|
||||||
|
count = 0
|
||||||
|
|
||||||
|
for w1 in sRef:
|
||||||
|
for w2 in sEx:
|
||||||
|
if w1 == w2:
|
||||||
|
count += 1
|
||||||
|
|
||||||
|
# We check how well does the extraction lexically cover the reference
|
||||||
|
# Note: this is somewhat lenient as it doesn't penalize the extraction for
|
||||||
|
# being too long
|
||||||
|
coverage = float(count) / len(sRef)
|
||||||
|
|
||||||
|
|
||||||
|
return coverage > Matcher.LEXICAL_THRESHOLD
|
||||||
|
|
||||||
|
@staticmethod
|
||||||
|
def bleuMatch(ref, ex, ignoreStopwords, ignoreCase):
|
||||||
|
sRef = ref.bow()
|
||||||
|
sEx = ex.bow()
|
||||||
|
bleu = sentence_bleu(references = [sRef.split(' ')], hypothesis = sEx.split(' '))
|
||||||
|
return bleu > Matcher.BLEU_THRESHOLD
|
||||||
|
|
||||||
|
@staticmethod
|
||||||
|
def lexicalMatch(ref, ex, ignoreStopwords, ignoreCase):
|
||||||
|
sRef = ref.bow().split(' ')
|
||||||
|
sEx = ex.bow().split(' ')
|
||||||
|
count = 0
|
||||||
|
#for w1 in sRef:
|
||||||
|
# if w1 in sEx:
|
||||||
|
# count += 1
|
||||||
|
# sEx.remove(w1)
|
||||||
|
for w1 in sRef:
|
||||||
|
for w2 in sEx:
|
||||||
|
if w1 == w2:
|
||||||
|
count += 1
|
||||||
|
|
||||||
|
# We check how well does the extraction lexically cover the reference
|
||||||
|
# Note: this is somewhat lenient as it doesn't penalize the extraction for
|
||||||
|
# being too long
|
||||||
|
coverage = float(count) / len(sRef)
|
||||||
|
|
||||||
|
return coverage > Matcher.LEXICAL_THRESHOLD
|
||||||
|
|
||||||
|
@staticmethod
|
||||||
|
def tuple_match(ref, ex, ignoreStopwords, ignoreCase):
|
||||||
|
precision = [0, 0] # 0 out of 0 predicted words match
|
||||||
|
recall = [0, 0] # 0 out of 0 reference words match
|
||||||
|
# If, for each part, any word is the same as a reference word, then it's a match.
|
||||||
|
|
||||||
|
predicted_words = ex.pred.split()
|
||||||
|
gold_words = ref.pred.split()
|
||||||
|
precision[1] += len(predicted_words)
|
||||||
|
recall[1] += len(gold_words)
|
||||||
|
|
||||||
|
# matching_words = sum(1 for w in predicted_words if w in gold_words)
|
||||||
|
matching_words = 0
|
||||||
|
for w in gold_words:
|
||||||
|
if w in predicted_words:
|
||||||
|
matching_words += 1
|
||||||
|
predicted_words.remove(w)
|
||||||
|
|
||||||
|
if matching_words == 0:
|
||||||
|
return False # t <-> gt is not a match
|
||||||
|
precision[0] += matching_words
|
||||||
|
recall[0] += matching_words
|
||||||
|
|
||||||
|
for i in range(len(ref.args)):
|
||||||
|
gold_words = ref.args[i].split()
|
||||||
|
recall[1] += len(gold_words)
|
||||||
|
if len(ex.args) <= i:
|
||||||
|
if i<2:
|
||||||
|
return False
|
||||||
|
else:
|
||||||
|
continue
|
||||||
|
predicted_words = ex.args[i].split()
|
||||||
|
precision[1] += len(predicted_words)
|
||||||
|
matching_words = 0
|
||||||
|
for w in gold_words:
|
||||||
|
if w in predicted_words:
|
||||||
|
matching_words += 1
|
||||||
|
predicted_words.remove(w)
|
||||||
|
|
||||||
|
if matching_words == 0 and i<2:
|
||||||
|
return False # t <-> gt is not a match
|
||||||
|
precision[0] += matching_words
|
||||||
|
# Currently this slightly penalises systems when the reference
|
||||||
|
# reformulates the sentence words, because the reformulation doesn't
|
||||||
|
# match the predicted word. It's a one-wrong-word penalty to precision,
|
||||||
|
# to all systems that correctly extracted the reformulated word.
|
||||||
|
recall[0] += matching_words
|
||||||
|
|
||||||
|
prec = 1.0 * precision[0] / precision[1]
|
||||||
|
rec = 1.0 * recall[0] / recall[1]
|
||||||
|
return [prec, rec]
|
||||||
|
|
||||||
|
# STRICTER LINIENT MATCH
|
||||||
|
def linient_tuple_match(ref, ex, ignoreStopwords, ignoreCase):
|
||||||
|
precision = [0, 0] # 0 out of 0 predicted words match
|
||||||
|
recall = [0, 0] # 0 out of 0 reference words match
|
||||||
|
# If, for each part, any word is the same as a reference word, then it's a match.
|
||||||
|
|
||||||
|
predicted_words = ex.pred.split()
|
||||||
|
gold_words = ref.pred.split()
|
||||||
|
precision[1] += len(predicted_words)
|
||||||
|
recall[1] += len(gold_words)
|
||||||
|
|
||||||
|
# matching_words = sum(1 for w in predicted_words if w in gold_words)
|
||||||
|
matching_words = 0
|
||||||
|
for w in gold_words:
|
||||||
|
if w in predicted_words:
|
||||||
|
matching_words += 1
|
||||||
|
predicted_words.remove(w)
|
||||||
|
|
||||||
|
# matching 'be' with its different forms
|
||||||
|
forms_of_be = ["be","is","am","are","was","were","been","being"]
|
||||||
|
if "be" in predicted_words:
|
||||||
|
for form in forms_of_be:
|
||||||
|
if form in gold_words:
|
||||||
|
matching_words += 1
|
||||||
|
predicted_words.remove("be")
|
||||||
|
break
|
||||||
|
|
||||||
|
if matching_words == 0:
|
||||||
|
return [0,0] # t <-> gt is not a match
|
||||||
|
|
||||||
|
precision[0] += matching_words
|
||||||
|
recall[0] += matching_words
|
||||||
|
|
||||||
|
for i in range(len(ref.args)):
|
||||||
|
gold_words = ref.args[i].split()
|
||||||
|
recall[1] += len(gold_words)
|
||||||
|
if len(ex.args) <= i:
|
||||||
|
if i<2:
|
||||||
|
return [0,0] # changed
|
||||||
|
else:
|
||||||
|
continue
|
||||||
|
predicted_words = ex.args[i].split()
|
||||||
|
precision[1] += len(predicted_words)
|
||||||
|
matching_words = 0
|
||||||
|
for w in gold_words:
|
||||||
|
if w in predicted_words:
|
||||||
|
matching_words += 1
|
||||||
|
predicted_words.remove(w)
|
||||||
|
|
||||||
|
precision[0] += matching_words
|
||||||
|
# Currently this slightly penalises systems when the reference
|
||||||
|
# reformulates the sentence words, because the reformulation doesn't
|
||||||
|
# match the predicted word. It's a one-wrong-word penalty to precision,
|
||||||
|
# to all systems that correctly extracted the reformulated word.
|
||||||
|
recall[0] += matching_words
|
||||||
|
|
||||||
|
if(precision[1] == 0):
|
||||||
|
prec = 0
|
||||||
|
else:
|
||||||
|
prec = 1.0 * precision[0] / precision[1]
|
||||||
|
if(recall[1] == 0):
|
||||||
|
rec = 0
|
||||||
|
else:
|
||||||
|
rec = 1.0 * recall[0] / recall[1]
|
||||||
|
return [prec, rec]
|
||||||
|
|
||||||
|
|
||||||
|
@staticmethod
|
||||||
|
def simple_tuple_match(ref, ex, ignoreStopwords, ignoreCase):
|
||||||
|
ref.args = [ref.args[0], ' '.join(ref.args[1:])]
|
||||||
|
ex.args = [ex.args[0], ' '.join(ex.args[1:])]
|
||||||
|
|
||||||
|
precision = [0, 0] # 0 out of 0 predicted words match
|
||||||
|
recall = [0, 0] # 0 out of 0 reference words match
|
||||||
|
# If, for each part, any word is the same as a reference word, then it's a match.
|
||||||
|
|
||||||
|
predicted_words = ex.pred.split()
|
||||||
|
gold_words = ref.pred.split()
|
||||||
|
precision[1] += len(predicted_words)
|
||||||
|
recall[1] += len(gold_words)
|
||||||
|
|
||||||
|
matching_words = 0
|
||||||
|
for w in gold_words:
|
||||||
|
if w in predicted_words:
|
||||||
|
matching_words += 1
|
||||||
|
predicted_words.remove(w)
|
||||||
|
|
||||||
|
precision[0] += matching_words
|
||||||
|
recall[0] += matching_words
|
||||||
|
|
||||||
|
for i in range(len(ref.args)):
|
||||||
|
gold_words = ref.args[i].split()
|
||||||
|
recall[1] += len(gold_words)
|
||||||
|
if len(ex.args) <= i:
|
||||||
|
break
|
||||||
|
predicted_words = ex.args[i].split()
|
||||||
|
precision[1] += len(predicted_words)
|
||||||
|
matching_words = 0
|
||||||
|
for w in gold_words:
|
||||||
|
if w in predicted_words:
|
||||||
|
matching_words += 1
|
||||||
|
predicted_words.remove(w)
|
||||||
|
precision[0] += matching_words
|
||||||
|
|
||||||
|
# Currently this slightly penalises systems when the reference
|
||||||
|
# reformulates the sentence words, because the reformulation doesn't
|
||||||
|
# match the predicted word. It's a one-wrong-word penalty to precision,
|
||||||
|
# to all systems that correctly extracted the reformulated word.
|
||||||
|
recall[0] += matching_words
|
||||||
|
|
||||||
|
prec = 1.0 * precision[0] / precision[1]
|
||||||
|
rec = 1.0 * recall[0] / recall[1]
|
||||||
|
return [prec, rec]
|
||||||
|
|
||||||
|
# @staticmethod
|
||||||
|
# def binary_linient_tuple_match(ref, ex, ignoreStopwords, ignoreCase):
|
||||||
|
# if len(ref.args)>=2:
|
||||||
|
# # r = ref.copy()
|
||||||
|
# r = copy(ref)
|
||||||
|
# r.args = [ref.args[0], ' '.join(ref.args[1:])]
|
||||||
|
# else:
|
||||||
|
# r = ref
|
||||||
|
# if len(ex.args)>=2:
|
||||||
|
# # e = ex.copy()
|
||||||
|
# e = copy(ex)
|
||||||
|
# e.args = [ex.args[0], ' '.join(ex.args[1:])]
|
||||||
|
# else:
|
||||||
|
# e = ex
|
||||||
|
# return Matcher.linient_tuple_match(r, e, ignoreStopwords, ignoreCase)
|
||||||
|
|
||||||
|
@staticmethod
|
||||||
|
def binary_linient_tuple_match(ref, ex, ignoreStopwords, ignoreCase):
|
||||||
|
if len(ref.args)>=2:
|
||||||
|
r = copy(ref)
|
||||||
|
r.args = [ref.args[0], ' '.join(ref.args[1:])]
|
||||||
|
else:
|
||||||
|
r = ref
|
||||||
|
if len(ex.args)>=2:
|
||||||
|
e = copy(ex)
|
||||||
|
e.args = [ex.args[0], ' '.join(ex.args[1:])]
|
||||||
|
else:
|
||||||
|
e = ex
|
||||||
|
stright_match = Matcher.linient_tuple_match(r, e, ignoreStopwords, ignoreCase)
|
||||||
|
|
||||||
|
said_type_reln = ["said", "told", "added", "adds", "says", "adds"]
|
||||||
|
said_type_sentence = False
|
||||||
|
for said_verb in said_type_reln:
|
||||||
|
if said_verb in ref.pred:
|
||||||
|
said_type_sentence = True
|
||||||
|
break
|
||||||
|
if not said_type_sentence:
|
||||||
|
return stright_match
|
||||||
|
else:
|
||||||
|
if len(ex.args)>=2:
|
||||||
|
e = copy(ex)
|
||||||
|
e.args = [' '.join(ex.args[1:]), ex.args[0]]
|
||||||
|
else:
|
||||||
|
e = ex
|
||||||
|
reverse_match = Matcher.linient_tuple_match(r, e, ignoreStopwords, ignoreCase)
|
||||||
|
|
||||||
|
return max(stright_match, reverse_match)
|
||||||
|
|
||||||
|
@staticmethod
|
||||||
|
def binary_tuple_match(ref, ex, ignoreStopwords, ignoreCase):
|
||||||
|
if len(ref.args)>=2:
|
||||||
|
# r = ref.copy()
|
||||||
|
r = copy(ref)
|
||||||
|
r.args = [ref.args[0], ' '.join(ref.args[1:])]
|
||||||
|
else:
|
||||||
|
r = ref
|
||||||
|
if len(ex.args)>=2:
|
||||||
|
# e = ex.copy()
|
||||||
|
e = copy(ex)
|
||||||
|
e.args = [ex.args[0], ' '.join(ex.args[1:])]
|
||||||
|
else:
|
||||||
|
e = ex
|
||||||
|
return Matcher.tuple_match(r, e, ignoreStopwords, ignoreCase)
|
||||||
|
|
||||||
|
@staticmethod
|
||||||
|
def removeStopwords(ls):
|
||||||
|
return [w for w in ls if w.lower() not in Matcher.stopwords]
|
||||||
|
|
||||||
|
# CONSTANTS
|
||||||
|
BLEU_THRESHOLD = 0.4
|
||||||
|
LEXICAL_THRESHOLD = 0.5 # Note: changing this value didn't change the ordering of the tested systems
|
||||||
|
stopwords = stopwords.words('english') + list(string.punctuation)
|
||||||
|
|
45
3-NLP_services/src/Multi2OIE/carb/oieReader.py
Normal file
|
@ -0,0 +1,45 @@
|
||||||
|
class OieReader:
|
||||||
|
|
||||||
|
def read(self, fn, includeNominal):
|
||||||
|
''' should set oie as a class member
|
||||||
|
as a dictionary of extractions by sentence'''
|
||||||
|
raise Exception("Don't run me")
|
||||||
|
|
||||||
|
def count(self):
|
||||||
|
''' number of extractions '''
|
||||||
|
return sum([len(extractions) for _, extractions in self.oie.items()])
|
||||||
|
|
||||||
|
def split_to_corpus(self, corpus_fn, out_fn):
|
||||||
|
"""
|
||||||
|
Given a corpus file name, containing a list of sentences
|
||||||
|
print only the extractions pertaining to it to out_fn in a tab separated format:
|
||||||
|
sent, prob, pred, arg1, arg2, ...
|
||||||
|
"""
|
||||||
|
raw_sents = [line.strip() for line in open(corpus_fn)]
|
||||||
|
with open(out_fn, 'w') as fout:
|
||||||
|
for line in self.get_tabbed().split('\n'):
|
||||||
|
data = line.split('\t')
|
||||||
|
sent = data[0]
|
||||||
|
if sent in raw_sents:
|
||||||
|
fout.write(line + '\n')
|
||||||
|
|
||||||
|
def output_tabbed(self, out_fn):
|
||||||
|
"""
|
||||||
|
Write a tabbed represenation of this corpus.
|
||||||
|
"""
|
||||||
|
with open(out_fn, 'w') as fout:
|
||||||
|
fout.write(self.get_tabbed())
|
||||||
|
|
||||||
|
def get_tabbed(self):
|
||||||
|
"""
|
||||||
|
Get a tabbed format representation of this corpus (assumes that input was
|
||||||
|
already read).
|
||||||
|
"""
|
||||||
|
return "\n".join(['\t'.join(map(str,
|
||||||
|
[ex.sent,
|
||||||
|
ex.confidence,
|
||||||
|
ex.pred,
|
||||||
|
'\t'.join(ex.args)]))
|
||||||
|
for (sent, exs) in self.oie.iteritems()
|
||||||
|
for ex in exs])
|
||||||
|
|
21
3-NLP_services/src/Multi2OIE/carb/oie_readers/argument.py
Normal file
|
@ -0,0 +1,21 @@
|
||||||
|
import nltk
|
||||||
|
from operator import itemgetter
|
||||||
|
|
||||||
|
class Argument:
|
||||||
|
def __init__(self, arg):
|
||||||
|
self.words = [x for x in arg[0].strip().split(' ') if x]
|
||||||
|
self.posTags = map(itemgetter(1), nltk.pos_tag(self.words))
|
||||||
|
self.indices = arg[1]
|
||||||
|
self.feats = {}
|
||||||
|
|
||||||
|
def __str__(self):
|
||||||
|
return "({})".format('\t'.join(map(str,
|
||||||
|
[escape_special_chars(' '.join(self.words)),
|
||||||
|
str(self.indices)])))
|
||||||
|
|
||||||
|
COREF = 'coref'
|
||||||
|
|
||||||
|
## Helper functions
|
||||||
|
def escape_special_chars(s):
|
||||||
|
return s.replace('\t', '\\t')
|
||||||
|
|
|
@ -0,0 +1,55 @@
|
||||||
|
""" Usage:
|
||||||
|
benchmarkGoldReader --in=INPUT_FILE
|
||||||
|
|
||||||
|
Read a tab-formatted file.
|
||||||
|
Each line consists of:
|
||||||
|
sent, prob, pred, arg1, arg2, ...
|
||||||
|
|
||||||
|
"""
|
||||||
|
|
||||||
|
from oie_readers.oieReader import OieReader
|
||||||
|
from oie_readers.extraction import Extraction
|
||||||
|
from docopt import docopt
|
||||||
|
import logging
|
||||||
|
|
||||||
|
logging.basicConfig(level = logging.DEBUG)
|
||||||
|
|
||||||
|
class BenchmarkGoldReader(OieReader):
|
||||||
|
|
||||||
|
def __init__(self):
|
||||||
|
self.name = 'BenchmarkGoldReader'
|
||||||
|
|
||||||
|
def read(self, fn):
|
||||||
|
"""
|
||||||
|
Read a tabbed format line
|
||||||
|
Each line consists of:
|
||||||
|
sent, prob, pred, arg1, arg2, ...
|
||||||
|
"""
|
||||||
|
d = {}
|
||||||
|
ex_index = 0
|
||||||
|
with open(fn) as fin:
|
||||||
|
for line in fin:
|
||||||
|
if not line.strip():
|
||||||
|
continue
|
||||||
|
data = line.strip().split('\t')
|
||||||
|
text, rel = data[:2]
|
||||||
|
curExtraction = Extraction(pred = rel.strip(),
|
||||||
|
head_pred_index = None,
|
||||||
|
sent = text.strip(),
|
||||||
|
confidence = 1.0,
|
||||||
|
question_dist = "./question_distributions/dist_wh_sbj_obj1.json",
|
||||||
|
index = ex_index)
|
||||||
|
ex_index += 1
|
||||||
|
|
||||||
|
for arg in data[2:]:
|
||||||
|
curExtraction.addArg(arg.strip())
|
||||||
|
|
||||||
|
d[text] = d.get(text, []) + [curExtraction]
|
||||||
|
self.oie = d
|
||||||
|
|
||||||
|
|
||||||
|
if __name__ == "__main__":
|
||||||
|
args = docopt(__doc__)
|
||||||
|
input_fn = args["--in"]
|
||||||
|
tr = BenchmarkGoldReader()
|
||||||
|
tr.read(input_fn)
|
|
@ -0,0 +1,90 @@
|
||||||
|
""" Usage:
|
||||||
|
<file-name> --in=INPUT_FILE --out=OUTPUT_FILE [--debug]
|
||||||
|
|
||||||
|
Convert to tabbed format
|
||||||
|
"""
|
||||||
|
# External imports
|
||||||
|
import logging
|
||||||
|
from pprint import pprint
|
||||||
|
from pprint import pformat
|
||||||
|
from docopt import docopt
|
||||||
|
|
||||||
|
# Local imports
|
||||||
|
from oie_readers.oieReader import OieReader
|
||||||
|
from oie_readers.extraction import Extraction
|
||||||
|
import ipdb
|
||||||
|
#=-----
|
||||||
|
|
||||||
|
class ClausieReader(OieReader):
|
||||||
|
|
||||||
|
def __init__(self):
|
||||||
|
self.name = 'ClausIE'
|
||||||
|
|
||||||
|
def read(self, fn):
|
||||||
|
d = {}
|
||||||
|
with open(fn, encoding="utf-8") as fin:
|
||||||
|
for line in fin:
|
||||||
|
data = line.strip().split('\t')
|
||||||
|
if len(data) == 1:
|
||||||
|
text = data[0]
|
||||||
|
elif len(data) == 5:
|
||||||
|
arg1, rel, arg2 = [s[1:-1] for s in data[1:4]]
|
||||||
|
confidence = data[4]
|
||||||
|
|
||||||
|
curExtraction = Extraction(pred = rel,
|
||||||
|
head_pred_index = -1,
|
||||||
|
sent = text,
|
||||||
|
confidence = float(confidence))
|
||||||
|
|
||||||
|
curExtraction.addArg(arg1)
|
||||||
|
curExtraction.addArg(arg2)
|
||||||
|
d[text] = d.get(text, []) + [curExtraction]
|
||||||
|
self.oie = d
|
||||||
|
# self.normalizeConfidence()
|
||||||
|
|
||||||
|
# # remove exxtractions below the confidence threshold
|
||||||
|
# if type(self.threshold) != type(None):
|
||||||
|
# new_d = {}
|
||||||
|
# for sent in self.oie:
|
||||||
|
# for extraction in self.oie[sent]:
|
||||||
|
# if extraction.confidence < self.threshold:
|
||||||
|
# continue
|
||||||
|
# else:
|
||||||
|
# new_d[sent] = new_d.get(sent, []) + [extraction]
|
||||||
|
# self.oie = new_d
|
||||||
|
|
||||||
|
|
||||||
|
|
||||||
|
def normalizeConfidence(self):
|
||||||
|
''' Normalize confidence to resemble probabilities '''
|
||||||
|
EPSILON = 1e-3
|
||||||
|
|
||||||
|
confidences = [extraction.confidence for sent in self.oie for extraction in self.oie[sent]]
|
||||||
|
maxConfidence = max(confidences)
|
||||||
|
minConfidence = min(confidences)
|
||||||
|
|
||||||
|
denom = maxConfidence - minConfidence + (2*EPSILON)
|
||||||
|
|
||||||
|
for sent, extractions in self.oie.items():
|
||||||
|
for extraction in extractions:
|
||||||
|
extraction.confidence = ( (extraction.confidence - minConfidence) + EPSILON) / denom
|
||||||
|
|
||||||
|
|
||||||
|
|
||||||
|
if __name__ == "__main__":
|
||||||
|
# Parse command line arguments
|
||||||
|
args = docopt(__doc__)
|
||||||
|
inp_fn = args["--in"]
|
||||||
|
out_fn = args["--out"]
|
||||||
|
debug = args["--debug"]
|
||||||
|
if debug:
|
||||||
|
logging.basicConfig(level = logging.DEBUG)
|
||||||
|
else:
|
||||||
|
logging.basicConfig(level = logging.INFO)
|
||||||
|
|
||||||
|
|
||||||
|
oie = ClausieReader()
|
||||||
|
oie.read(inp_fn)
|
||||||
|
oie.output_tabbed(out_fn)
|
||||||
|
|
||||||
|
logging.info("DONE")
|
444
3-NLP_services/src/Multi2OIE/carb/oie_readers/extraction.py
Normal file
|
@ -0,0 +1,444 @@
|
||||||
|
from sklearn.preprocessing.data import binarize
|
||||||
|
from oie_readers.argument import Argument
|
||||||
|
from operator import itemgetter
|
||||||
|
from collections import defaultdict
|
||||||
|
import nltk
|
||||||
|
import itertools
|
||||||
|
import logging
|
||||||
|
import numpy as np
|
||||||
|
import pdb
|
||||||
|
|
||||||
|
class Extraction:
|
||||||
|
"""
|
||||||
|
Stores sentence, single predicate and corresponding arguments.
|
||||||
|
"""
|
||||||
|
def __init__(self, pred, head_pred_index, sent, confidence, question_dist = '', index = -1):
|
||||||
|
self.pred = pred
|
||||||
|
self.head_pred_index = head_pred_index
|
||||||
|
self.sent = sent
|
||||||
|
self.args = []
|
||||||
|
self.confidence = confidence
|
||||||
|
self.matched = []
|
||||||
|
self.questions = {}
|
||||||
|
self.indsForQuestions = defaultdict(lambda: set())
|
||||||
|
self.is_mwp = False
|
||||||
|
self.question_dist = question_dist
|
||||||
|
self.index = index
|
||||||
|
|
||||||
|
def distArgFromPred(self, arg):
|
||||||
|
assert(len(self.pred) == 2)
|
||||||
|
dists = []
|
||||||
|
for x in self.pred[1]:
|
||||||
|
for y in arg.indices:
|
||||||
|
dists.append(abs(x - y))
|
||||||
|
|
||||||
|
return min(dists)
|
||||||
|
|
||||||
|
def argsByDistFromPred(self, question):
|
||||||
|
return sorted(self.questions[question], key = lambda arg: self.distArgFromPred(arg))
|
||||||
|
|
||||||
|
def addArg(self, arg, question = None):
|
||||||
|
self.args.append(arg)
|
||||||
|
if question:
|
||||||
|
self.questions[question] = self.questions.get(question,[]) + [Argument(arg)]
|
||||||
|
|
||||||
|
def noPronounArgs(self):
|
||||||
|
"""
|
||||||
|
Returns True iff all of this extraction's arguments are not pronouns.
|
||||||
|
"""
|
||||||
|
for (a, _) in self.args:
|
||||||
|
tokenized_arg = nltk.word_tokenize(a)
|
||||||
|
if len(tokenized_arg) == 1:
|
||||||
|
_, pos_tag = nltk.pos_tag(tokenized_arg)[0]
|
||||||
|
if ('PRP' in pos_tag):
|
||||||
|
return False
|
||||||
|
return True
|
||||||
|
|
||||||
|
def isContiguous(self):
|
||||||
|
return all([indices for (_, indices) in self.args])
|
||||||
|
|
||||||
|
def toBinary(self):
|
||||||
|
''' Try to represent this extraction's arguments as binary
|
||||||
|
If fails, this function will return an empty list. '''
|
||||||
|
|
||||||
|
ret = [self.elementToStr(self.pred)]
|
||||||
|
|
||||||
|
if len(self.args) == 2:
|
||||||
|
# we're in luck
|
||||||
|
return ret + [self.elementToStr(arg) for arg in self.args]
|
||||||
|
|
||||||
|
return []
|
||||||
|
|
||||||
|
if not self.isContiguous():
|
||||||
|
# give up on non contiguous arguments (as we need indexes)
|
||||||
|
return []
|
||||||
|
|
||||||
|
# otherwise, try to merge based on indices
|
||||||
|
# TODO: you can explore other methods for doing this
|
||||||
|
binarized = self.binarizeByIndex()
|
||||||
|
|
||||||
|
if binarized:
|
||||||
|
return ret + binarized
|
||||||
|
|
||||||
|
return []
|
||||||
|
|
||||||
|
|
||||||
|
def elementToStr(self, elem, print_indices = True):
|
||||||
|
''' formats an extraction element (pred or arg) as a raw string
|
||||||
|
removes indices and trailing spaces '''
|
||||||
|
if print_indices:
|
||||||
|
return str(elem)
|
||||||
|
if isinstance(elem, str):
|
||||||
|
return elem
|
||||||
|
if isinstance(elem, tuple):
|
||||||
|
ret = elem[0].rstrip().lstrip()
|
||||||
|
else:
|
||||||
|
ret = ' '.join(elem.words)
|
||||||
|
assert ret, "empty element? {0}".format(elem)
|
||||||
|
return ret
|
||||||
|
|
||||||
|
def binarizeByIndex(self):
|
||||||
|
extraction = [self.pred] + self.args
|
||||||
|
markPred = [(w, ind, i == 0) for i, (w, ind) in enumerate(extraction)]
|
||||||
|
sortedExtraction = sorted(markPred, key = lambda ws, indices, f : indices[0])
|
||||||
|
s = ' '.join(['{1} {0} {1}'.format(self.elementToStr(elem), SEP) if elem[2] else self.elementToStr(elem) for elem in sortedExtraction])
|
||||||
|
binArgs = [a for a in s.split(SEP) if a.rstrip().lstrip()]
|
||||||
|
|
||||||
|
if len(binArgs) == 2:
|
||||||
|
return binArgs
|
||||||
|
|
||||||
|
# failure
|
||||||
|
return []
|
||||||
|
|
||||||
|
def bow(self):
|
||||||
|
return ' '.join([self.elementToStr(elem) for elem in [self.pred] + self.args])
|
||||||
|
|
||||||
|
def getSortedArgs(self):
|
||||||
|
"""
|
||||||
|
Sort the list of arguments.
|
||||||
|
If a question distribution is provided - use it,
|
||||||
|
otherwise, default to the order of appearance in the sentence.
|
||||||
|
"""
|
||||||
|
if self.question_dist:
|
||||||
|
# There's a question distribtuion - use it
|
||||||
|
return self.sort_args_by_distribution()
|
||||||
|
ls = []
|
||||||
|
for q, args in self.questions.iteritems():
|
||||||
|
if (len(args) != 1):
|
||||||
|
logging.debug("Not one argument: {}".format(args))
|
||||||
|
continue
|
||||||
|
arg = args[0]
|
||||||
|
indices = list(self.indsForQuestions[q].union(arg.indices))
|
||||||
|
if not indices:
|
||||||
|
logging.debug("Empty indexes for arg {} -- backing to zero".format(arg))
|
||||||
|
indices = [0]
|
||||||
|
ls.append(((arg, q), indices))
|
||||||
|
return [a for a, _ in sorted(ls,
|
||||||
|
key = lambda _, indices: min(indices))]
|
||||||
|
|
||||||
|
def question_prob_for_loc(self, question, loc):
|
||||||
|
"""
|
||||||
|
Returns the probability of the given question leading to argument
|
||||||
|
appearing in the given location in the output slot.
|
||||||
|
"""
|
||||||
|
gen_question = generalize_question(question)
|
||||||
|
q_dist = self.question_dist[gen_question]
|
||||||
|
logging.debug("distribution of {}: {}".format(gen_question,
|
||||||
|
q_dist))
|
||||||
|
|
||||||
|
return float(q_dist.get(loc, 0)) / \
|
||||||
|
sum(q_dist.values())
|
||||||
|
|
||||||
|
def sort_args_by_distribution(self):
|
||||||
|
"""
|
||||||
|
Use this instance's question distribution (this func assumes it exists)
|
||||||
|
in determining the positioning of the arguments.
|
||||||
|
Greedy algorithm:
|
||||||
|
0. Decide on which argument will serve as the ``subject'' (first slot) of this extraction
|
||||||
|
0.1 Based on the most probable one for this spot
|
||||||
|
(special care is given to select the highly-influential subject position)
|
||||||
|
1. For all other arguments, sort arguments by the prevalance of their questions
|
||||||
|
2. For each argument:
|
||||||
|
2.1 Assign to it the most probable slot still available
|
||||||
|
2.2 If non such exist (fallback) - default to put it in the last location
|
||||||
|
"""
|
||||||
|
INF_LOC = 100 # Used as an impractical last argument
|
||||||
|
|
||||||
|
# Store arguments by slot
|
||||||
|
ret = {INF_LOC: []}
|
||||||
|
logging.debug("sorting: {}".format(self.questions))
|
||||||
|
|
||||||
|
# Find the most suitable arguemnt for the subject location
|
||||||
|
logging.debug("probs for subject: {}".format([(q, self.question_prob_for_loc(q, 0))
|
||||||
|
for (q, _) in self.questions.iteritems()]))
|
||||||
|
|
||||||
|
subj_question, subj_args = max(self.questions.iteritems(),
|
||||||
|
key = lambda q, _: self.question_prob_for_loc(q, 0))
|
||||||
|
|
||||||
|
ret[0] = [(subj_args[0], subj_question)]
|
||||||
|
|
||||||
|
# Find the rest
|
||||||
|
for (question, args) in sorted([(q, a)
|
||||||
|
for (q, a) in self.questions.iteritems() if (q not in [subj_question])],
|
||||||
|
key = lambda q, _: \
|
||||||
|
sum(self.question_dist[generalize_question(q)].values()),
|
||||||
|
reverse = True):
|
||||||
|
gen_question = generalize_question(question)
|
||||||
|
arg = args[0]
|
||||||
|
assigned_flag = False
|
||||||
|
for (loc, count) in sorted(self.question_dist[gen_question].iteritems(),
|
||||||
|
key = lambda _ , c: c,
|
||||||
|
reverse = True):
|
||||||
|
if loc not in ret:
|
||||||
|
# Found an empty slot for this item
|
||||||
|
# Place it there and break out
|
||||||
|
ret[loc] = [(arg, question)]
|
||||||
|
assigned_flag = True
|
||||||
|
break
|
||||||
|
|
||||||
|
if not assigned_flag:
|
||||||
|
# Add this argument to the non-assigned (hopefully doesn't happen much)
|
||||||
|
logging.debug("Couldn't find an open assignment for {}".format((arg, gen_question)))
|
||||||
|
ret[INF_LOC].append((arg, question))
|
||||||
|
|
||||||
|
logging.debug("Linearizing arg list: {}".format(ret))
|
||||||
|
|
||||||
|
# Finished iterating - consolidate and return a list of arguments
|
||||||
|
return [arg
|
||||||
|
for (_, arg_ls) in sorted(ret.iteritems(),
|
||||||
|
key = lambda k, v: int(k))
|
||||||
|
for arg in arg_ls]
|
||||||
|
|
||||||
|
|
||||||
|
def __str__(self):
|
||||||
|
pred_str = self.elementToStr(self.pred)
|
||||||
|
return '{}\t{}\t{}'.format(self.get_base_verb(pred_str),
|
||||||
|
self.compute_global_pred(pred_str,
|
||||||
|
self.questions.keys()),
|
||||||
|
'\t'.join([escape_special_chars(self.augment_arg_with_question(self.elementToStr(arg),
|
||||||
|
question))
|
||||||
|
for arg, question in self.getSortedArgs()]))
|
||||||
|
|
||||||
|
def get_base_verb(self, surface_pred):
|
||||||
|
"""
|
||||||
|
Given the surface pred, return the original annotated verb
|
||||||
|
"""
|
||||||
|
# Assumes that at this point the verb is always the last word
|
||||||
|
# in the surface predicate
|
||||||
|
return surface_pred.split(' ')[-1]
|
||||||
|
|
||||||
|
|
||||||
|
def compute_global_pred(self, surface_pred, questions):
|
||||||
|
"""
|
||||||
|
Given the surface pred and all instansiations of questions,
|
||||||
|
make global coherence decisions regarding the final form of the predicate
|
||||||
|
This should hopefully take care of multi word predicates and correct inflections
|
||||||
|
"""
|
||||||
|
from operator import itemgetter
|
||||||
|
split_surface = surface_pred.split(' ')
|
||||||
|
|
||||||
|
if len(split_surface) > 1:
|
||||||
|
# This predicate has a modal preceding the base verb
|
||||||
|
verb = split_surface[-1]
|
||||||
|
ret = split_surface[:-1] # get all of the elements in the modal
|
||||||
|
else:
|
||||||
|
verb = split_surface[0]
|
||||||
|
ret = []
|
||||||
|
|
||||||
|
split_questions = map(lambda question: question.split(' '),
|
||||||
|
questions)
|
||||||
|
|
||||||
|
preds = map(normalize_element,
|
||||||
|
map(itemgetter(QUESTION_TRG_INDEX),
|
||||||
|
split_questions))
|
||||||
|
if len(set(preds)) > 1:
|
||||||
|
# This predicate is appears in multiple ways, let's stick to the base form
|
||||||
|
ret.append(verb)
|
||||||
|
|
||||||
|
if len(set(preds)) == 1:
|
||||||
|
# Change the predciate to the inflected form
|
||||||
|
# if there's exactly one way in which the predicate is conveyed
|
||||||
|
ret.append(preds[0])
|
||||||
|
|
||||||
|
pps = map(normalize_element,
|
||||||
|
map(itemgetter(QUESTION_PP_INDEX),
|
||||||
|
split_questions))
|
||||||
|
|
||||||
|
obj2s = map(normalize_element,
|
||||||
|
map(itemgetter(QUESTION_OBJ2_INDEX),
|
||||||
|
split_questions))
|
||||||
|
|
||||||
|
if (len(set(pps)) == 1):
|
||||||
|
# If all questions for the predicate include the same pp attachemnt -
|
||||||
|
# assume it's a multiword predicate
|
||||||
|
self.is_mwp = True # Signal to arguments that they shouldn't take the preposition
|
||||||
|
ret.append(pps[0])
|
||||||
|
|
||||||
|
# Concat all elements in the predicate and return
|
||||||
|
return " ".join(ret).strip()
|
||||||
|
|
||||||
|
|
||||||
|
def augment_arg_with_question(self, arg, question):
|
||||||
|
"""
|
||||||
|
Decide what elements from the question to incorporate in the given
|
||||||
|
corresponding argument
|
||||||
|
"""
|
||||||
|
# Parse question
|
||||||
|
wh, aux, sbj, trg, obj1, pp, obj2 = map(normalize_element,
|
||||||
|
question.split(' ')[:-1]) # Last split is the question mark
|
||||||
|
|
||||||
|
# Place preposition in argument
|
||||||
|
# This is safer when dealing with n-ary arguments, as it's directly attaches to the
|
||||||
|
# appropriate argument
|
||||||
|
if (not self.is_mwp) and pp and (not obj2):
|
||||||
|
if not(arg.startswith("{} ".format(pp))):
|
||||||
|
# Avoid repeating the preporition in cases where both question and answer contain it
|
||||||
|
return " ".join([pp,
|
||||||
|
arg])
|
||||||
|
|
||||||
|
# Normal cases
|
||||||
|
return arg
|
||||||
|
|
||||||
|
def clusterScore(self, cluster):
|
||||||
|
"""
|
||||||
|
Calculate cluster density score as the mean distance of the maximum distance of each slot.
|
||||||
|
Lower score represents a denser cluster.
|
||||||
|
"""
|
||||||
|
logging.debug("*-*-*- Cluster: {}".format(cluster))
|
||||||
|
|
||||||
|
# Find global centroid
|
||||||
|
arr = np.array([x for ls in cluster for x in ls])
|
||||||
|
centroid = np.sum(arr)/arr.shape[0]
|
||||||
|
logging.debug("Centroid: {}".format(centroid))
|
||||||
|
|
||||||
|
# Calculate mean over all maxmimum points
|
||||||
|
return np.average([max([abs(x - centroid) for x in ls]) for ls in cluster])
|
||||||
|
|
||||||
|
def resolveAmbiguity(self):
|
||||||
|
"""
|
||||||
|
Heursitic to map the elments (argument and predicates) of this extraction
|
||||||
|
back to the indices of the sentence.
|
||||||
|
"""
|
||||||
|
## TODO: This removes arguments for which there was no consecutive span found
|
||||||
|
## Part of these are non-consecutive arguments,
|
||||||
|
## but other could be a bug in recognizing some punctuation marks
|
||||||
|
|
||||||
|
elements = [self.pred] \
|
||||||
|
+ [(s, indices)
|
||||||
|
for (s, indices)
|
||||||
|
in self.args
|
||||||
|
if indices]
|
||||||
|
logging.debug("Resolving ambiguity in: {}".format(elements))
|
||||||
|
|
||||||
|
# Collect all possible combinations of arguments and predicate indices
|
||||||
|
# (hopefully it's not too much)
|
||||||
|
all_combinations = list(itertools.product(*map(itemgetter(1), elements)))
|
||||||
|
logging.debug("Number of combinations: {}".format(len(all_combinations)))
|
||||||
|
|
||||||
|
# Choose the ones with best clustering and unfold them
|
||||||
|
resolved_elements = zip(map(itemgetter(0), elements),
|
||||||
|
min(all_combinations,
|
||||||
|
key = lambda cluster: self.clusterScore(cluster)))
|
||||||
|
logging.debug("Resolved elements = {}".format(resolved_elements))
|
||||||
|
|
||||||
|
self.pred = resolved_elements[0]
|
||||||
|
self.args = resolved_elements[1:]
|
||||||
|
|
||||||
|
def conll(self, external_feats = {}):
|
||||||
|
"""
|
||||||
|
Return a CoNLL string representation of this extraction
|
||||||
|
"""
|
||||||
|
return '\n'.join(["\t".join(map(str,
|
||||||
|
[i, w] + \
|
||||||
|
list(self.pred) + \
|
||||||
|
[self.head_pred_index] + \
|
||||||
|
external_feats + \
|
||||||
|
[self.get_label(i)]))
|
||||||
|
for (i, w)
|
||||||
|
in enumerate(self.sent.split(" "))]) + '\n'
|
||||||
|
|
||||||
|
def get_label(self, index):
|
||||||
|
"""
|
||||||
|
Given an index of a word in the sentence -- returns the appropriate BIO conll label
|
||||||
|
Assumes that ambiguation was already resolved.
|
||||||
|
"""
|
||||||
|
# Get the element(s) in which this index appears
|
||||||
|
ent = [(elem_ind, elem)
|
||||||
|
for (elem_ind, elem)
|
||||||
|
in enumerate(map(itemgetter(1),
|
||||||
|
[self.pred] + self.args))
|
||||||
|
if index in elem]
|
||||||
|
|
||||||
|
if not ent:
|
||||||
|
# index doesnt appear in any element
|
||||||
|
return "O"
|
||||||
|
|
||||||
|
if len(ent) > 1:
|
||||||
|
# The same word appears in two different answers
|
||||||
|
# In this case we choose the first one as label
|
||||||
|
logging.warn("Index {} appears in one than more element: {}".\
|
||||||
|
format(index,
|
||||||
|
"\t".join(map(str,
|
||||||
|
[ent,
|
||||||
|
self.sent,
|
||||||
|
self.pred,
|
||||||
|
self.args]))))
|
||||||
|
|
||||||
|
## Some indices appear in more than one argument (ones where the above message appears)
|
||||||
|
## From empricial observation, these seem to mostly consist of different levels of granularity:
|
||||||
|
## what had _ been taken _ _ _ ? loan commitments topping $ 3 billion
|
||||||
|
## how much had _ been taken _ _ _ ? topping $ 3 billion
|
||||||
|
## In these cases we heuristically choose the shorter answer span, hopefully creating minimal spans
|
||||||
|
## E.g., in this example two arguemnts are created: (loan commitments, topping $ 3 billion)
|
||||||
|
|
||||||
|
elem_ind, elem = min(ent, key = lambda _, ls: len(ls))
|
||||||
|
|
||||||
|
# Distinguish between predicate and arguments
|
||||||
|
prefix = "P" if elem_ind == 0 else "A{}".format(elem_ind - 1)
|
||||||
|
|
||||||
|
# Distinguish between Beginning and Inside labels
|
||||||
|
suffix = "B" if index == elem[0] else "I"
|
||||||
|
|
||||||
|
return "{}-{}".format(prefix, suffix)
|
||||||
|
|
||||||
|
def __str__(self):
|
||||||
|
return '{0}\t{1}'.format(self.elementToStr(self.pred,
|
||||||
|
print_indices = True),
|
||||||
|
'\t'.join([self.elementToStr(arg)
|
||||||
|
for arg
|
||||||
|
in self.args]))
|
||||||
|
|
||||||
|
# Flatten a list of lists
|
||||||
|
flatten = lambda l: [item for sublist in l for item in sublist]
|
||||||
|
|
||||||
|
|
||||||
|
def normalize_element(elem):
|
||||||
|
"""
|
||||||
|
Return a surface form of the given question element.
|
||||||
|
the output should be properly able to precede a predicate (or blank otherwise)
|
||||||
|
"""
|
||||||
|
return elem.replace("_", " ") \
|
||||||
|
if (elem != "_")\
|
||||||
|
else ""
|
||||||
|
|
||||||
|
## Helper functions
|
||||||
|
def escape_special_chars(s):
|
||||||
|
return s.replace('\t', '\\t')
|
||||||
|
|
||||||
|
|
||||||
|
def generalize_question(question):
|
||||||
|
"""
|
||||||
|
Given a question in the context of the sentence and the predicate index within
|
||||||
|
the question - return a generalized version which extracts only order-imposing features
|
||||||
|
"""
|
||||||
|
import nltk # Using nltk since couldn't get spaCy to agree on the tokenization
|
||||||
|
wh, aux, sbj, trg, obj1, pp, obj2 = question.split(' ')[:-1] # Last split is the question mark
|
||||||
|
return ' '.join([wh, sbj, obj1])
|
||||||
|
|
||||||
|
|
||||||
|
|
||||||
|
## CONSTANTS
|
||||||
|
SEP = ';;;'
|
||||||
|
QUESTION_TRG_INDEX = 3 # index of the predicate within the question
|
||||||
|
QUESTION_PP_INDEX = 5
|
||||||
|
QUESTION_OBJ2_INDEX = 6
|
44
3-NLP_services/src/Multi2OIE/carb/oie_readers/goldReader.py
Normal file
|
@ -0,0 +1,44 @@
|
||||||
|
from oie_readers.oieReader import OieReader
|
||||||
|
from oie_readers.extraction import Extraction
|
||||||
|
from _collections import defaultdict
|
||||||
|
import ipdb
|
||||||
|
|
||||||
|
class GoldReader(OieReader):
|
||||||
|
|
||||||
|
# Path relative to repo root folder
|
||||||
|
default_filename = './oie_corpus/all.oie'
|
||||||
|
|
||||||
|
def __init__(self):
|
||||||
|
self.name = 'Gold'
|
||||||
|
|
||||||
|
def read(self, fn):
|
||||||
|
d = defaultdict(lambda: [])
|
||||||
|
with open(fn) as fin:
|
||||||
|
for line_ind, line in enumerate(fin):
|
||||||
|
# print line
|
||||||
|
data = line.strip().split('\t')
|
||||||
|
text, rel = data[:2]
|
||||||
|
args = data[2:]
|
||||||
|
confidence = 1
|
||||||
|
|
||||||
|
curExtraction = Extraction(pred = rel.strip(),
|
||||||
|
head_pred_index = None,
|
||||||
|
sent = text.strip(),
|
||||||
|
confidence = float(confidence),
|
||||||
|
index = line_ind)
|
||||||
|
for arg in args:
|
||||||
|
if "C: " in arg:
|
||||||
|
continue
|
||||||
|
curExtraction.addArg(arg.strip())
|
||||||
|
|
||||||
|
d[text.strip()].append(curExtraction)
|
||||||
|
self.oie = d
|
||||||
|
|
||||||
|
|
||||||
|
if __name__ == '__main__' :
|
||||||
|
g = GoldReader()
|
||||||
|
g.read('../oie_corpus/all.oie', includeNominal = False)
|
||||||
|
d = g.oie
|
||||||
|
e = d.items()[0]
|
||||||
|
print(e[1][0].bow())
|
||||||
|
print(g.count())
|
45
3-NLP_services/src/Multi2OIE/carb/oie_readers/oieReader.py
Normal file
|
@ -0,0 +1,45 @@
|
||||||
|
class OieReader:
|
||||||
|
|
||||||
|
def read(self, fn, includeNominal):
|
||||||
|
''' should set oie as a class member
|
||||||
|
as a dictionary of extractions by sentence'''
|
||||||
|
raise Exception("Don't run me")
|
||||||
|
|
||||||
|
def count(self):
|
||||||
|
''' number of extractions '''
|
||||||
|
return sum([len(extractions) for _, extractions in self.oie.items()])
|
||||||
|
|
||||||
|
def split_to_corpus(self, corpus_fn, out_fn):
|
||||||
|
"""
|
||||||
|
Given a corpus file name, containing a list of sentences
|
||||||
|
print only the extractions pertaining to it to out_fn in a tab separated format:
|
||||||
|
sent, prob, pred, arg1, arg2, ...
|
||||||
|
"""
|
||||||
|
raw_sents = [line.strip() for line in open(corpus_fn)]
|
||||||
|
with open(out_fn, 'w') as fout:
|
||||||
|
for line in self.get_tabbed().split('\n'):
|
||||||
|
data = line.split('\t')
|
||||||
|
sent = data[0]
|
||||||
|
if sent in raw_sents:
|
||||||
|
fout.write(line + '\n')
|
||||||
|
|
||||||
|
def output_tabbed(self, out_fn):
|
||||||
|
"""
|
||||||
|
Write a tabbed represenation of this corpus.
|
||||||
|
"""
|
||||||
|
with open(out_fn, 'w') as fout:
|
||||||
|
fout.write(self.get_tabbed())
|
||||||
|
|
||||||
|
def get_tabbed(self):
|
||||||
|
"""
|
||||||
|
Get a tabbed format representation of this corpus (assumes that input was
|
||||||
|
already read).
|
||||||
|
"""
|
||||||
|
return "\n".join(['\t'.join(map(str,
|
||||||
|
[ex.sent,
|
||||||
|
ex.confidence,
|
||||||
|
ex.pred,
|
||||||
|
'\t'.join(ex.args)]))
|
||||||
|
for (sent, exs) in self.oie.iteritems()
|
||||||
|
for ex in exs])
|
||||||
|
|
22
3-NLP_services/src/Multi2OIE/carb/oie_readers/ollieReader.py
Normal file
|
@ -0,0 +1,22 @@
|
||||||
|
from oie_readers.oieReader import OieReader
|
||||||
|
from oie_readers.extraction import Extraction
|
||||||
|
|
||||||
|
class OllieReader(OieReader):
|
||||||
|
|
||||||
|
def __init__(self):
|
||||||
|
self.name = 'OLLIE'
|
||||||
|
|
||||||
|
def read(self, fn):
|
||||||
|
d = {}
|
||||||
|
with open(fn) as fin:
|
||||||
|
fin.readline() #remove header
|
||||||
|
for line in fin:
|
||||||
|
data = line.strip().split('\t')
|
||||||
|
confidence, arg1, rel, arg2, enabler, attribution, text = data[:7]
|
||||||
|
curExtraction = Extraction(pred = rel, head_pred_index = -1, sent = text, confidence = float(confidence))
|
||||||
|
curExtraction.addArg(arg1)
|
||||||
|
curExtraction.addArg(arg2)
|
||||||
|
d[text] = d.get(text, []) + [curExtraction]
|
||||||
|
self.oie = d
|
||||||
|
|
||||||
|
|
|
@ -0,0 +1,38 @@
|
||||||
|
from oie_readers.oieReader import OieReader
|
||||||
|
from oie_readers.extraction import Extraction
|
||||||
|
|
||||||
|
class OpenieFiveReader(OieReader):
|
||||||
|
|
||||||
|
def __init__(self):
|
||||||
|
self.name = 'OpenIE-5'
|
||||||
|
|
||||||
|
def read(self, fn):
|
||||||
|
d = {}
|
||||||
|
with open(fn) as fin:
|
||||||
|
for line in fin:
|
||||||
|
data = line.strip().split('\t')
|
||||||
|
confidence = data[0]
|
||||||
|
|
||||||
|
if not all(data[2:5]):
|
||||||
|
continue
|
||||||
|
arg1, rel = [s[s.index('(') + 1:s.index(',List(')] for s in data[2:4]]
|
||||||
|
#args = data[4].strip().split(');')
|
||||||
|
#print arg2s
|
||||||
|
args = [s[s.index('(') + 1:s.index(',List(')] for s in data[4].strip().split(');')]
|
||||||
|
# if arg1 == "the younger La Flesche":
|
||||||
|
# print len(args)
|
||||||
|
text = data[5]
|
||||||
|
if data[1]:
|
||||||
|
#print arg1, rel
|
||||||
|
s = data[1]
|
||||||
|
if not (arg1 + ' ' + rel).startswith(s[s.index('(') + 1:s.index(',List(')]):
|
||||||
|
#print "##########Not adding context"
|
||||||
|
arg1 = s[s.index('(') + 1:s.index(',List(')] + ' ' + arg1
|
||||||
|
#print arg1 + rel, ",,,,, ", s[s.index('(') + 1:s.index(',List(')]
|
||||||
|
#curExtraction = Extraction(pred = rel, sent = text, confidence = float(confidence))
|
||||||
|
curExtraction = Extraction(pred = rel, head_pred_index = -1, sent = text, confidence = float(confidence))
|
||||||
|
curExtraction.addArg(arg1)
|
||||||
|
for arg in args:
|
||||||
|
curExtraction.addArg(arg)
|
||||||
|
d[text] = d.get(text, []) + [curExtraction]
|
||||||
|
self.oie = d
|
|
@ -0,0 +1,59 @@
|
||||||
|
""" Usage:
|
||||||
|
<file-name> --in=INPUT_FILE --out=OUTPUT_FILE [--debug]
|
||||||
|
|
||||||
|
Convert to tabbed format
|
||||||
|
"""
|
||||||
|
# External imports
|
||||||
|
import logging
|
||||||
|
from pprint import pprint
|
||||||
|
from pprint import pformat
|
||||||
|
from docopt import docopt
|
||||||
|
|
||||||
|
# Local imports
|
||||||
|
from oie_readers.oieReader import OieReader
|
||||||
|
from oie_readers.extraction import Extraction
|
||||||
|
import ipdb
|
||||||
|
|
||||||
|
#=-----
|
||||||
|
|
||||||
|
class OpenieFourReader(OieReader):
|
||||||
|
|
||||||
|
def __init__(self):
|
||||||
|
self.name = 'OpenIE-4'
|
||||||
|
|
||||||
|
def read(self, fn):
|
||||||
|
d = {}
|
||||||
|
with open(fn) as fin:
|
||||||
|
for line in fin:
|
||||||
|
data = line.strip().split('\t')
|
||||||
|
confidence = data[0]
|
||||||
|
if not all(data[2:5]):
|
||||||
|
logging.debug("Skipped line: {}".format(line))
|
||||||
|
continue
|
||||||
|
arg1, rel, arg2 = [s[s.index('(') + 1:s.index(',List(')] for s in data[2:5]]
|
||||||
|
text = data[5]
|
||||||
|
curExtraction = Extraction(pred = rel, head_pred_index = -1, sent = text, confidence = float(confidence))
|
||||||
|
curExtraction.addArg(arg1)
|
||||||
|
curExtraction.addArg(arg2)
|
||||||
|
d[text] = d.get(text, []) + [curExtraction]
|
||||||
|
self.oie = d
|
||||||
|
|
||||||
|
|
||||||
|
|
||||||
|
if __name__ == "__main__":
|
||||||
|
# Parse command line arguments
|
||||||
|
args = docopt(__doc__)
|
||||||
|
inp_fn = args["--in"]
|
||||||
|
out_fn = args["--out"]
|
||||||
|
debug = args["--debug"]
|
||||||
|
if debug:
|
||||||
|
logging.basicConfig(level = logging.DEBUG)
|
||||||
|
else:
|
||||||
|
logging.basicConfig(level = logging.INFO)
|
||||||
|
|
||||||
|
|
||||||
|
oie = OpenieFourReader()
|
||||||
|
oie.read(inp_fn)
|
||||||
|
oie.output_tabbed(out_fn)
|
||||||
|
|
||||||
|
logging.info("DONE")
|
44
3-NLP_services/src/Multi2OIE/carb/oie_readers/propsReader.py
Normal file
|
@ -0,0 +1,44 @@
|
||||||
|
from oie_readers.oieReader import OieReader
|
||||||
|
from oie_readers.extraction import Extraction
|
||||||
|
|
||||||
|
|
||||||
|
class PropSReader(OieReader):
|
||||||
|
|
||||||
|
def __init__(self):
|
||||||
|
self.name = 'PropS'
|
||||||
|
|
||||||
|
def read(self, fn):
|
||||||
|
d = {}
|
||||||
|
with open(fn) as fin:
|
||||||
|
for line in fin:
|
||||||
|
if not line.strip():
|
||||||
|
continue
|
||||||
|
data = line.strip().split('\t')
|
||||||
|
confidence, text, rel = data[:3]
|
||||||
|
curExtraction = Extraction(pred = rel, sent = text, confidence = float(confidence), head_pred_index=-1)
|
||||||
|
|
||||||
|
for arg in data[4::2]:
|
||||||
|
curExtraction.addArg(arg)
|
||||||
|
|
||||||
|
d[text] = d.get(text, []) + [curExtraction]
|
||||||
|
self.oie = d
|
||||||
|
# self.normalizeConfidence()
|
||||||
|
|
||||||
|
|
||||||
|
def normalizeConfidence(self):
|
||||||
|
''' Normalize confidence to resemble probabilities '''
|
||||||
|
EPSILON = 1e-3
|
||||||
|
|
||||||
|
self.confidences = [extraction.confidence for sent in self.oie for extraction in self.oie[sent]]
|
||||||
|
maxConfidence = max(self.confidences)
|
||||||
|
minConfidence = min(self.confidences)
|
||||||
|
|
||||||
|
denom = maxConfidence - minConfidence + (2*EPSILON)
|
||||||
|
|
||||||
|
for sent, extractions in self.oie.items():
|
||||||
|
for extraction in extractions:
|
||||||
|
extraction.confidence = ( (extraction.confidence - minConfidence) + EPSILON) / denom
|
||||||
|
|
||||||
|
|
||||||
|
|
||||||
|
|
|
@ -0,0 +1,29 @@
|
||||||
|
from oie_readers.oieReader import OieReader
|
||||||
|
from oie_readers.extraction import Extraction
|
||||||
|
|
||||||
|
class ReVerbReader(OieReader):
|
||||||
|
|
||||||
|
def __init__(self):
|
||||||
|
self.inputSents = [sent.strip() for sent in open(ReVerbReader.RAW_SENTS_FILE).readlines()]
|
||||||
|
self.name = 'ReVerb'
|
||||||
|
|
||||||
|
def read(self, fn):
|
||||||
|
d = {}
|
||||||
|
with open(fn) as fin:
|
||||||
|
for line in fin:
|
||||||
|
data = line.strip().split('\t')
|
||||||
|
arg1, rel, arg2 = data[2:5]
|
||||||
|
confidence = data[11]
|
||||||
|
text = self.inputSents[int(data[1])-1]
|
||||||
|
|
||||||
|
curExtraction = Extraction(pred = rel, sent = text, confidence = float(confidence))
|
||||||
|
curExtraction.addArg(arg1)
|
||||||
|
curExtraction.addArg(arg2)
|
||||||
|
d[text] = d.get(text, []) + [curExtraction]
|
||||||
|
self.oie = d
|
||||||
|
|
||||||
|
# ReVerb requires a different files from which to get the input sentences
|
||||||
|
# Relative to repo root folder
|
||||||
|
RAW_SENTS_FILE = './raw_sentences/all.txt'
|
||||||
|
|
||||||
|
|
|
@ -0,0 +1,37 @@
|
||||||
|
""" Usage:
|
||||||
|
split_corpus --corpus=CORPUS_FN --reader=READER --in=INPUT_FN --out=OUTPUT_FN
|
||||||
|
|
||||||
|
Split OIE extractions according to raw sentences.
|
||||||
|
This is used in order to split a large file into train, dev and test.
|
||||||
|
|
||||||
|
READER - points out which oie reader to use (see dictionary for possible entries)
|
||||||
|
"""
|
||||||
|
from clausieReader import ClausieReader
|
||||||
|
from ollieReader import OllieReader
|
||||||
|
from openieFourReader import OpenieFourReader
|
||||||
|
from propsReader import PropSReader
|
||||||
|
from reVerbReader import ReVerbReader
|
||||||
|
from stanfordReader import StanfordReader
|
||||||
|
from docopt import docopt
|
||||||
|
import logging
|
||||||
|
logging.basicConfig(level = logging.INFO)
|
||||||
|
|
||||||
|
available_readers = {
|
||||||
|
"clausie": ClausieReader,
|
||||||
|
"ollie": OllieReader,
|
||||||
|
"openie4": OpenieFourReader,
|
||||||
|
"props": PropSReader,
|
||||||
|
"reverb": ReVerbReader,
|
||||||
|
"stanford": StanfordReader
|
||||||
|
}
|
||||||
|
|
||||||
|
|
||||||
|
if __name__ == "__main__":
|
||||||
|
args = docopt(__doc__)
|
||||||
|
inp = args["--in"]
|
||||||
|
out = args["--out"]
|
||||||
|
corpus = args["--corpus"]
|
||||||
|
reader = available_readers[args["--reader"]]()
|
||||||
|
reader.read(inp)
|
||||||
|
reader.split_to_corpus(corpus,
|
||||||
|
out)
|
|
@ -0,0 +1,22 @@
|
||||||
|
from oie_readers.oieReader import OieReader
|
||||||
|
from oie_readers.extraction import Extraction
|
||||||
|
|
||||||
|
class StanfordReader(OieReader):
|
||||||
|
|
||||||
|
def __init__(self):
|
||||||
|
self.name = 'Stanford'
|
||||||
|
|
||||||
|
def read(self, fn):
|
||||||
|
d = {}
|
||||||
|
with open(fn) as fin:
|
||||||
|
for line in fin:
|
||||||
|
data = line.strip().split('\t')
|
||||||
|
arg1, rel, arg2 = data[2:5]
|
||||||
|
confidence = data[11]
|
||||||
|
text = data[12]
|
||||||
|
|
||||||
|
curExtraction = Extraction(pred = rel, head_pred_index = -1, sent = text, confidence = float(confidence))
|
||||||
|
curExtraction.addArg(arg1)
|
||||||
|
curExtraction.addArg(arg2)
|
||||||
|
d[text] = d.get(text, []) + [curExtraction]
|
||||||
|
self.oie = d
|
56
3-NLP_services/src/Multi2OIE/carb/oie_readers/tabReader.py
Normal file
|
@ -0,0 +1,56 @@
|
||||||
|
""" Usage:
|
||||||
|
tabReader --in=INPUT_FILE
|
||||||
|
|
||||||
|
Read a tab-formatted file.
|
||||||
|
Each line consists of:
|
||||||
|
sent, prob, pred, arg1, arg2, ...
|
||||||
|
|
||||||
|
"""
|
||||||
|
|
||||||
|
from oie_readers.oieReader import OieReader
|
||||||
|
from oie_readers.extraction import Extraction
|
||||||
|
from docopt import docopt
|
||||||
|
import logging
|
||||||
|
import ipdb
|
||||||
|
|
||||||
|
logging.basicConfig(level = logging.DEBUG)
|
||||||
|
|
||||||
|
class TabReader(OieReader):
|
||||||
|
|
||||||
|
def __init__(self):
|
||||||
|
self.name = 'TabReader'
|
||||||
|
|
||||||
|
def read(self, fn):
|
||||||
|
"""
|
||||||
|
Read a tabbed format line
|
||||||
|
Each line consists of:
|
||||||
|
sent, prob, pred, arg1, arg2, ...
|
||||||
|
"""
|
||||||
|
d = {}
|
||||||
|
ex_index = 0
|
||||||
|
with open(fn) as fin:
|
||||||
|
for line in fin:
|
||||||
|
if not line.strip():
|
||||||
|
continue
|
||||||
|
data = line.strip().split('\t')
|
||||||
|
text, confidence, rel = data[:3]
|
||||||
|
curExtraction = Extraction(pred = rel,
|
||||||
|
head_pred_index = None,
|
||||||
|
sent = text,
|
||||||
|
confidence = float(confidence),
|
||||||
|
question_dist = "./question_distributions/dist_wh_sbj_obj1.json",
|
||||||
|
index = ex_index)
|
||||||
|
ex_index += 1
|
||||||
|
|
||||||
|
for arg in data[3:]:
|
||||||
|
curExtraction.addArg(arg)
|
||||||
|
|
||||||
|
d[text] = d.get(text, []) + [curExtraction]
|
||||||
|
self.oie = d
|
||||||
|
|
||||||
|
|
||||||
|
if __name__ == "__main__":
|
||||||
|
args = docopt(__doc__)
|
||||||
|
input_fn = args["--in"]
|
||||||
|
tr = TabReader()
|
||||||
|
tr.read(input_fn)
|
59
3-NLP_services/src/Multi2OIE/carb/tabReader.py
Normal file
|
@ -0,0 +1,59 @@
|
||||||
|
""" Usage:
|
||||||
|
tabReader --in=INPUT_FILE
|
||||||
|
|
||||||
|
Read a tab-formatted file.
|
||||||
|
Each line consists of:
|
||||||
|
sent, prob, pred, arg1, arg2, ...
|
||||||
|
|
||||||
|
"""
|
||||||
|
|
||||||
|
from carb.oieReader import OieReader
|
||||||
|
from carb.extraction import Extraction
|
||||||
|
from docopt import docopt
|
||||||
|
import logging
|
||||||
|
import ipdb
|
||||||
|
|
||||||
|
logging.basicConfig(level = logging.DEBUG)
|
||||||
|
|
||||||
|
class TabReader(OieReader):
|
||||||
|
|
||||||
|
def __init__(self):
|
||||||
|
self.name = 'TabReader'
|
||||||
|
|
||||||
|
def read(self, fn):
|
||||||
|
"""
|
||||||
|
Read a tabbed format line
|
||||||
|
Each line consists of:
|
||||||
|
sent, prob, pred, arg1, arg2, ...
|
||||||
|
"""
|
||||||
|
d = {}
|
||||||
|
ex_index = 0
|
||||||
|
with open(fn) as fin:
|
||||||
|
for line in fin:
|
||||||
|
if not line.strip():
|
||||||
|
continue
|
||||||
|
data = line.strip().split('\t')
|
||||||
|
try:
|
||||||
|
text, confidence, rel = data[:3]
|
||||||
|
except ValueError:
|
||||||
|
continue
|
||||||
|
curExtraction = Extraction(pred = rel,
|
||||||
|
head_pred_index = None,
|
||||||
|
sent = text,
|
||||||
|
confidence = float(confidence),
|
||||||
|
question_dist = "./question_distributions/dist_wh_sbj_obj1.json",
|
||||||
|
index = ex_index)
|
||||||
|
ex_index += 1
|
||||||
|
|
||||||
|
for arg in data[3:]:
|
||||||
|
curExtraction.addArg(arg)
|
||||||
|
|
||||||
|
d[text] = d.get(text, []) + [curExtraction]
|
||||||
|
self.oie = d
|
||||||
|
|
||||||
|
|
||||||
|
if __name__ == "__main__":
|
||||||
|
args = docopt(__doc__)
|
||||||
|
input_fn = args["--in"]
|
||||||
|
tr = TabReader()
|
||||||
|
tr.read(input_fn)
|
162
3-NLP_services/src/Multi2OIE/dataset.py
Normal file
|
@ -0,0 +1,162 @@
|
||||||
|
import torch
|
||||||
|
import numpy as np
|
||||||
|
from torch.utils.data import DataLoader
|
||||||
|
from torch.utils.data import Dataset
|
||||||
|
from utils import utils
|
||||||
|
from transformers import BertTokenizer
|
||||||
|
from utils.bio import pred_tag2idx, arg_tag2idx
|
||||||
|
|
||||||
|
|
||||||
|
def load_data(data_path,
|
||||||
|
batch_size,
|
||||||
|
max_len=64,
|
||||||
|
train=True,
|
||||||
|
tokenizer_config='bert-base-cased'):
|
||||||
|
if train:
|
||||||
|
return DataLoader(
|
||||||
|
dataset=OieDataset(
|
||||||
|
data_path,
|
||||||
|
max_len,
|
||||||
|
tokenizer_config),
|
||||||
|
batch_size=batch_size,
|
||||||
|
shuffle=True,
|
||||||
|
num_workers=4,
|
||||||
|
pin_memory=True,
|
||||||
|
drop_last=True)
|
||||||
|
else:
|
||||||
|
return DataLoader(
|
||||||
|
dataset=OieEvalDataset(
|
||||||
|
data_path,
|
||||||
|
max_len,
|
||||||
|
tokenizer_config),
|
||||||
|
batch_size=batch_size,
|
||||||
|
num_workers=4,
|
||||||
|
pin_memory=True)
|
||||||
|
|
||||||
|
|
||||||
|
class OieDataset(Dataset):
|
||||||
|
def __init__(self, data_path, max_len=64, tokenizer_config='bert-base-cased'):
|
||||||
|
data = utils.load_pkl(data_path)
|
||||||
|
self.tokens = data['tokens']
|
||||||
|
self.single_pred_labels = data['single_pred_labels']
|
||||||
|
self.single_arg_labels = data['single_arg_labels']
|
||||||
|
self.all_pred_labels = data['all_pred_labels']
|
||||||
|
|
||||||
|
self.max_len = max_len
|
||||||
|
self.tokenizer = BertTokenizer.from_pretrained(tokenizer_config)
|
||||||
|
self.vocab = self.tokenizer.vocab
|
||||||
|
|
||||||
|
self.pad_idx = self.vocab['[PAD]']
|
||||||
|
self.cls_idx = self.vocab['[CLS]']
|
||||||
|
self.sep_idx = self.vocab['[SEP]']
|
||||||
|
self.mask_idx = self.vocab['[MASK]']
|
||||||
|
|
||||||
|
def add_pad(self, token_ids):
|
||||||
|
diff = self.max_len - len(token_ids)
|
||||||
|
if diff > 0:
|
||||||
|
token_ids += [self.pad_idx] * diff
|
||||||
|
else:
|
||||||
|
token_ids = token_ids[:self.max_len-1] + [self.sep_idx]
|
||||||
|
return token_ids
|
||||||
|
|
||||||
|
def add_special_token(self, token_ids):
|
||||||
|
return [self.cls_idx] + token_ids + [self.sep_idx]
|
||||||
|
|
||||||
|
def idx2mask(self, token_ids):
|
||||||
|
return [token_id != self.pad_idx for token_id in token_ids]
|
||||||
|
|
||||||
|
def add_pad_to_labels(self, pred_label, arg_label, all_pred_label):
|
||||||
|
pred_outside = np.array([pred_tag2idx['O']])
|
||||||
|
arg_outside = np.array([arg_tag2idx['O']])
|
||||||
|
|
||||||
|
pred_label = np.concatenate([pred_outside, pred_label, pred_outside])
|
||||||
|
arg_label = np.concatenate([arg_outside, arg_label, arg_outside])
|
||||||
|
all_pred_label = np.concatenate([pred_outside, all_pred_label, pred_outside])
|
||||||
|
|
||||||
|
diff = self.max_len - pred_label.shape[0]
|
||||||
|
if diff > 0:
|
||||||
|
pred_pad = np.array([pred_tag2idx['O']] * diff)
|
||||||
|
arg_pad = np.array([arg_tag2idx['O']] * diff)
|
||||||
|
pred_label = np.concatenate([pred_label, pred_pad])
|
||||||
|
arg_label = np.concatenate([arg_label, arg_pad])
|
||||||
|
all_pred_label = np.concatenate([all_pred_label, pred_pad])
|
||||||
|
elif diff == 0:
|
||||||
|
pass
|
||||||
|
else:
|
||||||
|
pred_label = np.concatenate([pred_label[:-1], pred_outside])
|
||||||
|
arg_label = np.concatenate([arg_label[:-1], arg_outside])
|
||||||
|
all_pred_label = np.concatenate([all_pred_label[:-1], pred_outside])
|
||||||
|
return [pred_label, arg_label, all_pred_label]
|
||||||
|
|
||||||
|
def __len__(self):
|
||||||
|
return len(self.tokens)
|
||||||
|
|
||||||
|
def __getitem__(self, idx):
|
||||||
|
token_ids = self.tokenizer.convert_tokens_to_ids(self.tokens[idx])
|
||||||
|
token_ids_padded = self.add_pad(self.add_special_token(token_ids))
|
||||||
|
att_mask = self.idx2mask(token_ids_padded)
|
||||||
|
labels = self.add_pad_to_labels(
|
||||||
|
self.single_pred_labels[idx],
|
||||||
|
self.single_arg_labels[idx],
|
||||||
|
self.all_pred_labels[idx])
|
||||||
|
single_pred_label, single_arg_label, all_pred_label = labels
|
||||||
|
|
||||||
|
assert len(token_ids_padded) == self.max_len
|
||||||
|
assert len(att_mask) == self.max_len
|
||||||
|
assert single_pred_label.shape[0] == self.max_len
|
||||||
|
assert single_arg_label.shape[0] == self.max_len
|
||||||
|
assert all_pred_label.shape[0] == self.max_len
|
||||||
|
|
||||||
|
batch = [
|
||||||
|
torch.tensor(token_ids_padded),
|
||||||
|
torch.tensor(att_mask),
|
||||||
|
torch.tensor(single_pred_label),
|
||||||
|
torch.tensor(single_arg_label),
|
||||||
|
torch.tensor(all_pred_label)
|
||||||
|
]
|
||||||
|
return batch
|
||||||
|
|
||||||
|
|
||||||
|
class OieEvalDataset(Dataset):
|
||||||
|
def __init__(self, data_path, max_len, tokenizer_config='bert-base-cased'):
|
||||||
|
self.sentences = utils.load_pkl(data_path)
|
||||||
|
self.tokenizer = BertTokenizer.from_pretrained(tokenizer_config)
|
||||||
|
self.vocab = self.tokenizer.vocab
|
||||||
|
self.max_len = max_len
|
||||||
|
|
||||||
|
self.pad_idx = self.vocab['[PAD]']
|
||||||
|
self.cls_idx = self.vocab['[CLS]']
|
||||||
|
self.sep_idx = self.vocab['[SEP]']
|
||||||
|
self.mask_idx = self.vocab['[MASK]']
|
||||||
|
|
||||||
|
def add_pad(self, token_ids):
|
||||||
|
diff = self.max_len - len(token_ids)
|
||||||
|
if diff > 0:
|
||||||
|
token_ids += [self.pad_idx] * diff
|
||||||
|
else:
|
||||||
|
token_ids = token_ids[:self.max_len-1] + [self.sep_idx]
|
||||||
|
return token_ids
|
||||||
|
|
||||||
|
def idx2mask(self, token_ids):
|
||||||
|
return [token_id != self.pad_idx for token_id in token_ids]
|
||||||
|
|
||||||
|
def __len__(self):
|
||||||
|
return len(self.sentences)
|
||||||
|
|
||||||
|
def __getitem__(self, idx):
|
||||||
|
token_ids = self.add_pad(self.tokenizer.encode(self.sentences[idx]))
|
||||||
|
att_mask = self.idx2mask(token_ids)
|
||||||
|
token_strs = self.tokenizer.convert_ids_to_tokens(token_ids)
|
||||||
|
sentence = self.sentences[idx]
|
||||||
|
|
||||||
|
assert len(token_ids) == self.max_len
|
||||||
|
assert len(att_mask) == self.max_len
|
||||||
|
assert len(token_strs) == self.max_len
|
||||||
|
batch = [
|
||||||
|
torch.tensor(token_ids),
|
||||||
|
torch.tensor(att_mask),
|
||||||
|
token_strs,
|
||||||
|
sentence
|
||||||
|
]
|
||||||
|
return batch
|
||||||
|
|
97
3-NLP_services/src/Multi2OIE/environment.yml
Normal file
|
@ -0,0 +1,97 @@
|
||||||
|
name: multi2oie
|
||||||
|
channels:
|
||||||
|
- conda-forge
|
||||||
|
- defaults
|
||||||
|
dependencies:
|
||||||
|
- _libgcc_mutex=0.1=main
|
||||||
|
- _pytorch_select=0.2=gpu_0
|
||||||
|
- attrs=19.3.0=py_0
|
||||||
|
- blas=1.0=mkl
|
||||||
|
- brotlipy=0.7.0=py37h8f50634_1000
|
||||||
|
- ca-certificates=2020.4.5.1=hecc5488_0
|
||||||
|
- catalogue=1.0.0=py_0
|
||||||
|
- certifi=2020.4.5.1=py37hc8dfbb8_0
|
||||||
|
- cffi=1.14.0=py37he30daa8_1
|
||||||
|
- chardet=3.0.4=py37hc8dfbb8_1006
|
||||||
|
- cryptography=2.9.2=py37hb09aad4_0
|
||||||
|
- cudatoolkit=10.1.243=h6bb024c_0
|
||||||
|
- cudnn=7.6.5=cuda10.1_0
|
||||||
|
- cymem=2.0.3=py37h3340039_1
|
||||||
|
- cython-blis=0.4.1=py37h8f50634_1
|
||||||
|
- idna=2.9=py_1
|
||||||
|
- importlib-metadata=1.6.0=py37hc8dfbb8_0
|
||||||
|
- importlib_metadata=1.6.0=0
|
||||||
|
- intel-openmp=2020.1=217
|
||||||
|
- joblib=0.15.1=py_0
|
||||||
|
- jsonschema=3.2.0=py37hc8dfbb8_1
|
||||||
|
- ld_impl_linux-64=2.33.1=h53a641e_7
|
||||||
|
- libedit=3.1.20181209=hc058e9b_0
|
||||||
|
- libffi=3.3=he6710b0_1
|
||||||
|
- libgcc-ng=9.1.0=hdf63c60_0
|
||||||
|
- libgfortran-ng=7.3.0=hdf63c60_0
|
||||||
|
- libstdcxx-ng=9.1.0=hdf63c60_0
|
||||||
|
- mkl=2020.1=217
|
||||||
|
- mkl-service=2.3.0=py37he904b0f_0
|
||||||
|
- mkl_fft=1.0.15=py37ha843d7b_0
|
||||||
|
- mkl_random=1.1.1=py37h0573a6f_0
|
||||||
|
- murmurhash=1.0.0=py37h3340039_0
|
||||||
|
- ncurses=6.2=he6710b0_1
|
||||||
|
- ninja=1.9.0=py37hfd86e86_0
|
||||||
|
- numpy=1.18.1=py37h4f9e942_0
|
||||||
|
- numpy-base=1.18.1=py37hde5b4d6_1
|
||||||
|
- openssl=1.1.1g=h516909a_0
|
||||||
|
- pandas=1.0.3=py37h0573a6f_0
|
||||||
|
- pip=20.0.2=py37_3
|
||||||
|
- plac=0.9.6=py37_0
|
||||||
|
- preshed=3.0.2=py37h3340039_2
|
||||||
|
- pycparser=2.20=py_0
|
||||||
|
- pyopenssl=19.1.0=py_1
|
||||||
|
- pyrsistent=0.16.0=py37h8f50634_0
|
||||||
|
- pysocks=1.7.1=py37hc8dfbb8_1
|
||||||
|
- python=3.7.7=hcff3b4d_5
|
||||||
|
- python-dateutil=2.8.1=py_0
|
||||||
|
- python_abi=3.7=1_cp37m
|
||||||
|
- pytorch=1.4.0=cuda101py37h02f0884_0
|
||||||
|
- pytz=2020.1=py_0
|
||||||
|
- readline=8.0=h7b6447c_0
|
||||||
|
- requests=2.23.0=pyh8c360ce_2
|
||||||
|
- scikit-learn=0.22.1=py37hd81dba3_0
|
||||||
|
- scipy=1.4.1=py37h0b6359f_0
|
||||||
|
- setuptools=46.4.0=py37_0
|
||||||
|
- six=1.14.0=py37_0
|
||||||
|
- spacy=2.2.4=py37h99015e2_1
|
||||||
|
- sqlite=3.31.1=h62c20be_1
|
||||||
|
- srsly=1.0.2=py37h3340039_0
|
||||||
|
- thinc=7.4.0=py37h99015e2_2
|
||||||
|
- tk=8.6.8=hbc83047_0
|
||||||
|
- tqdm=4.46.0=pyh9f0ad1d_0
|
||||||
|
- urllib3=1.25.9=py_0
|
||||||
|
- wasabi=0.6.0=py_0
|
||||||
|
- wheel=0.34.2=py37_0
|
||||||
|
- xz=5.2.5=h7b6447c_0
|
||||||
|
- zipp=3.1.0=py_0
|
||||||
|
- zlib=1.2.11=h7b6447c_3
|
||||||
|
- pip:
|
||||||
|
- backcall==0.1.0
|
||||||
|
- click==7.1.2
|
||||||
|
- decorator==4.4.2
|
||||||
|
- docopt==0.6.2
|
||||||
|
- filelock==3.0.12
|
||||||
|
- ipdb==0.13.2
|
||||||
|
- ipython==7.14.0
|
||||||
|
- ipython-genutils==0.2.0
|
||||||
|
- jedi==0.17.0
|
||||||
|
- nltk==3.5
|
||||||
|
- parso==0.7.0
|
||||||
|
- pexpect==4.8.0
|
||||||
|
- pickleshare==0.7.5
|
||||||
|
- prompt-toolkit==3.0.5
|
||||||
|
- ptyprocess==0.6.0
|
||||||
|
- pygments==2.6.1
|
||||||
|
- regex==2020.5.14
|
||||||
|
- sacremoses==0.0.43
|
||||||
|
- sentencepiece==0.1.91
|
||||||
|
- tokenizers==0.7.0
|
||||||
|
- traitlets==4.3.3
|
||||||
|
- transformers==2.10.0
|
||||||
|
- wcwidth==0.1.9
|
1672
3-NLP_services/src/Multi2OIE/evaluate/OIE2016_dev.txt
Normal file
1730
3-NLP_services/src/Multi2OIE/evaluate/OIE2016_test.txt
Normal file
44407
3-NLP_services/src/Multi2OIE/evaluate/Re-OIE2016.json
Normal file
0
3-NLP_services/src/Multi2OIE/evaluate/__init__.py
Normal file
21
3-NLP_services/src/Multi2OIE/evaluate/argument.py
Normal file
|
@ -0,0 +1,21 @@
|
||||||
|
import nltk
|
||||||
|
from operator import itemgetter
|
||||||
|
|
||||||
|
class Argument:
|
||||||
|
def __init__(self, arg):
|
||||||
|
self.words = [x for x in arg[0].strip().split(' ') if x]
|
||||||
|
self.posTags = map(itemgetter(1), nltk.pos_tag(self.words))
|
||||||
|
self.indices = arg[1]
|
||||||
|
self.feats = {}
|
||||||
|
|
||||||
|
def __str__(self):
|
||||||
|
return "({})".format('\t'.join(map(str,
|
||||||
|
[escape_special_chars(' '.join(self.words)),
|
||||||
|
str(self.indices)])))
|
||||||
|
|
||||||
|
COREF = 'coref'
|
||||||
|
|
||||||
|
## Helper functions
|
||||||
|
def escape_special_chars(s):
|
||||||
|
return s.replace('\t', '\\t')
|
||||||
|
|
52038
3-NLP_services/src/Multi2OIE/evaluate/dev.oie.conll.txt
Normal file
219
3-NLP_services/src/Multi2OIE/evaluate/evaluate.py
Normal file
|
@ -0,0 +1,219 @@
|
||||||
|
#!/usr/bin/env python3
|
||||||
|
# -*- coding: utf-8 -*-
|
||||||
|
"""
|
||||||
|
Created on Thu Oct 25 19:24:30 2018
|
||||||
|
|
||||||
|
@author: longzhan
|
||||||
|
"""
|
||||||
|
|
||||||
|
import string
|
||||||
|
import numpy as np
|
||||||
|
from sklearn.metrics import precision_recall_curve
|
||||||
|
from sklearn.metrics import auc
|
||||||
|
import re
|
||||||
|
import logging
|
||||||
|
import sys
|
||||||
|
logging.basicConfig(level=logging.INFO)
|
||||||
|
|
||||||
|
from evaluate.generalReader import GeneralReader
|
||||||
|
from evaluate.goldReader import GoldReader
|
||||||
|
from evaluate.gold_relabel import Relabel_GoldReader
|
||||||
|
from evaluate.matcher import Matcher
|
||||||
|
from operator import itemgetter
|
||||||
|
|
||||||
|
|
||||||
|
class Benchmark:
|
||||||
|
''' Compare the gold OIE dataset against a predicted equivalent '''
|
||||||
|
def __init__(self, gold_fn):
|
||||||
|
''' Load gold Open IE, this will serve to compare against using the compare function '''
|
||||||
|
if 'Re' in gold_fn:
|
||||||
|
gr = Relabel_GoldReader()
|
||||||
|
else:
|
||||||
|
gr = GoldReader()
|
||||||
|
gr.read(gold_fn)
|
||||||
|
self.gold = gr.oie
|
||||||
|
|
||||||
|
def compare(self, predicted, matchingFunc, output_fn, error_file = None):
|
||||||
|
''' Compare gold against predicted using a specified matching function.
|
||||||
|
Outputs PR curve to output_fn '''
|
||||||
|
|
||||||
|
y_true = []
|
||||||
|
y_scores = []
|
||||||
|
errors = []
|
||||||
|
|
||||||
|
correctTotal = 0
|
||||||
|
unmatchedCount = 0
|
||||||
|
|
||||||
|
non_sent = 0
|
||||||
|
non_match = 0
|
||||||
|
|
||||||
|
predicted = Benchmark.normalizeDict(predicted)
|
||||||
|
gold = Benchmark.normalizeDict(self.gold)
|
||||||
|
|
||||||
|
for sent, goldExtractions in gold.items():
|
||||||
|
if sent not in predicted:
|
||||||
|
# The extractor didn't find any extractions for this sentence
|
||||||
|
for goldEx in goldExtractions:
|
||||||
|
non_sent += 1
|
||||||
|
unmatchedCount += len(goldExtractions)
|
||||||
|
correctTotal += len(goldExtractions)
|
||||||
|
continue
|
||||||
|
|
||||||
|
predictedExtractions = predicted[sent]
|
||||||
|
|
||||||
|
for goldEx in goldExtractions:
|
||||||
|
correctTotal += 1
|
||||||
|
found = False
|
||||||
|
|
||||||
|
for predictedEx in predictedExtractions:
|
||||||
|
if output_fn in predictedEx.matched:
|
||||||
|
# This predicted extraction was already matched against a gold extraction
|
||||||
|
# Don't allow to match it again
|
||||||
|
continue
|
||||||
|
|
||||||
|
if matchingFunc(goldEx,
|
||||||
|
predictedEx,
|
||||||
|
ignoreStopwords=True,
|
||||||
|
ignoreCase=True):
|
||||||
|
|
||||||
|
y_true.append(1)
|
||||||
|
y_scores.append(predictedEx.confidence)
|
||||||
|
predictedEx.matched.append(output_fn)
|
||||||
|
found = True
|
||||||
|
break
|
||||||
|
|
||||||
|
if not found:
|
||||||
|
non_match += 1
|
||||||
|
errors.append(goldEx.index)
|
||||||
|
unmatchedCount += 1
|
||||||
|
|
||||||
|
for predictedEx in [x for x in predictedExtractions if (output_fn not in x.matched)]:
|
||||||
|
# Add false positives
|
||||||
|
y_true.append(0)
|
||||||
|
y_scores.append(predictedEx.confidence)
|
||||||
|
|
||||||
|
y_true = y_true
|
||||||
|
y_scores = y_scores
|
||||||
|
|
||||||
|
print("non_sent: ", non_sent)
|
||||||
|
print("non_match: ", non_match)
|
||||||
|
print("correctTotal: ", correctTotal)
|
||||||
|
print("unmatchedCount: ", unmatchedCount)
|
||||||
|
|
||||||
|
# recall on y_true, y (r')_scores computes |covered by extractor| / |True in what's covered by extractor|
|
||||||
|
# to get to true recall we do:
|
||||||
|
# r' * (|True in what's covered by extractor| / |True in gold|) = |true in what's covered| / |true in gold|
|
||||||
|
(p, r), optimal = Benchmark.prCurve(np.array(y_true), np.array(y_scores),
|
||||||
|
recallMultiplier = ((correctTotal - unmatchedCount)/float(correctTotal)))
|
||||||
|
cur_auc = auc(r, p)
|
||||||
|
print("AUC: {}\n Optimal (precision, recall, F1, threshold): {}".format(cur_auc, optimal))
|
||||||
|
|
||||||
|
# Write error log to file
|
||||||
|
if error_file:
|
||||||
|
logging.info("Writing {} error indices to {}".format(len(errors),
|
||||||
|
error_file))
|
||||||
|
with open(error_file, 'w') as fout:
|
||||||
|
fout.write('\n'.join([str(error)
|
||||||
|
for error
|
||||||
|
in errors]) + '\n')
|
||||||
|
|
||||||
|
# write PR to file
|
||||||
|
with open(output_fn, 'w') as fout:
|
||||||
|
fout.write('{0}\t{1}\n'.format("Precision", "Recall"))
|
||||||
|
for cur_p, cur_r in sorted(zip(p, r), key = lambda cur: cur[1]):
|
||||||
|
fout.write('{0}\t{1}\n'.format(cur_p, cur_r))
|
||||||
|
|
||||||
|
return optimal[:-1], cur_auc
|
||||||
|
|
||||||
|
@staticmethod
|
||||||
|
def prCurve(y_true, y_scores, recallMultiplier):
|
||||||
|
# Recall multiplier - accounts for the percentage examples unreached
|
||||||
|
# Return (precision [list], recall[list]), (Optimal F1, Optimal threshold)
|
||||||
|
y_scores = [score \
|
||||||
|
if not (np.isnan(score) or (not np.isfinite(score))) \
|
||||||
|
else 0
|
||||||
|
for score in y_scores]
|
||||||
|
|
||||||
|
precision_ls, recall_ls, thresholds = precision_recall_curve(y_true, y_scores)
|
||||||
|
recall_ls = recall_ls * recallMultiplier
|
||||||
|
optimal = max([(precision, recall, f_beta(precision, recall, beta = 1), threshold)
|
||||||
|
for ((precision, recall), threshold)
|
||||||
|
in zip(zip(precision_ls[:-1], recall_ls[:-1]),
|
||||||
|
thresholds)],
|
||||||
|
key=itemgetter(2)) # Sort by f1 score
|
||||||
|
|
||||||
|
return ((precision_ls, recall_ls),
|
||||||
|
optimal)
|
||||||
|
|
||||||
|
# Helper functions:
|
||||||
|
@staticmethod
|
||||||
|
def normalizeDict(d):
|
||||||
|
return dict([(Benchmark.normalizeKey(k), v) for k, v in d.items()])
|
||||||
|
|
||||||
|
@staticmethod
|
||||||
|
def normalizeKey(k):
|
||||||
|
return Benchmark.removePunct(Benchmark.PTB_unescape(k.replace(' ','')))
|
||||||
|
|
||||||
|
@staticmethod
|
||||||
|
def PTB_escape(s):
|
||||||
|
for u, e in Benchmark.PTB_ESCAPES:
|
||||||
|
s = s.replace(u, e)
|
||||||
|
return s
|
||||||
|
|
||||||
|
@staticmethod
|
||||||
|
def PTB_unescape(s):
|
||||||
|
for u, e in Benchmark.PTB_ESCAPES:
|
||||||
|
s = s.replace(e, u)
|
||||||
|
return s
|
||||||
|
|
||||||
|
@staticmethod
|
||||||
|
def removePunct(s):
|
||||||
|
return Benchmark.regex.sub('', s)
|
||||||
|
|
||||||
|
# CONSTANTS
|
||||||
|
regex = re.compile('[%s]' % re.escape(string.punctuation))
|
||||||
|
|
||||||
|
# Penn treebank bracket escapes
|
||||||
|
# Taken from: https://github.com/nlplab/brat/blob/master/server/src/gtbtokenize.py
|
||||||
|
PTB_ESCAPES = [('(', '-LRB-'),
|
||||||
|
(')', '-RRB-'),
|
||||||
|
('[', '-LSB-'),
|
||||||
|
(']', '-RSB-'),
|
||||||
|
('{', '-LCB-'),
|
||||||
|
('}', '-RCB-'),]
|
||||||
|
|
||||||
|
|
||||||
|
def f_beta(precision, recall, beta = 1):
|
||||||
|
"""
|
||||||
|
Get F_beta score from precision and recall.
|
||||||
|
"""
|
||||||
|
beta = float(beta) # Make sure that results are in float
|
||||||
|
return (1 + pow(beta, 2)) * (precision * recall) / ((pow(beta, 2) * precision) + recall)
|
||||||
|
|
||||||
|
|
||||||
|
f1 = lambda precision, recall: f_beta(precision, recall, beta = 1)
|
||||||
|
|
||||||
|
#gold_flag = sys.argv[1] # to choose whether to use OIE2016 or Re-OIE2016
|
||||||
|
#in_path = sys.argv[2] # input file
|
||||||
|
#out_path = sys.argv[3] # output file
|
||||||
|
|
||||||
|
if __name__ == '__main__':
|
||||||
|
|
||||||
|
gold = gold_flag
|
||||||
|
matchingFunc = Matcher.lexicalMatch
|
||||||
|
error_fn = "error.txt"
|
||||||
|
|
||||||
|
if gold == "old":
|
||||||
|
gold_fn = "OIE2016.txt"
|
||||||
|
else:
|
||||||
|
gold_fn = "Re-OIE2016.json"
|
||||||
|
|
||||||
|
b = Benchmark(gold_fn)
|
||||||
|
s_fn = in_path
|
||||||
|
p = GeneralReader()
|
||||||
|
other_p = GeneralReader()
|
||||||
|
other_p.read(s_fn)
|
||||||
|
b.compare(predicted = other_p.oie,
|
||||||
|
matchingFunc = matchingFunc,
|
||||||
|
output_fn = out_path,
|
||||||
|
error_file = error_fn)
|
444
3-NLP_services/src/Multi2OIE/evaluate/extraction.py
Normal file
|
@ -0,0 +1,444 @@
|
||||||
|
from sklearn.preprocessing.data import binarize
|
||||||
|
from evaluate.argument import Argument
|
||||||
|
from operator import itemgetter
|
||||||
|
from collections import defaultdict
|
||||||
|
import nltk
|
||||||
|
import itertools
|
||||||
|
import logging
|
||||||
|
import numpy as np
|
||||||
|
import pdb
|
||||||
|
|
||||||
|
|
||||||
|
class Extraction:
|
||||||
|
"""
|
||||||
|
Stores sentence, single predicate and corresponding arguments.
|
||||||
|
"""
|
||||||
|
def __init__(self, pred, head_pred_index, sent, confidence, question_dist = '', index = -1):
|
||||||
|
self.pred = pred
|
||||||
|
self.head_pred_index = head_pred_index
|
||||||
|
self.sent = sent
|
||||||
|
self.args = []
|
||||||
|
self.confidence = confidence
|
||||||
|
self.matched = []
|
||||||
|
self.questions = {}
|
||||||
|
self.indsForQuestions = defaultdict(lambda: set())
|
||||||
|
self.is_mwp = False
|
||||||
|
self.question_dist = question_dist
|
||||||
|
self.index = index
|
||||||
|
|
||||||
|
def distArgFromPred(self, arg):
|
||||||
|
assert(len(self.pred) == 2)
|
||||||
|
dists = []
|
||||||
|
for x in self.pred[1]:
|
||||||
|
for y in arg.indices:
|
||||||
|
dists.append(abs(x - y))
|
||||||
|
|
||||||
|
return min(dists)
|
||||||
|
|
||||||
|
def argsByDistFromPred(self, question):
|
||||||
|
return sorted(self.questions[question], key = lambda arg: self.distArgFromPred(arg))
|
||||||
|
|
||||||
|
def addArg(self, arg, question = None):
|
||||||
|
self.args.append(arg)
|
||||||
|
if question:
|
||||||
|
self.questions[question] = self.questions.get(question,[]) + [Argument(arg)]
|
||||||
|
|
||||||
|
def noPronounArgs(self):
|
||||||
|
"""
|
||||||
|
Returns True iff all of this extraction's arguments are not pronouns.
|
||||||
|
"""
|
||||||
|
for (a, _) in self.args:
|
||||||
|
tokenized_arg = nltk.word_tokenize(a)
|
||||||
|
if len(tokenized_arg) == 1:
|
||||||
|
_, pos_tag = nltk.pos_tag(tokenized_arg)[0]
|
||||||
|
if ('PRP' in pos_tag):
|
||||||
|
return False
|
||||||
|
return True
|
||||||
|
|
||||||
|
def isContiguous(self):
|
||||||
|
return all([indices for (_, indices) in self.args])
|
||||||
|
|
||||||
|
def toBinary(self):
|
||||||
|
''' Try to represent this extraction's arguments as binary
|
||||||
|
If fails, this function will return an empty list. '''
|
||||||
|
|
||||||
|
ret = [self.elementToStr(self.pred)]
|
||||||
|
|
||||||
|
if len(self.args) == 2:
|
||||||
|
# we're in luck
|
||||||
|
return ret + [self.elementToStr(arg) for arg in self.args]
|
||||||
|
|
||||||
|
return []
|
||||||
|
|
||||||
|
if not self.isContiguous():
|
||||||
|
# give up on non contiguous arguments (as we need indexes)
|
||||||
|
return []
|
||||||
|
|
||||||
|
# otherwise, try to merge based on indices
|
||||||
|
# TODO: you can explore other methods for doing this
|
||||||
|
binarized = self.binarizeByIndex()
|
||||||
|
|
||||||
|
if binarized:
|
||||||
|
return ret + binarized
|
||||||
|
|
||||||
|
return []
|
||||||
|
|
||||||
|
|
||||||
|
def elementToStr(self, elem, print_indices = True):
|
||||||
|
''' formats an extraction element (pred or arg) as a raw string
|
||||||
|
removes indices and trailing spaces '''
|
||||||
|
if print_indices:
|
||||||
|
return str(elem)
|
||||||
|
if isinstance(elem, str):
|
||||||
|
return elem
|
||||||
|
if isinstance(elem, tuple):
|
||||||
|
ret = elem[0].rstrip().lstrip()
|
||||||
|
else:
|
||||||
|
ret = ' '.join(elem.words)
|
||||||
|
assert ret, "empty element? {0}".format(elem)
|
||||||
|
return ret
|
||||||
|
|
||||||
|
def binarizeByIndex(self):
|
||||||
|
extraction = [self.pred] + self.args
|
||||||
|
markPred = [(w, ind, i == 0) for i, (w, ind) in enumerate(extraction)]
|
||||||
|
sortedExtraction = sorted(markPred, key = lambda x : x[1][0])
|
||||||
|
s = ' '.join(['{1} {0} {1}'.format(self.elementToStr(elem), SEP) if elem[2] else self.elementToStr(elem) for elem in sortedExtraction])
|
||||||
|
binArgs = [a for a in s.split(SEP) if a.rstrip().lstrip()]
|
||||||
|
|
||||||
|
if len(binArgs) == 2:
|
||||||
|
return binArgs
|
||||||
|
|
||||||
|
# failure
|
||||||
|
return []
|
||||||
|
|
||||||
|
def bow(self):
|
||||||
|
return ' '.join([self.elementToStr(elem) for elem in [self.pred] + self.args])
|
||||||
|
|
||||||
|
def getSortedArgs(self):
|
||||||
|
"""
|
||||||
|
Sort the list of arguments.
|
||||||
|
If a question distribution is provided - use it,
|
||||||
|
otherwise, default to the order of appearance in the sentence.
|
||||||
|
"""
|
||||||
|
if self.question_dist:
|
||||||
|
# There's a question distribtuion - use it
|
||||||
|
return self.sort_args_by_distribution()
|
||||||
|
ls = []
|
||||||
|
for q, args in self.questions.iteritems():
|
||||||
|
if (len(args) != 1):
|
||||||
|
logging.debug("Not one argument: {}".format(args))
|
||||||
|
continue
|
||||||
|
arg = args[0]
|
||||||
|
indices = list(self.indsForQuestions[q].union(arg.indices))
|
||||||
|
if not indices:
|
||||||
|
logging.debug("Empty indexes for arg {} -- backing to zero".format(arg))
|
||||||
|
indices = [0]
|
||||||
|
ls.append(((arg, q), indices))
|
||||||
|
return [a for a, _ in sorted(ls,key = lambda x: min(x[1]))]
|
||||||
|
|
||||||
|
def question_prob_for_loc(self, question, loc):
|
||||||
|
"""
|
||||||
|
Returns the probability of the given question leading to argument
|
||||||
|
appearing in the given location in the output slot.
|
||||||
|
"""
|
||||||
|
gen_question = generalize_question(question)
|
||||||
|
q_dist = self.question_dist[gen_question]
|
||||||
|
logging.debug("distribution of {}: {}".format(gen_question,
|
||||||
|
q_dist))
|
||||||
|
|
||||||
|
return float(q_dist.get(loc, 0)) / \
|
||||||
|
sum(q_dist.values())
|
||||||
|
|
||||||
|
def sort_args_by_distribution(self):
|
||||||
|
"""
|
||||||
|
Use this instance's question distribution (this func assumes it exists)
|
||||||
|
in determining the positioning of the arguments.
|
||||||
|
Greedy algorithm:
|
||||||
|
0. Decide on which argument will serve as the ``subject'' (first slot) of this extraction
|
||||||
|
0.1 Based on the most probable one for this spot
|
||||||
|
(special care is given to select the highly-influential subject position)
|
||||||
|
1. For all other arguments, sort arguments by the prevalance of their questions
|
||||||
|
2. For each argument:
|
||||||
|
2.1 Assign to it the most probable slot still available
|
||||||
|
2.2 If non such exist (fallback) - default to put it in the last location
|
||||||
|
"""
|
||||||
|
INF_LOC = 100 # Used as an impractical last argument
|
||||||
|
|
||||||
|
# Store arguments by slot
|
||||||
|
ret = {INF_LOC: []}
|
||||||
|
logging.debug("sorting: {}".format(self.questions))
|
||||||
|
|
||||||
|
# Find the most suitable arguemnt for the subject location
|
||||||
|
logging.debug("probs for subject: {}".format([(q, self.question_prob_for_loc(q, 0))
|
||||||
|
for (q, _) in self.questions.iteritems()]))
|
||||||
|
|
||||||
|
subj_question, subj_args = max(self.questions.iteritems(),
|
||||||
|
key = lambda x: self.question_prob_for_loc(x[0], 0))
|
||||||
|
|
||||||
|
ret[0] = [(subj_args[0], subj_question)]
|
||||||
|
|
||||||
|
# Find the rest
|
||||||
|
for (question, args) in sorted([(q, a)
|
||||||
|
for (q, a) in self.questions.iteritems() if (q not in [subj_question])],
|
||||||
|
key = lambda x: \
|
||||||
|
sum(self.question_dist[generalize_question(x[0])].values()),
|
||||||
|
reverse = True):
|
||||||
|
gen_question = generalize_question(question)
|
||||||
|
arg = args[0]
|
||||||
|
assigned_flag = False
|
||||||
|
for (loc, count) in sorted(self.question_dist[gen_question].iteritems(),
|
||||||
|
key = lambda x: x[1],
|
||||||
|
reverse = True):
|
||||||
|
if loc not in ret:
|
||||||
|
# Found an empty slot for this item
|
||||||
|
# Place it there and break out
|
||||||
|
ret[loc] = [(arg, question)]
|
||||||
|
assigned_flag = True
|
||||||
|
break
|
||||||
|
|
||||||
|
if not assigned_flag:
|
||||||
|
# Add this argument to the non-assigned (hopefully doesn't happen much)
|
||||||
|
logging.debug("Couldn't find an open assignment for {}".format((arg, gen_question)))
|
||||||
|
ret[INF_LOC].append((arg, question))
|
||||||
|
|
||||||
|
logging.debug("Linearizing arg list: {}".format(ret))
|
||||||
|
|
||||||
|
# Finished iterating - consolidate and return a list of arguments
|
||||||
|
return [arg
|
||||||
|
for (_, arg_ls) in sorted(ret.iteritems(),
|
||||||
|
key = lambda x: int(x[0]))
|
||||||
|
for arg in arg_ls]
|
||||||
|
|
||||||
|
|
||||||
|
def __str__(self):
|
||||||
|
pred_str = self.elementToStr(self.pred)
|
||||||
|
return '{}\t{}\t{}'.format(self.get_base_verb(pred_str),
|
||||||
|
self.compute_global_pred(pred_str,
|
||||||
|
self.questions.keys()),
|
||||||
|
'\t'.join([escape_special_chars(self.augment_arg_with_question(self.elementToStr(arg),
|
||||||
|
question))
|
||||||
|
for arg, question in self.getSortedArgs()]))
|
||||||
|
|
||||||
|
def get_base_verb(self, surface_pred):
|
||||||
|
"""
|
||||||
|
Given the surface pred, return the original annotated verb
|
||||||
|
"""
|
||||||
|
# Assumes that at this point the verb is always the last word
|
||||||
|
# in the surface predicate
|
||||||
|
return surface_pred.split(' ')[-1]
|
||||||
|
|
||||||
|
|
||||||
|
def compute_global_pred(self, surface_pred, questions):
|
||||||
|
"""
|
||||||
|
Given the surface pred and all instansiations of questions,
|
||||||
|
make global coherence decisions regarding the final form of the predicate
|
||||||
|
This should hopefully take care of multi word predicates and correct inflections
|
||||||
|
"""
|
||||||
|
from operator import itemgetter
|
||||||
|
split_surface = surface_pred.split(' ')
|
||||||
|
|
||||||
|
if len(split_surface) > 1:
|
||||||
|
# This predicate has a modal preceding the base verb
|
||||||
|
verb = split_surface[-1]
|
||||||
|
ret = split_surface[:-1] # get all of the elements in the modal
|
||||||
|
else:
|
||||||
|
verb = split_surface[0]
|
||||||
|
ret = []
|
||||||
|
|
||||||
|
split_questions = map(lambda question: question.split(' '),
|
||||||
|
questions)
|
||||||
|
|
||||||
|
preds = map(normalize_element,
|
||||||
|
map(itemgetter(QUESTION_TRG_INDEX),
|
||||||
|
split_questions))
|
||||||
|
if len(set(preds)) > 1:
|
||||||
|
# This predicate is appears in multiple ways, let's stick to the base form
|
||||||
|
ret.append(verb)
|
||||||
|
|
||||||
|
if len(set(preds)) == 1:
|
||||||
|
# Change the predciate to the inflected form
|
||||||
|
# if there's exactly one way in which the predicate is conveyed
|
||||||
|
ret.append(preds[0])
|
||||||
|
|
||||||
|
pps = map(normalize_element,
|
||||||
|
map(itemgetter(QUESTION_PP_INDEX),
|
||||||
|
split_questions))
|
||||||
|
|
||||||
|
obj2s = map(normalize_element,
|
||||||
|
map(itemgetter(QUESTION_OBJ2_INDEX),
|
||||||
|
split_questions))
|
||||||
|
|
||||||
|
if (len(set(pps)) == 1):
|
||||||
|
# If all questions for the predicate include the same pp attachemnt -
|
||||||
|
# assume it's a multiword predicate
|
||||||
|
self.is_mwp = True # Signal to arguments that they shouldn't take the preposition
|
||||||
|
ret.append(pps[0])
|
||||||
|
|
||||||
|
# Concat all elements in the predicate and return
|
||||||
|
return " ".join(ret).strip()
|
||||||
|
|
||||||
|
|
||||||
|
def augment_arg_with_question(self, arg, question):
|
||||||
|
"""
|
||||||
|
Decide what elements from the question to incorporate in the given
|
||||||
|
corresponding argument
|
||||||
|
"""
|
||||||
|
# Parse question
|
||||||
|
wh, aux, sbj, trg, obj1, pp, obj2 = map(normalize_element,
|
||||||
|
question.split(' ')[:-1]) # Last split is the question mark
|
||||||
|
|
||||||
|
# Place preposition in argument
|
||||||
|
# This is safer when dealing with n-ary arguments, as it's directly attaches to the
|
||||||
|
# appropriate argument
|
||||||
|
if (not self.is_mwp) and pp and (not obj2):
|
||||||
|
if not(arg.startswith("{} ".format(pp))):
|
||||||
|
# Avoid repeating the preporition in cases where both question and answer contain it
|
||||||
|
return " ".join([pp,
|
||||||
|
arg])
|
||||||
|
|
||||||
|
# Normal cases
|
||||||
|
return arg
|
||||||
|
|
||||||
|
def clusterScore(self, cluster):
|
||||||
|
"""
|
||||||
|
Calculate cluster density score as the mean distance of the maximum distance of each slot.
|
||||||
|
Lower score represents a denser cluster.
|
||||||
|
"""
|
||||||
|
logging.debug("*-*-*- Cluster: {}".format(cluster))
|
||||||
|
|
||||||
|
# Find global centroid
|
||||||
|
arr = np.array([x for ls in cluster for x in ls])
|
||||||
|
centroid = np.sum(arr)/arr.shape[0]
|
||||||
|
logging.debug("Centroid: {}".format(centroid))
|
||||||
|
|
||||||
|
# Calculate mean over all maxmimum points
|
||||||
|
return np.average([max([abs(x - centroid) for x in ls]) for ls in cluster])
|
||||||
|
|
||||||
|
def resolveAmbiguity(self):
|
||||||
|
"""
|
||||||
|
Heursitic to map the elments (argument and predicates) of this extraction
|
||||||
|
back to the indices of the sentence.
|
||||||
|
"""
|
||||||
|
## TODO: This removes arguments for which there was no consecutive span found
|
||||||
|
## Part of these are non-consecutive arguments,
|
||||||
|
## but other could be a bug in recognizing some punctuation marks
|
||||||
|
|
||||||
|
elements = [self.pred] \
|
||||||
|
+ [(s, indices)
|
||||||
|
for (s, indices)
|
||||||
|
in self.args
|
||||||
|
if indices]
|
||||||
|
logging.debug("Resolving ambiguity in: {}".format(elements))
|
||||||
|
|
||||||
|
# Collect all possible combinations of arguments and predicate indices
|
||||||
|
# (hopefully it's not too much)
|
||||||
|
all_combinations = list(itertools.product(*map(itemgetter(1), elements)))
|
||||||
|
logging.debug("Number of combinations: {}".format(len(all_combinations)))
|
||||||
|
|
||||||
|
# Choose the ones with best clustering and unfold them
|
||||||
|
resolved_elements = zip(map(itemgetter(0), elements),
|
||||||
|
min(all_combinations,
|
||||||
|
key = lambda cluster: self.clusterScore(cluster)))
|
||||||
|
logging.debug("Resolved elements = {}".format(resolved_elements))
|
||||||
|
|
||||||
|
self.pred = resolved_elements[0]
|
||||||
|
self.args = resolved_elements[1:]
|
||||||
|
|
||||||
|
def conll(self, external_feats = {}):
|
||||||
|
"""
|
||||||
|
Return a CoNLL string representation of this extraction
|
||||||
|
"""
|
||||||
|
return '\n'.join(["\t".join(map(str,
|
||||||
|
[i, w] + \
|
||||||
|
list(self.pred) + \
|
||||||
|
[self.head_pred_index] + \
|
||||||
|
external_feats + \
|
||||||
|
[self.get_label(i)]))
|
||||||
|
for (i, w)
|
||||||
|
in enumerate(self.sent.split(" "))]) + '\n'
|
||||||
|
|
||||||
|
def get_label(self, index):
|
||||||
|
"""
|
||||||
|
Given an index of a word in the sentence -- returns the appropriate BIO conll label
|
||||||
|
Assumes that ambiguation was already resolved.
|
||||||
|
"""
|
||||||
|
# Get the element(s) in which this index appears
|
||||||
|
ent = [(elem_ind, elem)
|
||||||
|
for (elem_ind, elem)
|
||||||
|
in enumerate(map(itemgetter(1),
|
||||||
|
[self.pred] + self.args))
|
||||||
|
if index in elem]
|
||||||
|
|
||||||
|
if not ent:
|
||||||
|
# index doesnt appear in any element
|
||||||
|
return "O"
|
||||||
|
|
||||||
|
if len(ent) > 1:
|
||||||
|
# The same word appears in two different answers
|
||||||
|
# In this case we choose the first one as label
|
||||||
|
logging.warn("Index {} appears in one than more element: {}".\
|
||||||
|
format(index,
|
||||||
|
"\t".join(map(str,
|
||||||
|
[ent,
|
||||||
|
self.sent,
|
||||||
|
self.pred,
|
||||||
|
self.args]))))
|
||||||
|
|
||||||
|
## Some indices appear in more than one argument (ones where the above message appears)
|
||||||
|
## From empricial observation, these seem to mostly consist of different levels of granularity:
|
||||||
|
## what had _ been taken _ _ _ ? loan commitments topping $ 3 billion
|
||||||
|
## how much had _ been taken _ _ _ ? topping $ 3 billion
|
||||||
|
## In these cases we heuristically choose the shorter answer span, hopefully creating minimal spans
|
||||||
|
## E.g., in this example two arguemnts are created: (loan commitments, topping $ 3 billion)
|
||||||
|
|
||||||
|
elem_ind, elem = min(ent, key = lambda x: len(x[1]))
|
||||||
|
|
||||||
|
# Distinguish between predicate and arguments
|
||||||
|
prefix = "P" if elem_ind == 0 else "A{}".format(elem_ind - 1)
|
||||||
|
|
||||||
|
# Distinguish between Beginning and Inside labels
|
||||||
|
suffix = "B" if index == elem[0] else "I"
|
||||||
|
|
||||||
|
return "{}-{}".format(prefix, suffix)
|
||||||
|
|
||||||
|
def __str__(self):
|
||||||
|
return '{0}\t{1}'.format(self.elementToStr(self.pred,
|
||||||
|
print_indices = True),
|
||||||
|
'\t'.join([self.elementToStr(arg)
|
||||||
|
for arg
|
||||||
|
in self.args]))
|
||||||
|
|
||||||
|
# Flatten a list of lists
|
||||||
|
flatten = lambda l: [item for sublist in l for item in sublist]
|
||||||
|
|
||||||
|
|
||||||
|
def normalize_element(elem):
|
||||||
|
"""
|
||||||
|
Return a surface form of the given question element.
|
||||||
|
the output should be properly able to precede a predicate (or blank otherwise)
|
||||||
|
"""
|
||||||
|
return elem.replace("_", " ") \
|
||||||
|
if (elem != "_")\
|
||||||
|
else ""
|
||||||
|
|
||||||
|
## Helper functions
|
||||||
|
def escape_special_chars(s):
|
||||||
|
return s.replace('\t', '\\t')
|
||||||
|
|
||||||
|
|
||||||
|
def generalize_question(question):
|
||||||
|
"""
|
||||||
|
Given a question in the context of the sentence and the predicate index within
|
||||||
|
the question - return a generalized version which extracts only order-imposing features
|
||||||
|
"""
|
||||||
|
import nltk # Using nltk since couldn't get spaCy to agree on the tokenization
|
||||||
|
wh, aux, sbj, trg, obj1, pp, obj2 = question.split(' ')[:-1] # Last split is the question mark
|
||||||
|
return ' '.join([wh, sbj, obj1])
|
||||||
|
|
||||||
|
|
||||||
|
|
||||||
|
## CONSTANTS
|
||||||
|
SEP = ';;;'
|
||||||
|
QUESTION_TRG_INDEX = 3 # index of the predicate within the question
|
||||||
|
QUESTION_PP_INDEX = 5
|
||||||
|
QUESTION_OBJ2_INDEX = 6
|
43
3-NLP_services/src/Multi2OIE/evaluate/generalReader.py
Normal file
|
@ -0,0 +1,43 @@
|
||||||
|
# -*- coding: utf-8 -*-
|
||||||
|
"""
|
||||||
|
Created on Thu Nov 15 21:05:15 2018
|
||||||
|
|
||||||
|
@author: win 10
|
||||||
|
"""
|
||||||
|
|
||||||
|
from evaluate.oieReader import OieReader
|
||||||
|
from evaluate.extraction import Extraction
|
||||||
|
|
||||||
|
class GeneralReader(OieReader):
|
||||||
|
|
||||||
|
def __init__(self):
|
||||||
|
self.name = 'General'
|
||||||
|
|
||||||
|
def read(self, fn):
|
||||||
|
d = {}
|
||||||
|
with open(fn) as fin:
|
||||||
|
for line in fin:
|
||||||
|
data = line.strip().split('\t')
|
||||||
|
if len(data) >= 4:
|
||||||
|
arg1 = data[3]
|
||||||
|
rel = data[2]
|
||||||
|
arg_else = data[4:]
|
||||||
|
confidence = data[1]
|
||||||
|
text = data[0]
|
||||||
|
|
||||||
|
curExtraction = Extraction(pred = rel, head_pred_index=-1, sent = text, confidence = float(confidence))
|
||||||
|
curExtraction.addArg(arg1)
|
||||||
|
for arg in arg_else:
|
||||||
|
curExtraction.addArg(arg)
|
||||||
|
d[text] = d.get(text, []) + [curExtraction]
|
||||||
|
self.oie = d
|
||||||
|
|
||||||
|
if __name__ == "__main__":
|
||||||
|
fn = "../data/other_systems/openie4_test.txt"
|
||||||
|
reader = GeneralReader()
|
||||||
|
reader.read(fn)
|
||||||
|
for key in reader.oie:
|
||||||
|
print(key)
|
||||||
|
print(reader.oie[key][0].pred)
|
||||||
|
print(reader.oie[key][0].args)
|
||||||
|
print(reader.oie[key][0].confidence)
|
58
3-NLP_services/src/Multi2OIE/evaluate/goldReader.py
Normal file
|
@ -0,0 +1,58 @@
|
||||||
|
#!/usr/bin/env python3
|
||||||
|
# -*- coding: utf-8 -*-
|
||||||
|
"""
|
||||||
|
Created on Thu Oct 25 19:24:30 2018
|
||||||
|
|
||||||
|
@author: longzhan
|
||||||
|
"""
|
||||||
|
|
||||||
|
from evaluate.oieReader import OieReader
|
||||||
|
from evaluate.extraction import Extraction
|
||||||
|
from _collections import defaultdict
|
||||||
|
|
||||||
|
|
||||||
|
class GoldReader(OieReader):
|
||||||
|
|
||||||
|
# Path relative to repo root folder
|
||||||
|
default_filename = './oie_corpus/all.oie'
|
||||||
|
|
||||||
|
def __init__(self):
|
||||||
|
self.name = 'Gold'
|
||||||
|
|
||||||
|
def read(self, fn):
|
||||||
|
d = defaultdict(lambda: [])
|
||||||
|
multilingual = False
|
||||||
|
for lang in ['spanish']:
|
||||||
|
if lang in fn:
|
||||||
|
multilingual = True
|
||||||
|
encoding = lang
|
||||||
|
break
|
||||||
|
if multilingual and encoding == 'spanish':
|
||||||
|
fin = open(fn, 'r', encoding='latin-1')
|
||||||
|
else:
|
||||||
|
fin = open(fn)
|
||||||
|
for line_ind, line in enumerate(fin):
|
||||||
|
data = line.strip().split('\t')
|
||||||
|
text, rel = data[:2]
|
||||||
|
args = data[2:]
|
||||||
|
confidence = 1
|
||||||
|
|
||||||
|
curExtraction = Extraction(pred = rel.strip(),
|
||||||
|
head_pred_index = None,
|
||||||
|
sent = text.strip(),
|
||||||
|
confidence = float(confidence),
|
||||||
|
index = line_ind)
|
||||||
|
for arg in args:
|
||||||
|
curExtraction.addArg(arg)
|
||||||
|
|
||||||
|
d[text.strip()].append(curExtraction)
|
||||||
|
self.oie = d
|
||||||
|
|
||||||
|
|
||||||
|
if __name__ == '__main__' :
|
||||||
|
g = GoldReader()
|
||||||
|
g.read('../test.oie')
|
||||||
|
d = g.oie
|
||||||
|
e = list(d.items())[0]
|
||||||
|
print (e[1][0].bow())
|
||||||
|
print (g.count())
|
46
3-NLP_services/src/Multi2OIE/evaluate/gold_relabel.py
Normal file
|
@ -0,0 +1,46 @@
|
||||||
|
from evaluate.oieReader import OieReader
|
||||||
|
from evaluate.extraction import Extraction
|
||||||
|
from _collections import defaultdict
|
||||||
|
import json
|
||||||
|
|
||||||
|
class Relabel_GoldReader(OieReader):
|
||||||
|
|
||||||
|
# Path relative to repo root folder
|
||||||
|
default_filename = './oie_corpus/all.oie'
|
||||||
|
|
||||||
|
def __init__(self):
|
||||||
|
self.name = 'Relabel_Gold'
|
||||||
|
|
||||||
|
def read(self, fn):
|
||||||
|
d = defaultdict(lambda: [])
|
||||||
|
with open(fn) as fin:
|
||||||
|
data = json.load(fin)
|
||||||
|
for sentence in data:
|
||||||
|
tuples = data[sentence]
|
||||||
|
for t in tuples:
|
||||||
|
if t["pred"].strip() == "<be>":
|
||||||
|
rel = "[is]"
|
||||||
|
else:
|
||||||
|
rel = t["pred"].replace("<be> ","")
|
||||||
|
confidence = 1
|
||||||
|
|
||||||
|
curExtraction = Extraction(pred = rel,
|
||||||
|
head_pred_index = None,
|
||||||
|
sent = sentence,
|
||||||
|
confidence = float(confidence),
|
||||||
|
index = None)
|
||||||
|
if t["arg0"] != "":
|
||||||
|
curExtraction.addArg(t["arg0"])
|
||||||
|
if t["arg1"] != "":
|
||||||
|
curExtraction.addArg(t["arg1"])
|
||||||
|
if t["arg2"] != "":
|
||||||
|
curExtraction.addArg(t["arg2"])
|
||||||
|
if t["arg3"] != "":
|
||||||
|
curExtraction.addArg(t["arg3"])
|
||||||
|
if t["temp"] != "":
|
||||||
|
curExtraction.addArg(t["temp"])
|
||||||
|
if t["loc"] != "":
|
||||||
|
curExtraction.addArg(t["loc"])
|
||||||
|
|
||||||
|
d[sentence].append(curExtraction)
|
||||||
|
self.oie = d
|